BeeX A.IKANBILIS
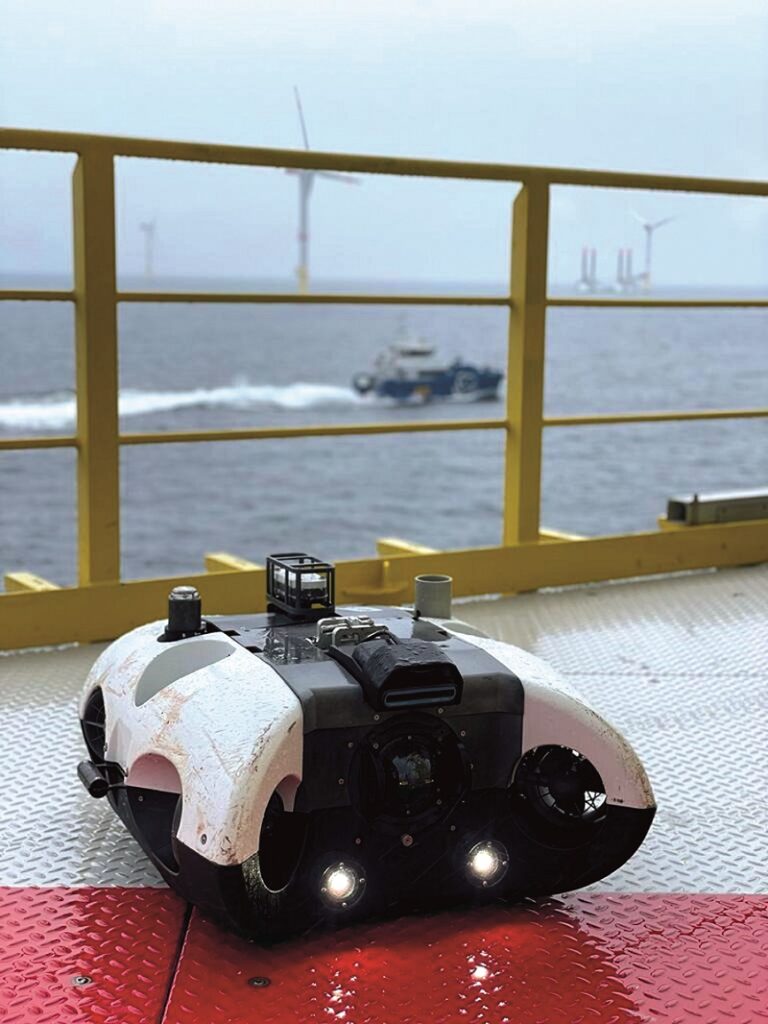
(Images courtesy of BeeX)
Standstill working
This hovering AUV’s capabilities are proving an ideal fit for inspecting the foundations of offshore wind farms. Rory Jackson reports
The global expansion of wind power has accelerated the uptake of uncrewed systems as a safe and cost-effective means of inspecting and maintaining wind turbines. And although UAVs and USVs might become ubiquitous in offshore wind farms, and even work together (see our dossier on TideWise in issue 45, August/September 2022), underwater vehicles for the most part have yet to report any great advances in the industry over the past few years.
Some may find that surprising, given that any non-floating wind farm relies on stable, well-maintained foundations – referred to in the industry as ‘piles’ – to keep them upright and working, so naturally they require inspection in order to identify and correct any damage over time.
“To be sure, wind farm operators are calling out for underwater autonomy,” says Jan Schmoekel, balance-of-plant engineer at Northland Power, which owns and operates wind farms. “In particular, offshore wind farms – like the Nordsee One field in the German North Sea that I’m responsible for – are most often built on piles. Nordsee One specifically uses monopiles, tripod and tri-pile foundations.”
These are simple structures typically made from stone, gravel and steel. They are economical to build and maintain, but as Schmoekel explains, regular inspection is vital for monitoring critical parts such as cable protection systems, scour protection systems (which prevent erosion of the pile from currents and waves) and current cathodic protection anodes (which guard against galvanic corrosion of the monopile).
However, ROVs require an extensive and costly spread of ancillary equipment in order to work, particularly with regard to launching, recovering, tethering, operating and maintaining them. They therefore need large, expensive vessels on site – whether owned or hired – to perform these tasks.
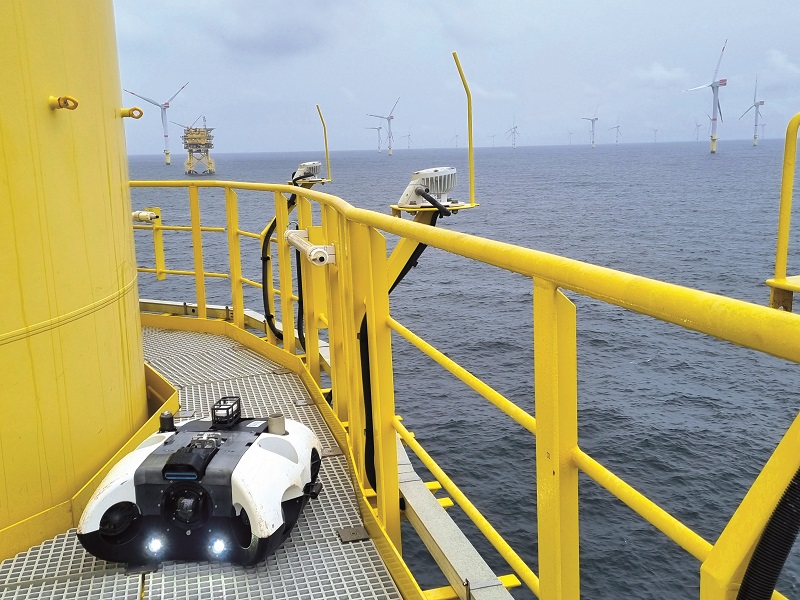
AUVs require no tether for power or control, nor do they always need a crane for launch and recovery, allowing a wider (and potentially less costly) range of smaller ships to be used for AUV inspection missions; their autonomy also makes their operations less labour-intensive. However, with those benefits comes the loss of real-time human intelligence, whereas ROVs stream live visual and sonar feedback to their operators, who can then direct the vehicle closer to or further along anything of note.
Most AUVs will only provide feedback once they have surfaced (delivering their survey data by wireless link) or been recovered (by cable or removable memory drive), so to gain further insight into any signs of wear or damage, the wind farm owner would need to perform or commission a second mission, potentially followed by a third, and so on.
Wind farms are also prone to tidal effects, particularly those relatively close to the shoreline such as Nordsee One. These play havoc with ROVs, primarily owing to their tethers being dragged around, and with AUVs as they lack the self-stabilising motor configurations of ROVs.
Maintenance expenses therefore remain an Achilles heel, not only for Northland Power but any company hoping to expand their wind farms.
However, a potential solution presented itself in the form of Singapore-based BeeX Autonomous Systems and its A.IKANBILIS HAUV (Hovering Autonomous Underwater Vehicle), which in September 2022 demonstrated its ‘adaptive autonomy’ technology at Nordsee One.
Adaptive autonomy refers collectively to the capabilities that BeeX (led by CEO and co-founder Grace Chia) has tailored to enable the A.IKANBILIS to inspect every nook and cranny of the Nordsee One piles with the persistence and wear recognition of a human operating an ROV, but while functioning with the superior autonomy and efficiency of an AUV.
“The main difference between us and other AUV companies is that BeeX’s genesis came from a team with 10 years of self-driving car development at the National University of Singapore, and although we’ve pivoted into the marine realm, we’ve carried over our core competencies in AI software,” Chia says.
“Self-driving autonomy stacks can actually be hugely applicable to underwater autonomy, but that applicability hasn’t received much attention or investment compared with autonomous cars or drones.”
BeeX found its automotive competencies to be a huge enabler in its comparatively recent ambitions to create a UUV that can think for itself – so much so that Northland Power specifically chose the company to demonstrate that its autonomous vehicle could perform a ‘launch and leave’ inspection of a wind farm under real-world conditions.
HAUV capabilities
Already available to rent in Europe through BeeX’s Hamburg-based distribution partner Subsea Europe Services, the A.IKANBILIS measures 90 x 80 x 40 cm and weighs about 60 kg in air. It is fully electric and is fitted with two 500 Wh subsea-rated lithium-ion battery packs, enabling up to 8 hours of underwater operation between charges.
The hovering element comes from an arrangement of seven shrouded thrusters disposed about the HUAV’s hull. Three are oriented vertically (and positioned at the left, right and rear) for ascent, descent, pitch and roll, while four are oriented horizontally and are pointed tangentially from the hull to enable forward, reverse, sideways and rotational movements.
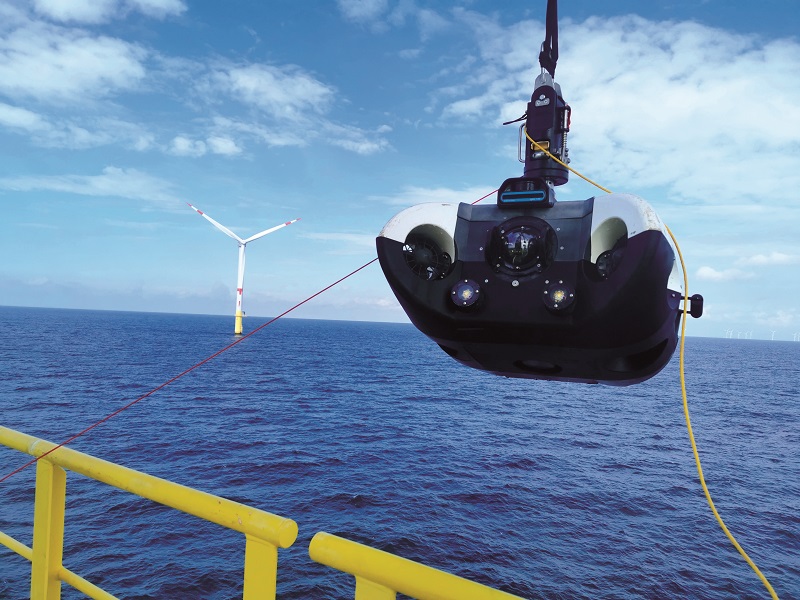
That enables it to hold its vertical and horizontal position much like an ROV, or like a couple of other AUVs with similar propulsion configurations (see Hydromea Vertex dossier, UST 16, October/November 2017, and Marin mAUV digest, UST 32, June/July 2020, for example). Having so many motors will consume a lot of energy in strong currents, but the end result is that the vehicle stays stable and continues to gather high-resolution data, and consumes less energy than a tethered ROV.
The autonomy is powered by an Intel CPU and an Nvidia GPU. These carry the algorithms for processing data from a frontal HD camera and Blueprint Subsea Oculus 3D imaging sonar (although different sensors can be integrated depending on the mission, environment and customer). That makes the A.IKANBILIS one of very few AUVs in the world to run a CPU and GPU in parallel in real time.
“We are partners in Nvidia’s Inception programme aimed at AI start-ups,” Chia adds. “We leverage the support from that programme to ensure we’re deploying their latest technology in our vehicles.”
As with many self-driving cars, the AI inherent in BeeX’s adaptive autonomy has been developed using deep neural networks through the use of copious image data, but as Chia notes, underwater image databases are far harder to come by than those of, say, roads and traffic.
“This capability of ours has therefore come through us thinking carefully about how to leverage the best of self-driving car algorithms, and then building our application-specific hardware and software around that middle layer,” she says. “Over the years, at university and after, we iterated different hardware products to find the package of elements that best gel together for the kind of AUV that works well for our customers.”
In the North Sea
Nordsee One and Northland Power’s other wind farms are serviced via a crew transfer vessel (CTV) and service operations vessel respectively. The CTV was therefore used to carry the Northland Power and BeeX technicians, and the A.IKANBILIS HAUV, to Nordsee One, specifically to the turbine where the HAUV was to be deployed to prove its capabilities. (Schmoekel explains that the turbines “all look the same”, so a single turbine was sufficient as a test case.)
“That’s an important point – we wanted to first show that existing CTVs and infrastructure in general were sufficient to transport everything necessary for our HAUV, so that buying our vehicle or renting our service could easily fit within existing operating procedures and equipment,” Chia says. “No-one should have to order in a whole new vessel just to use autonomous vehicles, no matter what the savings in their running costs brings.”
Once there, a crane on the turbine was used to lift the HAUV up from the CTV deck and onto the transition piece, a deck that sits around the middle of the wind turbine. That is difficult with work-class ROVs, which are often made larger and heavier to help them mitigate the effects of currents.
“From there, we activated the vehicle, lowered it into the water via a tether, and it then started to use its camera and sensors to build up a map around itself for navigation and localisation, so that the mission could begin,” Chia notes.
She adds that the tether was used purely as a recovery tool of last resort, simply to make sure the HAUV would not be lost owing to unforeseen circumstances, as the company has yet to complete development of a launch and recovery system. Being much thinner than power cables, its smaller footprint meant it underwent far reduced dragging and pulling than an ROV.
As well as the HD camera and imaging sonar, three other inspection sensors can be added on a plug-and-play basis, Chia’s team having observed that complex sensor integration processes take up inordinate amounts of preparation time in HAUV missions.
While the arrival and launch phases were straightforward, it belies the extensive preparation BeeX performed in-house to achieve the necessary intelligence specific to wind farm pile inspections. This required considerable r&d by the company on how the vehicle would move underwater in response to different forces.
“We started by importing 3D models of the wind farm into our simulator, and mimicking underwater currents as well as the power and thrust dynamics of our propulsors while dealing with those currents,” Chia recounts. “Our test pilot used that simulator to experiment with how the vehicle could achieve stable hovering and movement through the distributed thrust across the seven motors.”
Adaptive autonomy
Once underwater, the HAUV autonomously recognised the shape of the structure, which triggered it to approach and begin looking for key features. This included trained recognition of the cable protection systems at the structure’s base, as well as observing the foundation rocks that obscured these systems to ensure it captured clear images of them.
Although the A.IKANBILIS’ nominal endurance is 8 hours, in the environment around Nordsee One it was able to achieve only about 3 hours. The reason for that are the currents, which as mentioned are strong enough to heave any vehicle around and thus severely disrupt the quality as well as quantity of images, whether 2D or 3D.
Throughout the survey, therefore, the HAUV’s various thrusters worked persistently to maintain its position in all 3 axes while capturing 2D and 3D imagery, with the vehicle working at an average of about four times its typical power consumption throughout.
“This practical endurance will increase in the future – we’re looking to integrate more than 1 kWh of battery capacity, and we don’t anticipate much challenge in doing so, but our first priority was to make sure the autonomy engine was stable,” Chia explains.
Schmoekel adds, “The propulsion configuration in its current form worked great. Essentially the stability and precise point-to-point imaging it performed was typical of the sort of work-class inspection an observation-type ROV one might otherwise use, but with none of the downsides. Despite the strong northerly currents we get in the German North Sea, the HAUV could still safely manoeuvre around the wind turbine’s monopile, following along potential signs of wear when it ‘thought’ it had to.”
Chia notes that a lot of work went into developing the ideal kind of thrusters for hovering: most off-the-shelf AUV ones “are designed to go forwards, fast”, she says. “Most HAUV missions are centred on simply arriving at the mission location as quickly as possible, so you can then survey at a low forward cruising speed, so there aren’t really any thrusters out there for remaining as still as possible for high-resolution inspections.”
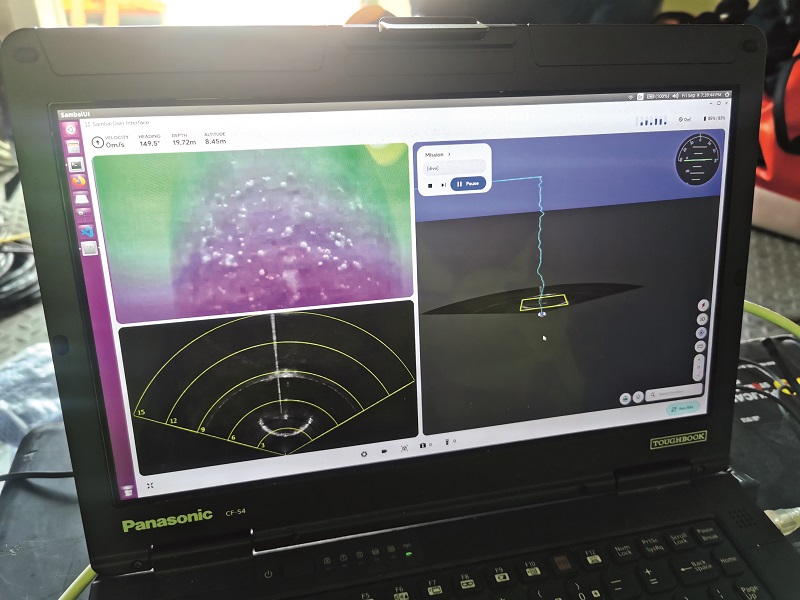
BeeX therefore developed its propulsion configuration in-house, optimising its motor-propeller combinations and integrations to achieve balanced, distributed thrust and hydrodynamics in all axes of movement (similarly to a multi-rotor). Even with strong lateral currents, the vehicle was never moved to any significant degree.
As the vehicle carries out a survey, two LED lamps on its front face (integrated just below the HD camera) provide up to 4700 lumens to illuminate the objects being inspected. The camera is optimised for ensuring effective visual data capture underwater, as well as perception and positioning.
“The lack of GNSS under water means AUVs need to rely on acoustic positioning in order to localise and navigate,” Chia comments. “We actually use quite a simple off-the-shelf HD camera but that, combined with a 3D imaging sonar used in a dual-purpose mode and with our software algorithms enables a multi-layer approach to achieve robust autonomous navigation without GNSS or the extra infrastructure and costs of a USBL or LBL system for acoustic navigation.”
BeeX’s in-house positioning system calculates its best estimate of the vehicle’s position in the x, y and z axes based on a fusion of inertial, camera and sonar data, while its autonomy algorithms take into account the likelihood that the wind farm will cause disturbances (given that metal can reflect acoustic signals and concrete will absorb them). Chia notes that any uncrewed underwater vehicle relying on raw navigation data among wind turbines without constant corrective GNSS or software updates will probably be navigating incorrectly.
“Our AI algorithm stack is split into two sections, one of which is for baseline navigation work, as in how the HAUV thinks and moves,” Chia says. “We operate very differently from vehicles that rely entirely on waypoints, because our HAUV can choose to deviate from them in order to prioritise the actual inspection goal our customers want.
“It follows that the second part is the analysis of mission-specific areas of concern – essentially the targets our end-users want the A.IKANBILIS to recognise, zoom in on and search around for detail.”
BeeX’s original purpose for installing an imaging sonar onboard was purely so that the A.IKANBILIS could sense where it was going. However, during development, Chia and her team realised that the sonar images could be stitched together with the camera and inertial information to create a new data product. That was a 3D mosaic of everything the vehicle could sense throughout its survey, and it provided a means of giving the end-user more high-quality data without adding more sensors or needing to swap them in or out for a second dive.
“Our technique of cross-referencing between camera and sonar images minimises the number of false positives that the vehicle detects underwater, primarily because we carried out a lot of real-world tests while developing the system in Singapore’s coastal waters, where we had even less visibility than at Nordsee One,” Chia recounts.
“These were mainly trial inspections of near-shore infrastructure, particularly of 50-100 cm pile structures along the wharf terminals with a similar shape to wind turbine monopiles, and some sloped land reclamation projects that would exhibit similar sensor interferences as the piles. Ports and harbours tend to have very murky waters that often make camera solutions unviable, but since our roots are in self-driving cars, we had considerable experience of using multiple payloads simultaneously.”
The tests were scaled up in May 2022 with the inspection of a 3 m-diameter monopile, which was repeated to optimise the A.IKANBILIS’ adaptive autonomy until the team was confident it could successfully inspect the 6.7 m monopile at Nordsee One.
“So, as much as the camera and illuminators are important for the end-user to gauge the condition of the wind farm – and we allow customers to install additional payloads specifically for data collection – our autonomy and inspection capabilities are designed to work with or without visibility in the water,” Chia says.
A data snapshot
The Nordsee One survey was performed repeatedly over the course of 5 days. Although most of the valuable data (for Northland Power) was captured on the first day, BeeX’s engineers were invited to develop, deploy and test new autonomous behaviours on the A.IKANBILIS using subsequent launches, as each survey’s telemetry was highly valuable for training the HAUV’s autonomy. The modular adaptive autonomy stack enabled a fast turnaround time and straightforward installations of new behavioural blocks developed ad hoc.
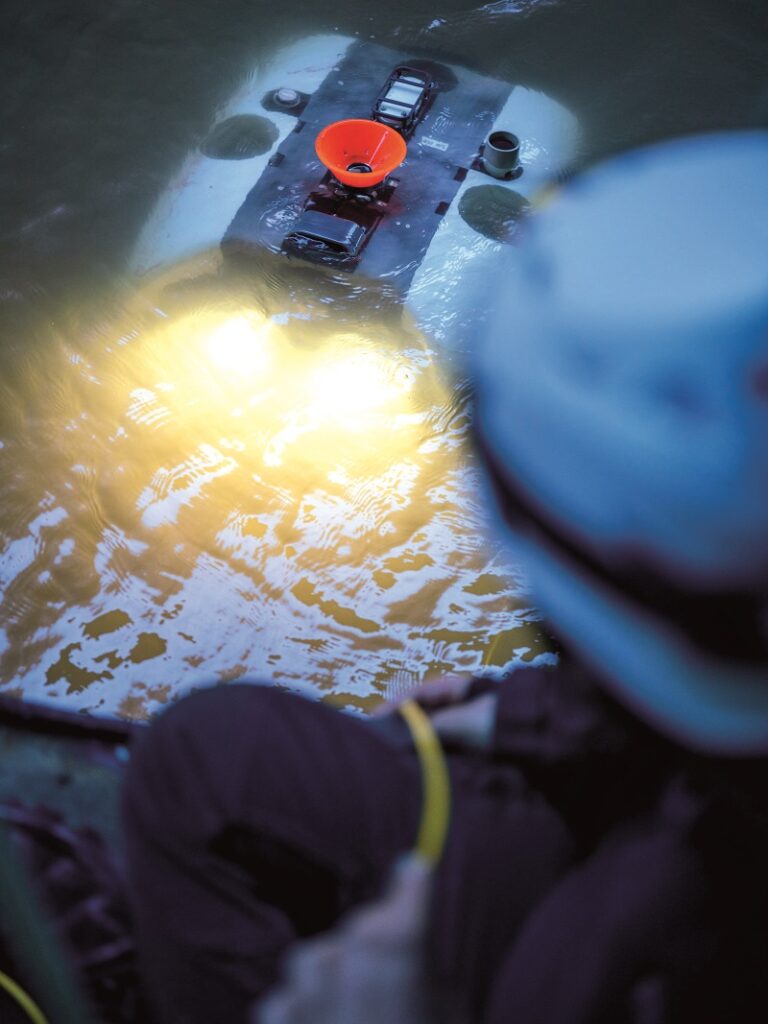
After hauling the HAUV out of the water using the tether each time, and performing a minor freshwater cleaning of the hull, sensors and thrusters, a technician presses the synchronise button on the GUI. That triggers the sending of ‘snapshots’ to the wind farm operator showing the most important areas observed during the inspection.
“An inspection of one wind farm’s foundations might take around 30 minutes but still only find a few areas of potential concern,” Chia says. “As well as building our software and the hardware around it, we also came up with a clear idea of how to handle our data and report it to the customer.”
The data processing software has therefore been optimised around quickly constructing these snapshot reports to enable a package of actionable information to be sent to the end-user, saving them the time and labour of poring through the 2D and 3D data for a large wind farm that could run into hundreds of hours’ worth.
“The snapshot is an interactive 3D representation of the wind farm, which was uploaded to a cloud-based workspace with an integral workflow functionality that served to guide Northland Power through the things they were actually after,” Chia notes. “The cloud connection is achieved by a 4G antenna, which we connect to the vehicle via a cable after recovery.”
From one to many
In addition to potential future wind farm inspections, Schmoekel envisions further uses for the A.IKANBILIS’ adaptive autonomy.
“I used to work in subsea ordnance clearances, which still rely on huge, heavy work-class ROVs,” he says. “From that background, it was important for me to see how stably the HAUV could behave around the monopile structure and within the water column, using all its sensors for simultaneous surveying and navigation – and as far as I’m concerned, that’s as much of a success as the data we got on our turbine,” he says.
“The AUV has huge potential across maritime inspections, particularly as its engineers improve its autonomy for guidance and inspection precision with each dive. We therefore want to give them the chance to trial and progress their development further, and to offer them our wind farms to do that.”
Northland Power, BeeX and Subsea Europe Services already intend to perform further trials with the A.IKANBILIS in 2023. The latter in particular hopes to begin honing the repeatability the vehicle can achieve across inspections of multiple wind turbines per dive, while Northland Power plans to work with BeeX to determine when it will be able to fully replace its ROVs with the HAUVs.
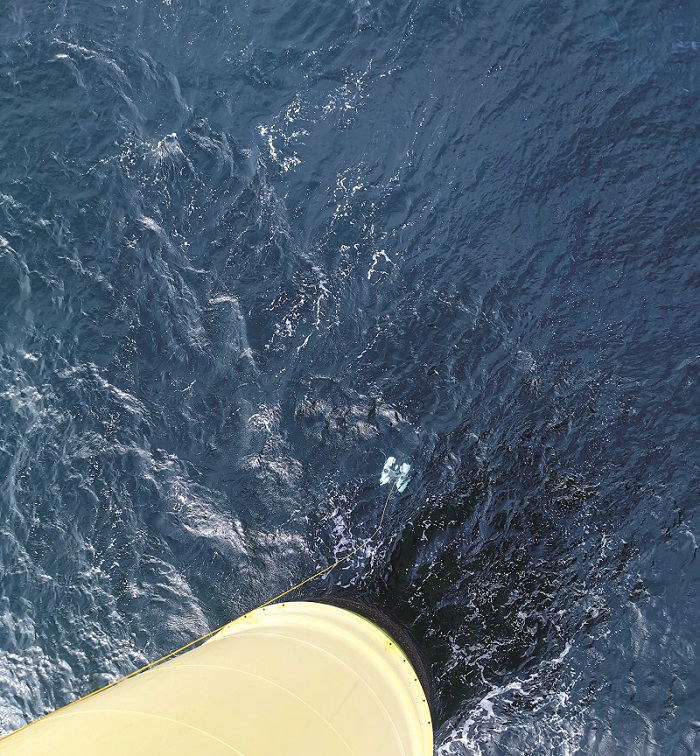
“Wind farms can’t continue expanding at their rapid pace if they aren’t offeredIn future, BeeX and Northland plan further trials with the A.IKANBILIS, including inspections of multiple wind farms per dive
a way to curb the accelerating costs associated with inspecting larger and larger farms,” Chia says. “If we can show industry regulators that the A.IKANBILIS can survey a whole wind farm autonomously, robustly and at low cost, it will kickstart a new chapter in renewable wind energy investment.”
Specifications
A.IKANBILIS HAUV
- Dimensions: 90 x 80 x 40 cm
- Weight: 60 kg
- Onboard energy: 1 kWh
- Maximum endurance: 8 hours
- Hovering endurance: 2 hours
Some key suppliers
- CPU: Intel
- GPU: Nvidia
- Sonar: Blueprint Subsea
- Acoustics comms: Sonardyne
- Gyrocompass: iXblue (now Exail)
- DVL: Teledyne RD Instruments
UPCOMING EVENTS
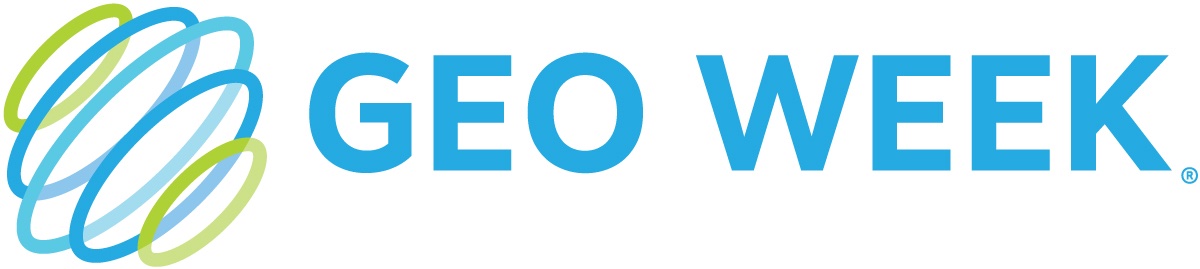
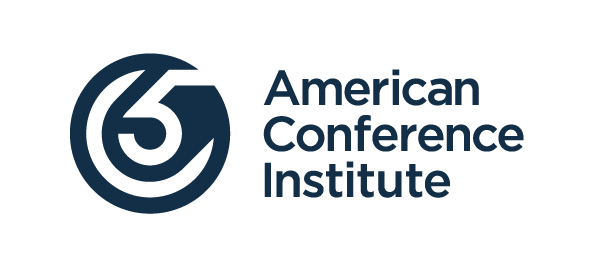
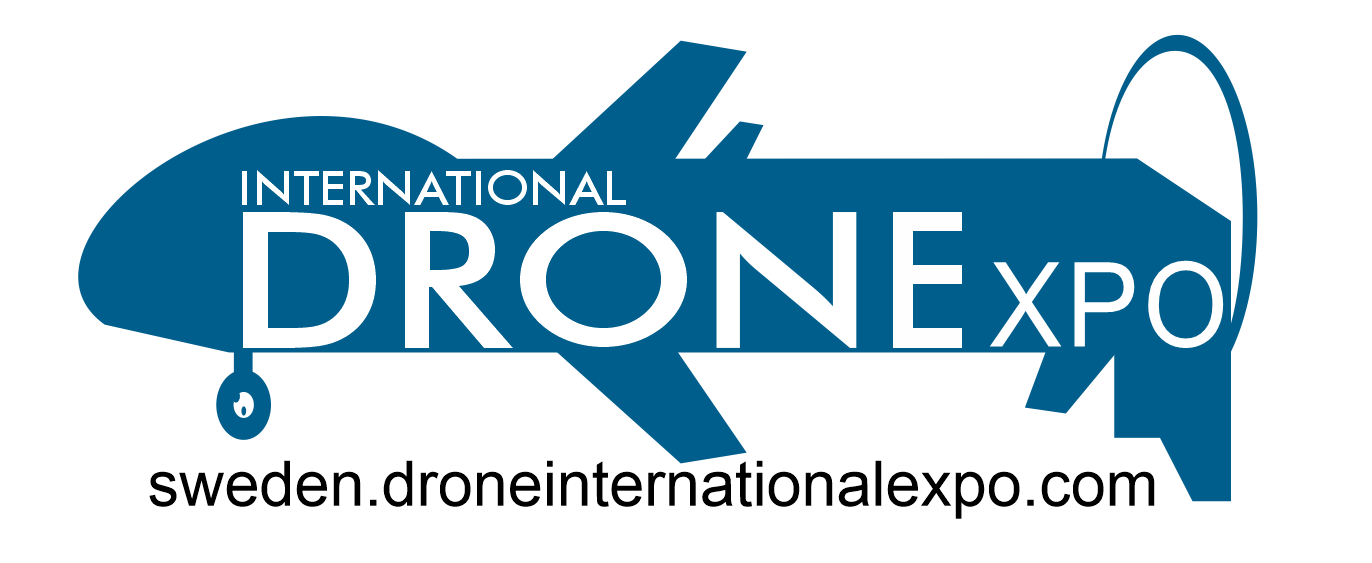
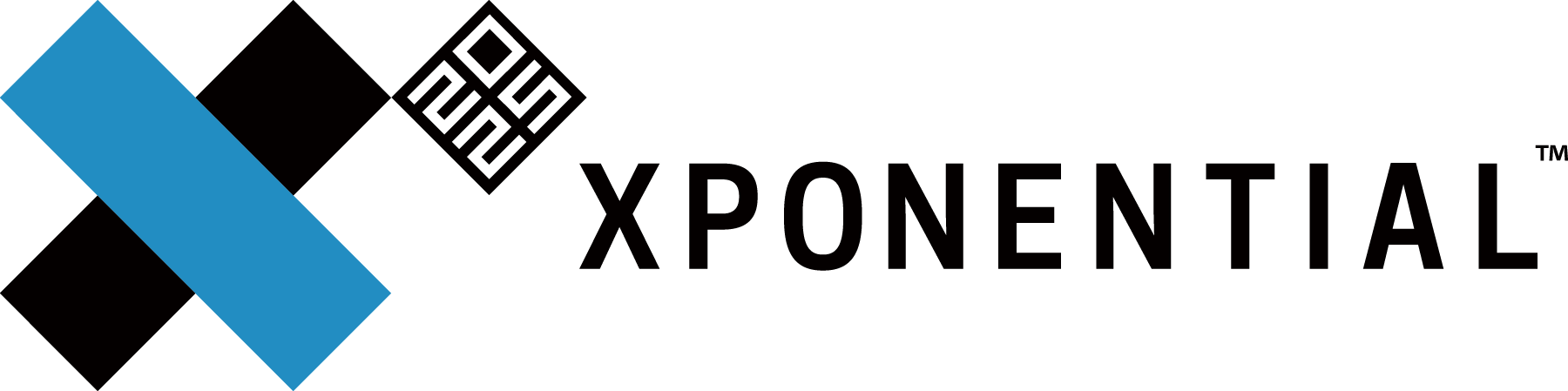
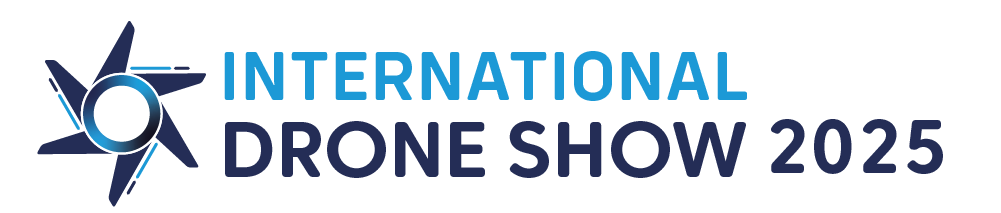