UTM
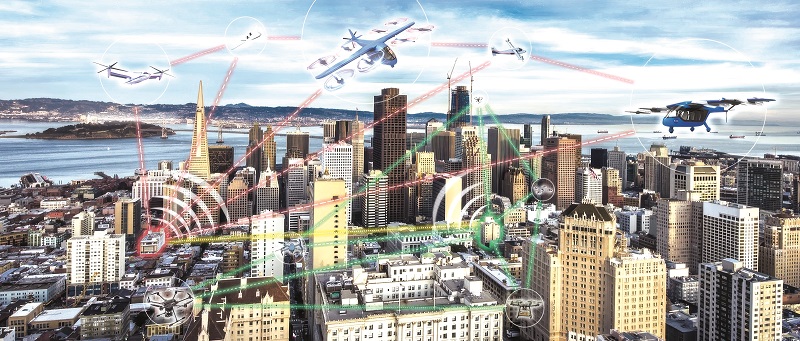
Today’s UAV designs require sense and avoid systems, and broader links with UTM systems for beyond line-of-sight operations, Nick Flaherty reports
July’s shooting of a supermarket’s delivery uncrewed aerial vehicle (UAV) in Texas, USA, highlights the challenges of operating them beyond visual line of sight (BVLOS).
They have to coordinate with other delivery craft, blue light UAVs used for rescue or surveillance missions, and even crewed helicopters and light aircraft that stray into the corridors used for deliveries.
Several companies are running small-scale delivery services with BVLOS, often with a remote operator handling 20 UAVs, mostly under a regulatory waiver under Part 107 of the US regulations, with operations up to an altitude of 400 ft.
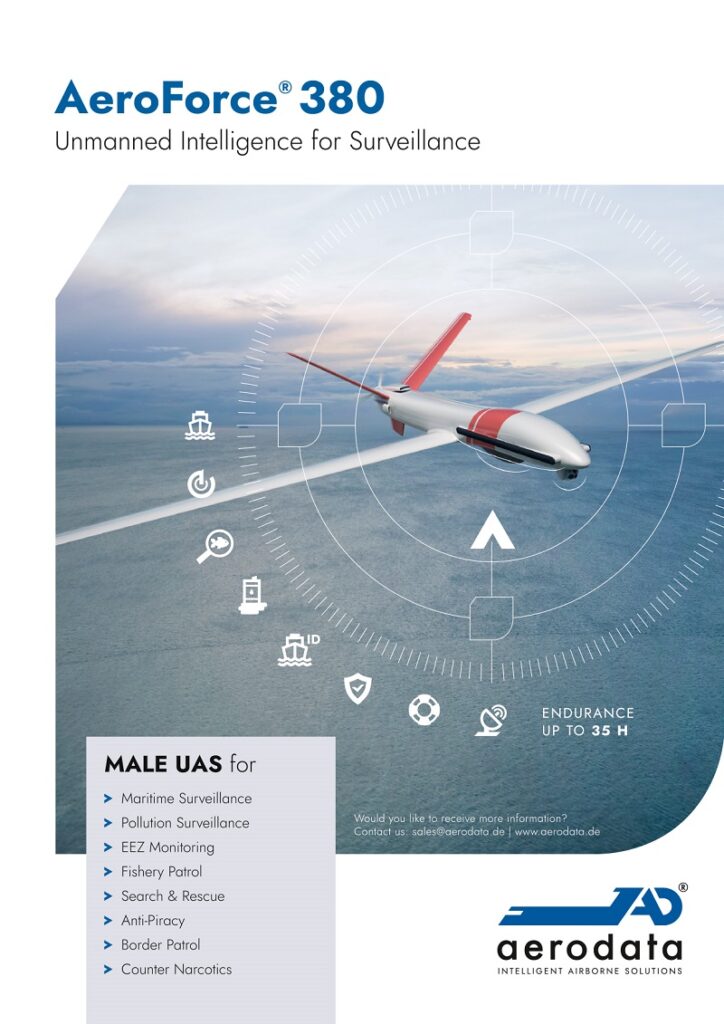
The challenge is to scale up to tens of thousands of craft with standard operating procedures, or Part 108, which is expected before winter of this year and will allow rule-based mission plans, some completely autonomous. There are many technologies, from radar to cloud, that are expected to enable the rollout of large-scale Uncrewed Aircraft System Traffic Management (UTM) systems from 2025.
This means the design of an UAV is not just about the airframe and the motors, but also the sense and avoid systems, and wider coordination for BVLOS operations with UTM systems. The next-generation delivery UAVs include specific technologies approved by regulators for UTM.
Several UAV developers include cloud services to manage their UAVs, but there is also a need to link them to the wider UTM systems of third parties. These have a range of technologies, from static and portable radar to monitor air corridors to GNSS satellite-positioning sensors on the aircraft, and even imaging or acoustic onboard sensors that can ‘see’ or ‘hear’ approaching aircraft.
This is creating a complex system with many engineering tradeoffs, and not just for the delivery of UAVs. The coming rollout of autonomous electric vertical take-off and landing (eVTOL) ‘air taxi’ platforms will require the UTM systems to integrate with traditional air traffic control (ATC) systems, and run vertiports where the eVTOLs can land and charge.
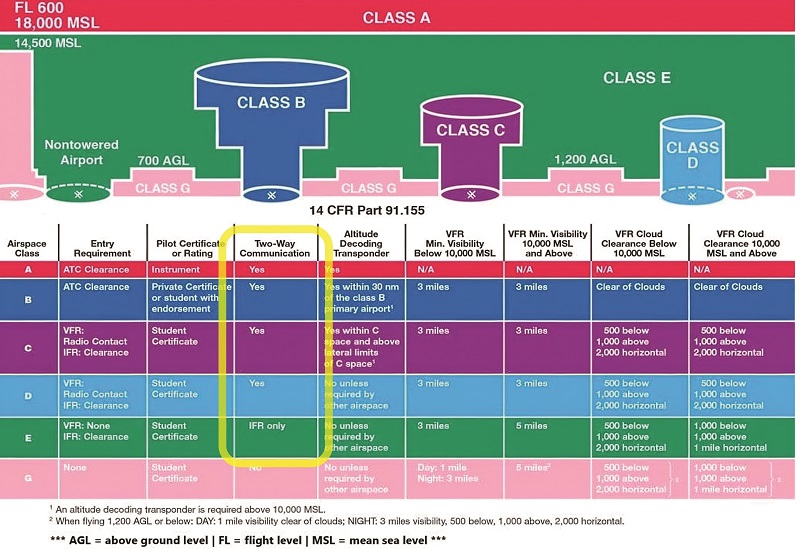
Data gathering
Beyond, a programme by the US Federal Aviation Administration (FAA), has been running for the last four years and is due to finish in October. It is focused on working towards operating under established rules rather than waivers, gathering data to develop performance-based standards, collecting and addressing community feedback and streamlining the approval processes for UAVs with UTM systems. This will face even more challenges in future as artificial intelligence (AI) and machine learning (ML) are increasingly integrated into UTM systems.
The US aeronautics agency, NASA, has been at the forefront of developments of UTM projects that take data from multiple sources to build a real-time airspace picture, coupled with collision avoidance technologies on the UAV and on the ground. This is key to detecting non-cooperative aircraft that might enter the airspace.
One of the key sensors is a network of on-the-ground radar systems that provides data on the sky several times a second. These can be fixed or portable, backpack-type sensors, and new ones can be added into the network.
This information is combined with data from sensors on the UAV, which can include ADS-B transceivers and airborne radars on larger UAVs, plus any communications data with the GPS position and input from the inertial measurement system via the autopilot. All of this is used to create an airspace visualisation that runs on a laptop, a secure server or a server in the cloud.
One key innovation is to limit the number of real-time sensors required, such as using just two radar sensors, an ADS-B transceiver or a remote ID broadcast in the latest ACAS-X system from a crewed aircraft (mentioned below).
Data from the airspace can be used to create autopilot waypoints, if allowed, to reroute a UAV around an exclusion area that appears for a blue light mission, for example.
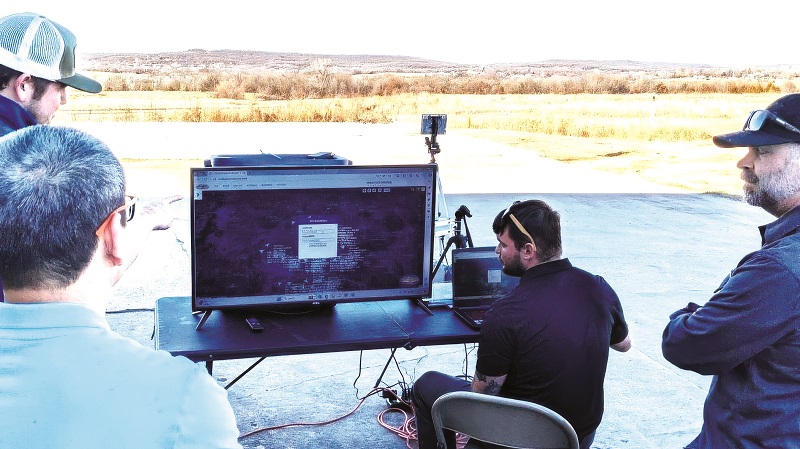
Collision countering
Avoiding a collision is usually achieved by changing the altitude of the UAV, either up or down. The UTM can monitor an intruder, and predict its trajectory and intersection with a UAV. An instruction to descend can be sent to the operator, or it can generate a waypoint that can be sent to the autopilot to override the existing flight plan and then release the autopilot back to the original one.
As the intruder may change direction or altitude, the system needs to be able to recalculate several times a second. This is achieved by having one server handle the sensors, usually radars, and another for user interface and calculation. The required processing power can be scaled in the cloud to handle the number of sensors and vehicles in the air.
Integrating radar systems from different suppliers into the UTM is another key challenge. UTM developers work with the radar makers, writing a parsing component for each radar model. Some of these can be used across multiple models such as the Asterix 2D data format, but a more generic approach is to use JavaScript Object Notation (JSON) to pull the specific data required by the UTM out of the radar sensor.
The radar-based approach has been tested with over 1000 aircraft, but the UTM typically only has to handle 20-30 UAVs at a time in a specific geographical area. Up to 10,000 flight plans can be simulated in the system to demonstrate that the UTM can cope with larger numbers.
For a large city, the system can scale in the cloud and take data from other sensors using Flight Information Management System (FIMS) and Discovery and Synchronization Service (DSS) data-exchange formats.
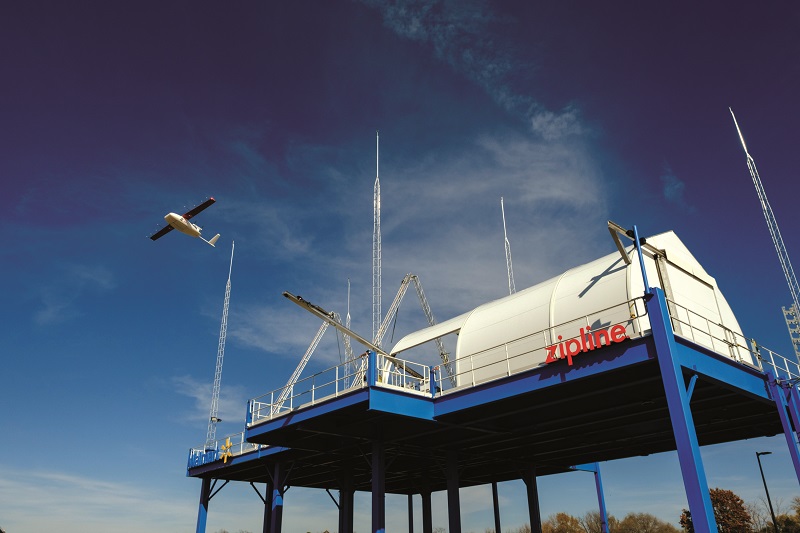
Gateways and bridges
FIMS is a gateway for data exchange between UTM participants and air traffic management systems (ATM), through which the ATM can provide directives and make relevant National Airspace System (NAS) information available to UAV operators via the UTM network.
The ATM also uses this gateway as an access point for information about operations as required and it is informed about any situations that could have an impact on the wider airspace. FIMS provides a mechanism for common situational awareness among all UTM participants and it is a central component of the overall UTM ecosystem.
FIMS is a central, cloud-based component that acts both as a bridge to the NAS and as a broker of information between stakeholders and participants. Connections to FIMS are made via a UTM service provider (UTMSP) that meets minimum certification requirements.
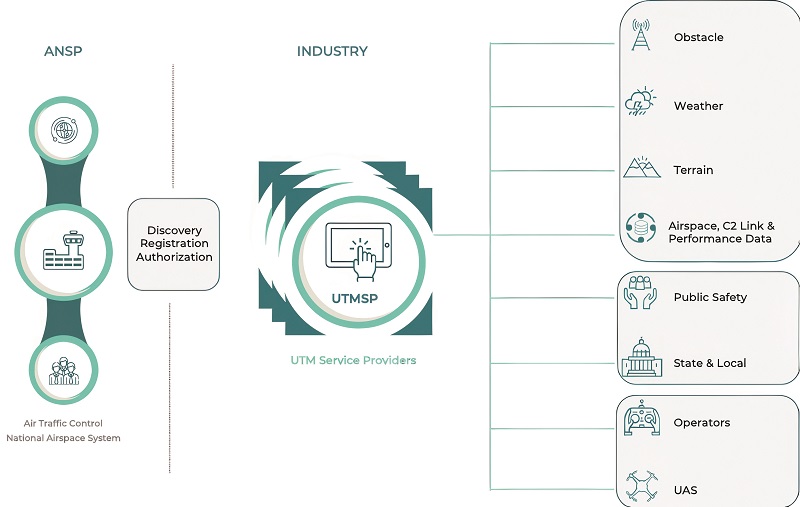
These UTMSPs support the entire lifecycle of a UAV mission flown by UAS operators. Data connections and communications between the UTMSP and FIMS are internet-based, and they are built on industry standards and protocols to ensure interoperability between multiple instances of the operator systems.
FIMS also acts as a central entity to which ATM and public services – major stakeholders – can directly input requests that affect the airspace, such as restrictions, but also extract information from the UTM system.
For example, emergency services may need to restrict UAV access to a geographic area due to a crewed helicopter conducting an emergency flight landing to pick up an accident victim. Public services will be able to extract historical data regarding the UAVs, such as flight-path telemetry, in the case of investigating a mishap.
The deployment of these functions can vary significantly and each of the features can be centralised or federated, with UTM systems linking together. For example, avoiding conflicts between aircraft in a federated architecture is more complex because the deconfliction algorithms must be universally standardised and adopted by the operators. This is similar to a problem that industry has already encountered for TCAS/ACAS avionics in commercial aviation.
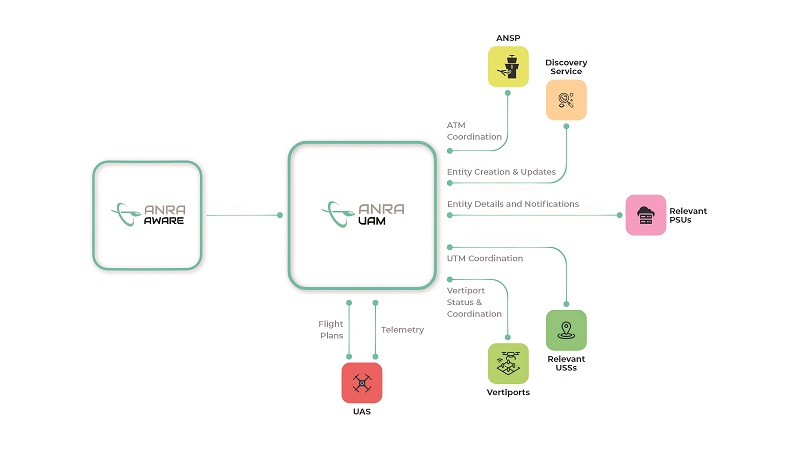
The most effective UTM systems use a blended architecture with elements of centralised and federated capabilities. This requires FIMS to play a central role in arbitrating air traffic management for crewed and, in some cases, uncrewed traffic in a mixed-use airspace when necessary, and it acts as the authorised single point of reference for critical, crewed air traffic information.
A blended model provides several advantages in terms of clearly assigning and controlling the safety-critical airspace-management functions in an unambiguous and efficient manner, thus reducing the need for complex information exchange requirements across UAV operators.
Projects to develop DSS for UTM systems are taking place in the UK, Switzerland, the US and across Europe. The DSS acts like a phone book to add UAV operators. An operator with a flight plan with a route can automatically check the DSS to get the all-clear to fly. If there is a conflict, the UAV is told to return to the operator, or dynamic rerouting is used to offer another route.
The gates are designed to ensure service providers can pass all protocols, ensuring conformance monitoring for flying the intended route, as well as setting up live flights, with the telemetry from the drones going into the network. This also accepts the upload of the mission plan and ‘deconflicts’ any clashes in real time when the UAVs are in flight, while providing notifications to nearby users.
This is being tested in simulations to ensure compliance, but it also needs automated bench-testing for the UTM system as every firmware change cannot take additional test time. So, a UTM developer works with the regulator to approve an automated test bench that can automatically test updates to the system.
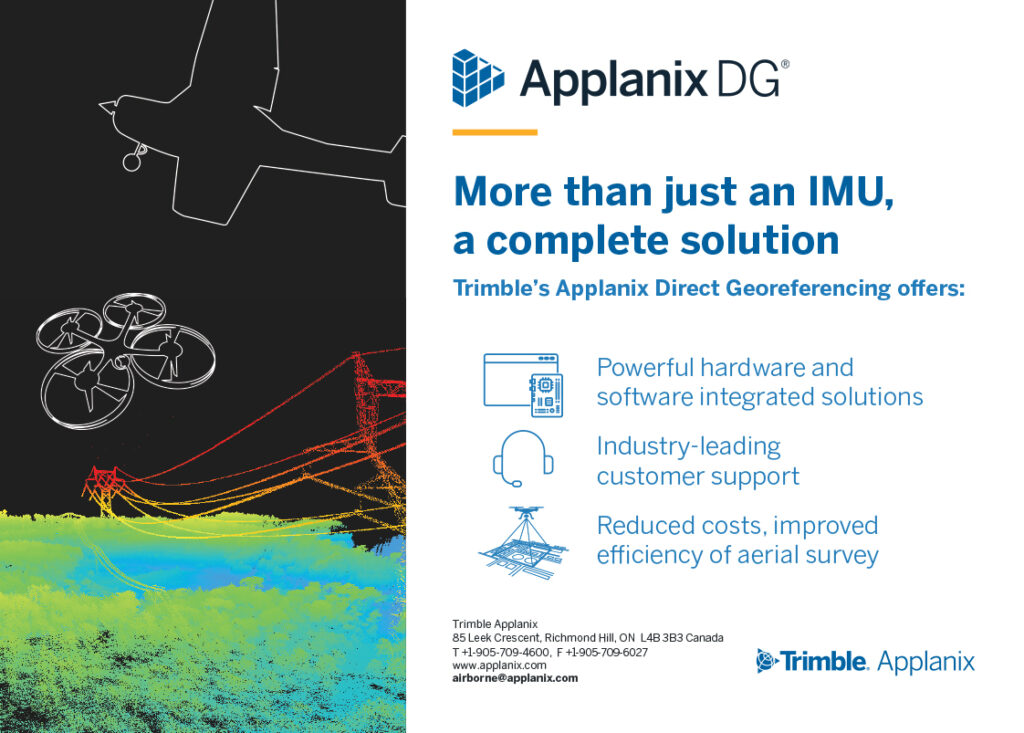
The governance that was created to ensure participants conform to the regulations allows the monitoring of uptime, aggregate conformance. This is the first time this has been done, and it sets a template for other areas and for the next UAV rules being developed.
Airspace management is expected to be included for BVLOS to make that management more routine, including architectures for communicating with each other through de-confliction.
There is also an issue around data privacy and awareness. With multiple operators, the UTM has to allow an operator to see its UAVs, but to only see other UAVs when there is a conflict as this is potential business intelligence about a competitor’s flight.
This might use geoawareness areas – a polygon with altitude, dimensions – for a no-fly zone. This is already accounted for in the standard, but the UTM has to implement it in a way that is not abused.
The central server runs an instance of Airborne Collision Avoidance System X (ACAS X) for each UAV, which provides appropriate collision avoidance for that particular aircraft. This is an onboard flight safety system that is designed to replace the current Traffic Alert and Collision Avoidance System II (TCAS) used in crewed aircraft.
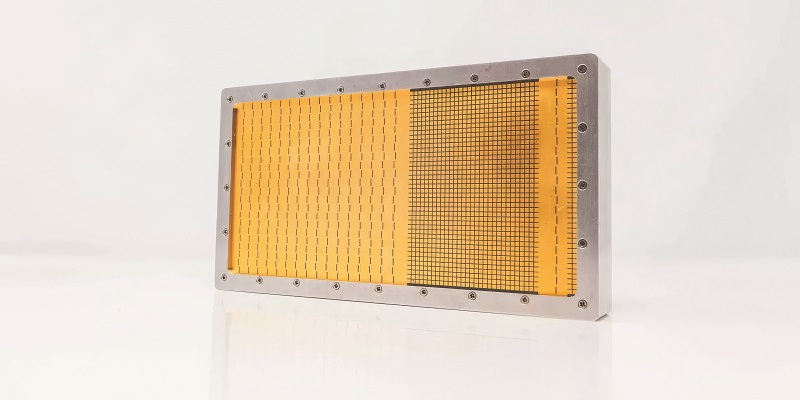
Surveillance systems
ACAS X is fully compatible with the current airspace procedures and technologies of the FAA’s Next-Generation Air Transportation System, which aims to reduce gridlock in the sky and at airports with new methods of surveillance and navigation.
ACAS X detects nearby aircraft by receiving sensor measurements from onboard surveillance systems, and it estimates their relative position and speed by using tracking algorithms. The system then weighs the costs of all the actions that the pilot could take and decides on the single best action. If a collision avoidance alert is necessary, ACAS X will send this information directly to the pilot via the flight-deck display.
A version of this system, called ACAS Xu, is tailored for use on larger, uncrewed aircraft that are equipped with collision avoidance protection, and this will provide unrestricted access to the National Airspace System for the very first time.
One of the largest UAV operations has an onboard perception system with ADS-B transponders that identify aircraft in the nearby airspace, as well as an acoustic avoidance system that uses small, lightweight microphones to detect and avoid other aircraft flying up to two miles away in all directions, including during the night and in challenging weather.
This uses an m:N operation (explained in our earlier In Conversation feature, p.20), where a small number of humans (m) manage many autonomous vehicles (N) to transport medical supplies and consumer goods in Arkansas, USA, Japan, and Ghana and Rwanda in Africa.
A working group is considering a variety of use cases for n:M operations, and addresses barriers such as technical, regulatory, safety assurance and community acceptance.
A central goal of this working group is to bring together a broad collective of interested stakeholders from government, industry and academia to identify and reduce barriers to m:N operations; an operational configuration that envisions a ratio of multiple operators (m) controlling multiple vehicles (N) between them.
The barriers addressed by the working group range from technical, regulatory and safety assurance to community acceptance. Identified barriers are considered across a variety of multi-vehicle control contexts (e.g. urban/advanced air mobility, delivery, infrastructure inspection, disaster response and recovery, and high-altitude pseudo-satellite operations), and they form the basis for future research to confront operational, technical and regulatory gaps.
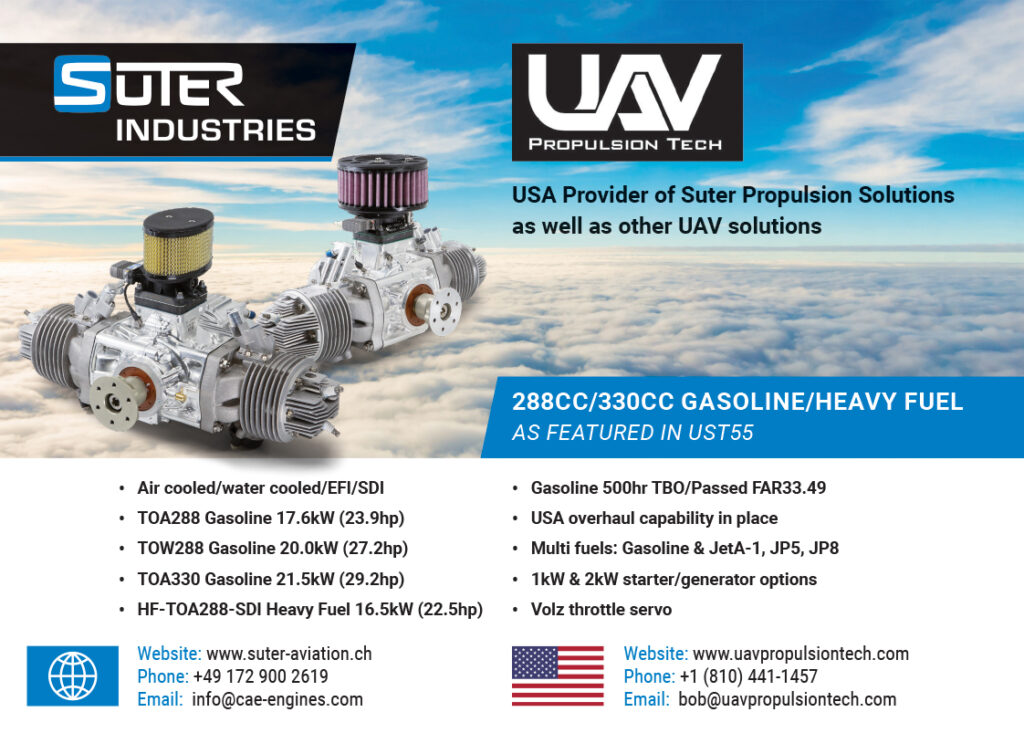
Sense and avoid
Radar on the UAV can also be used for sense and avoid. Recent tests in Phoenix, Arizona, USA, showed the radar can not only detect airborne traffic but can also decide autonomously on a course of action. The radar can take over navigation and pilot an aircraft to safety using its onboard processor.
Avoiding unforeseen objects is a key requirement for autonomous drones and other aircraft that fly beyond visual line of sight (BVLOS) of an operator. However, this detect-and-avoid capability is extremely difficult in the air. Radars must have long ranges because of the high speeds involved, and they must pick out airborne traffic from ground clutter, including moving cars. They also require precise location information to make sense of radar echoes.
This is difficult on the ground and even more complex in the sky. To compensate, pilots, and even huge air traffic control radars, rely on cooperative aircraft to beam out their locations using onboard transponders.
The onboard radar is the size of a paperback book and weighs less than 1 kg, and it has already been used on crewed aircraft and helicopters to detect non-cooperating traffic. The onboard processor calculates avoidance paths, meaning aircraft do not need a separate computer to do this work.
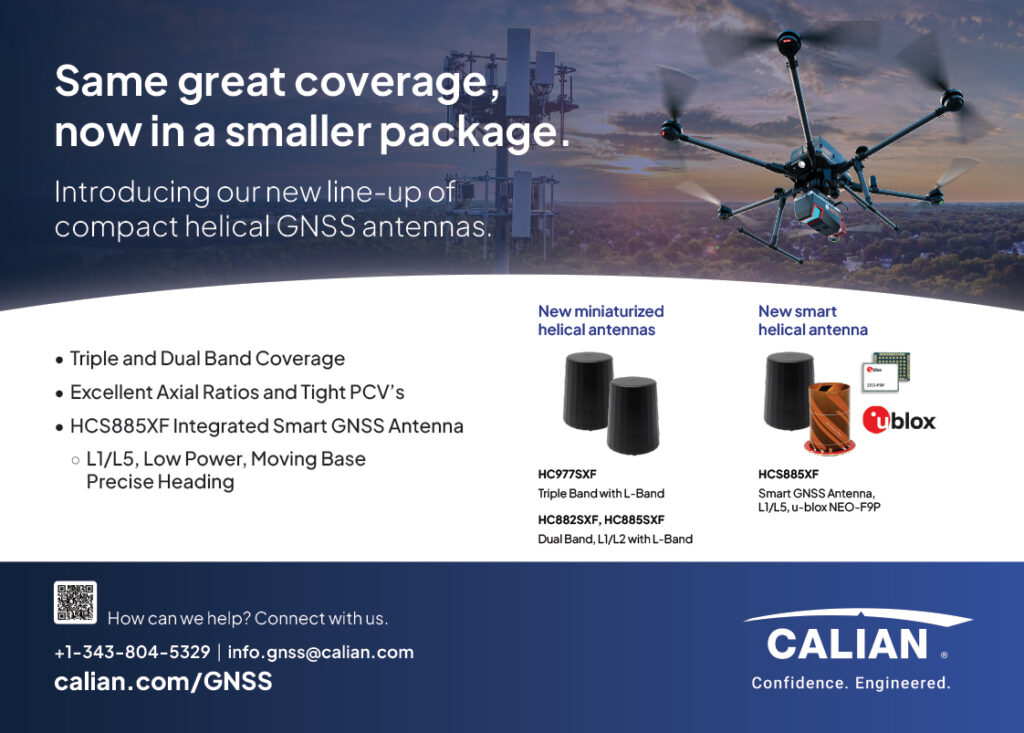
Two quadcopter UAVs flew at each other, 300 ft above the ground, at a test site in the desert. The UAV detected the ‘intruder’ and evaluated its flight path. Then it calculated an avoidance manoeuvre and took over the navigation to fly left, right, up, down or stop in midair, depending on winds and other factors.
Once the danger of a collision had passed, the radar released control of the drone and the autopilot guided it back to its original course, all automatically. The team then challenged the radar with increasingly difficult encounters, such as approaching from below to blend into ground clutter and from offset angles, testing the radar’s peripheral vision and high, angular detection capabilities.
In other flights, the team instructed the radar to wait longer before acting, forcing it to make more aggressive manoeuvres.
The radar has a range of 3 km using a monopulse technology with a system of overlapping beams to increase accuracy and eliminate ground clutter. The radar steers its beams electronically, so it has no moving parts.
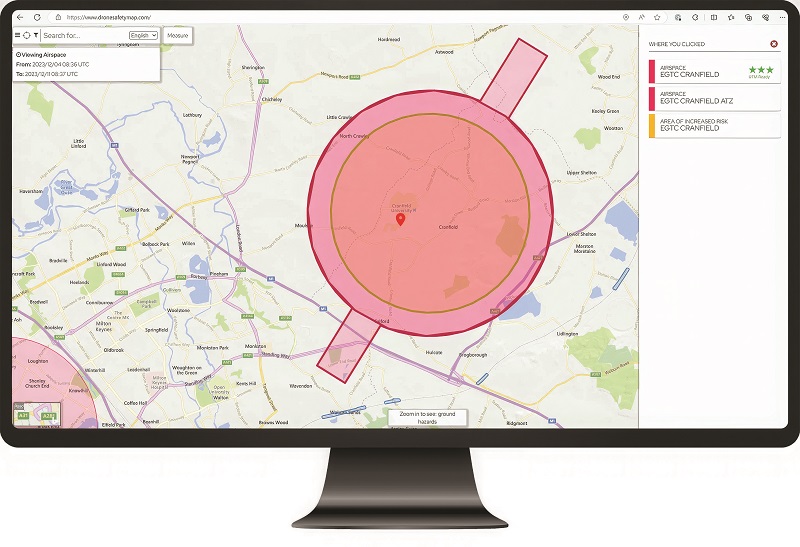
Approval services
An alternative UTM approach, especially around controlled airspace such as an airport, is an Approval Service platform. This allows UAV operators – and, in future, eVTOL pilots – to request and pay for operations within an airport’s airspace digitally at the touch of a button.
The Approval Services platform links to flight-planning software without having to contact an airport, airfield or aerodrome to seek approval, and allows delivery services to operate in areas that otherwise would be difficult.
By seeking a green light through Approval Services, UAV operators can get flights approved within minutes whereas previously it may have taken weeks. However, making airspace managers pay to configure and impose an administrative fee for reviewing and approving flight requests, where appropriate, is not necessarily popular as it is viewed as contradicting guidance that says UAV operators cannot be charged for access to airspace.
In the UK, the CAP 722C guidance provides policy and advice to sponsors of airspace and UAS operators, while the regulations for airspace sponsors and UAS operators can be found in UAS Regulation (EU) 2019/947.
Additional platform services are becoming necessary because the amount of information being handled by airspace managers is increasing rapidly. An Approval Services platform enables an airspace manager to understand and manage airspace in a safer, more controlled way, with UAV operation marked clearly on a map and the operator’s details to hand.
Japan has developed a UAV traffic management system for multiple operators in the same airspace. The project, named Drones and Robots for Ecologically Sustainable Societies (DRESS) brings together 29 partners, including private companies and research institutions.
In the DRESS project, the UTM system includes a simulator, whereby the traffic management of numerous UAV flights can be simulated. The UTM helps multiple UAVs to fly more freely beyond visual line of sight within the same airspace.
Testing at the Fukushima Robot Test Field in Minamisoma, a city in Fukushima Prefecture, connected 29 UAS operators via the UTM system and flew 46 drones in a shared airspace of 1 km2, completing a total of 146 flights within one hour.
During the test, the UAS operators used the UTM system to obtain information about the geography, climate and airspace, with flight-restricted areas. The operators also shared information on their flight plan (planned flight route or area) and real-time flight status (actual location, altitude and speed of UAS) via the UTM system.
Connecting the UTM simulator to the UTM system helped to verify the methods and procedures for avoiding mid-air collisions when a drone suddenly changes its flight route and approaches the proximity of other drones.
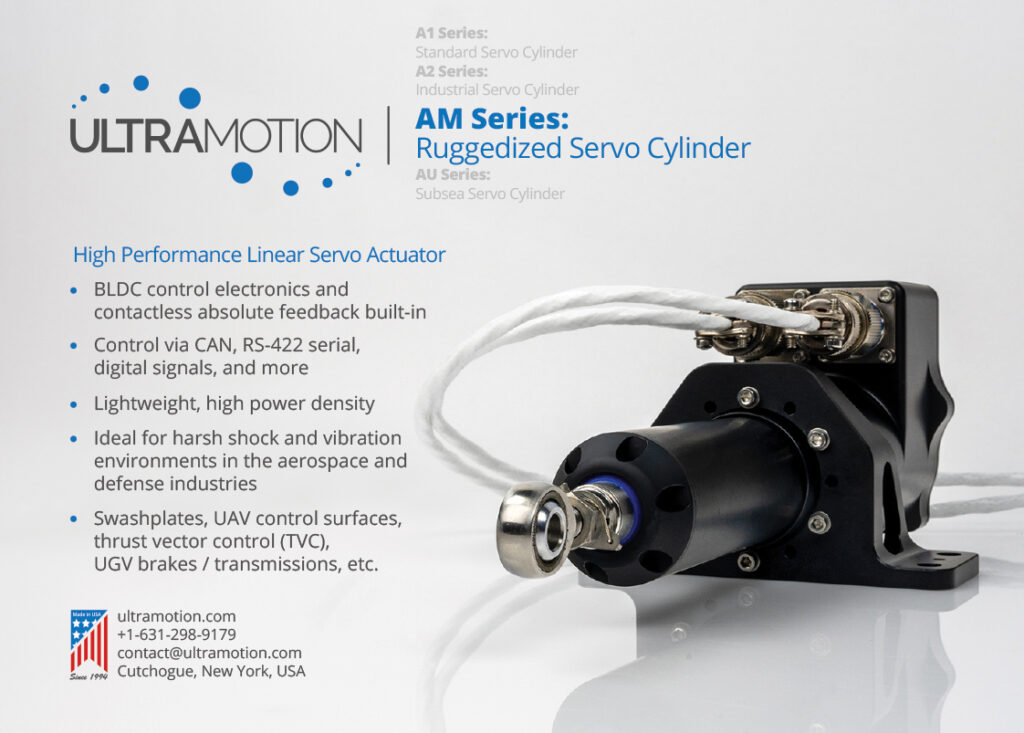
AI algos
The growth in Urban Air Mobility (UAM) and UAV operation is expected to see a significant growth in air traffic, and AI algorithms are viewed as a way to provide more automation. However, these can be difficult to certify, and they provide a deterministic system that can operate reliably and be approved by regulators.
Various forms of AI are being developed to support the UTM. One technique is based on aerial photos and geodata to automatically identify no-fly zones. The AI framework identifies appropriate landmarks in aerial photos that account for no-fly zones, such as over airports, federal waterways or wind turbines, in an automated process. Machine vision and learning methods are able to reliably detect structures and patterns, and classify them correctly.
Information from aerial photos is supplemented by geoinformation from various sources and its heterogeneity is a major challenge. The goal is to visually process all data for the user in an easily understandable way in order to provide a transparent view, and this data helps with immediate decision-making.
To accomplish this, a platform is being developed with AI-based computer-vision algorithms to automatically collect, process, virtually integrate and, ultimately, visually provide flight-relevant geoinformation.
Another approach to path planning problems with separation constraints for ATM also uses AI. MuZero is a deep reinforcement-learning algorithm that can be used for path-planning problems in dynamic air traffic environments. It uses a sequential, trajectory allocation approach that would act on a first come, first served basis for both online planning and moving time horizon problems.
Initial results show that agents can learn to mitigate collisions when trained with the obstacle avoidance framework, based on the MuZero algorithm, without requiring any knowledge of the domain rules.
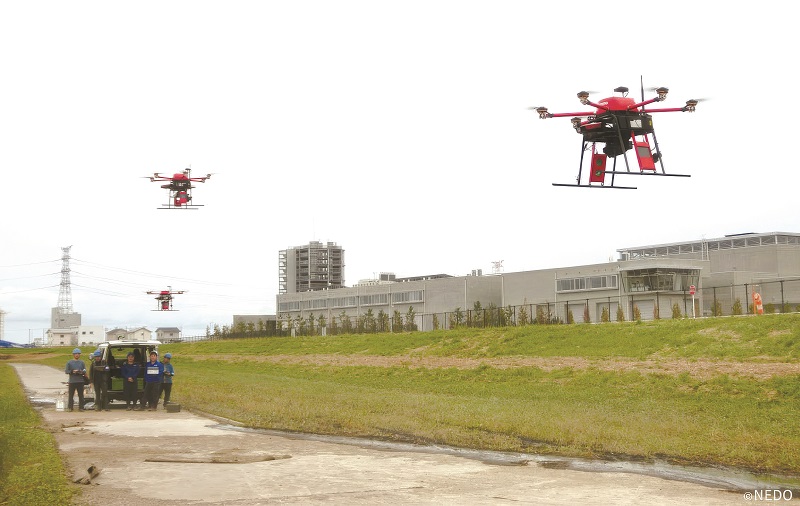
Acknowledgements
With thanks to Kraetti Epperson of Vigilant Aerospace Systems, Brent Klavon at ANRA and Harada Kenya of Jaxa.
Some examples of third-party UTM suppliers
FRANCE
Thales Aerospace | – | www.thales.com |
ISRAEL
Airwayz | – | www.airwayz.com |
POLAND
Drone Radar | +48 511 230 660 | www.droneradar.eu |
UK
Altitude Angel | +44 118 391 3503 | www.altitudeangel.com |
USA
ANRA Technologies | +1 703 239 3209 | www.anratechnologies.com |
Vigilant Aerospace | +1 405 445 7224 | www.vigilantaerospace.com |
UPCOMING EVENTS
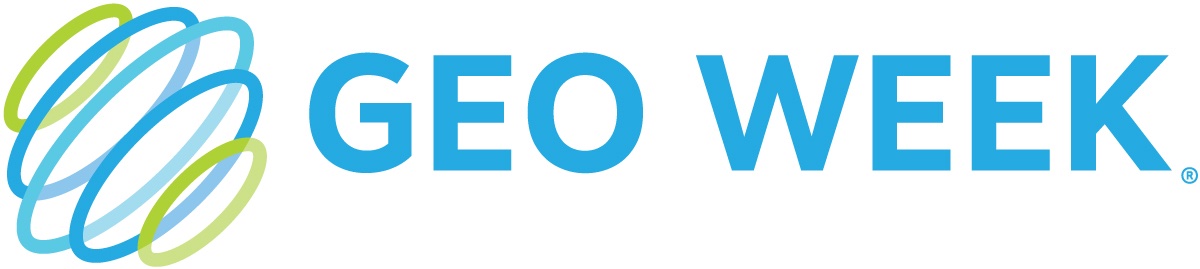
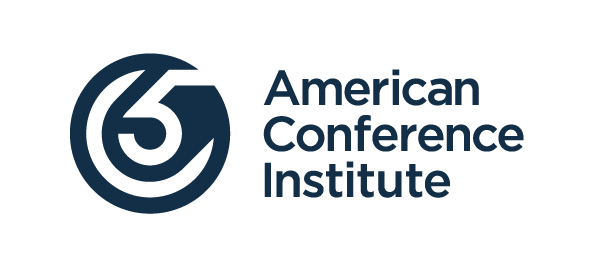
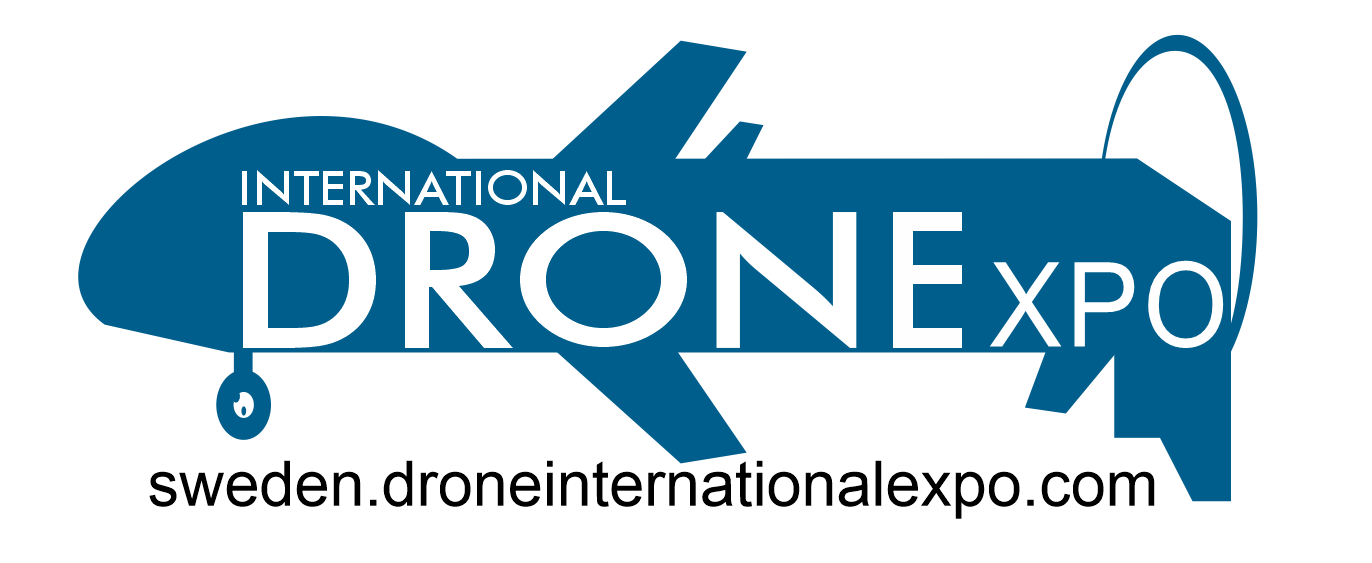
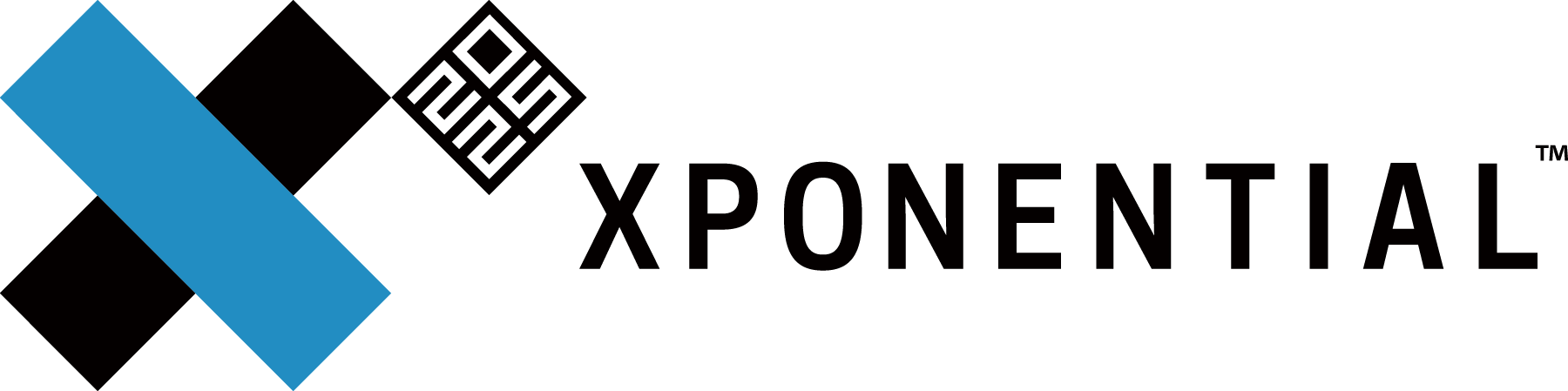
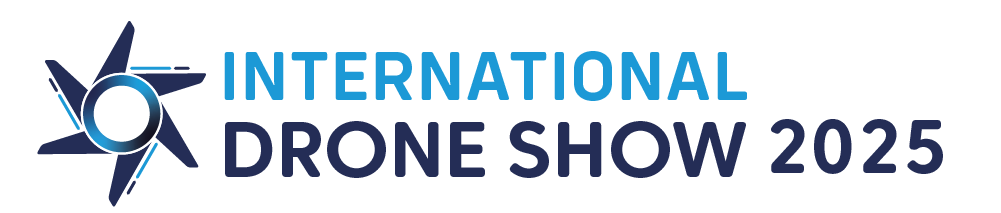