Thermal imaging
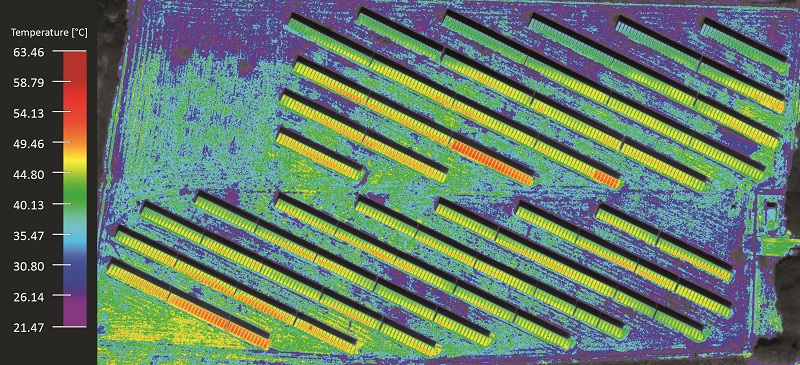
Rory Jackson investigates the factors driving developments in thermal cameras and the advances suppliers are making with the technology
Looking at the various inspection and monitoring missions uncrewed systems carry out these days, it soon becomes clear that thermal imagers have become central to almost every one. Measuring for thermographic differentials across objects, infrastructure and terrain reveals details that are invisible in the visible light spectrum or from 3D Lidar scans, and this can be critical in some circumstances.
For instance, aerial photography taken in the long-wave infrared (LWIR) wavelengths of 8-15 µm can show where water is concentrated or absent across swathes of land, which can be vital in agricultural and wildfire monitoring. Also, as contiguous planes or pieces of solid material such as metal or stone will exhibit some homogeneity (or at least continuity) in visible light, thermal cameras can reveal cracks or micro-fractures in structures; they can also be used in aerial archaeology by generating thermal ‘shadows’ of features below ground.
Mid-wave infrared (MWIR) cameras meanwhile are growing in use, in defence missions where their higher thermal sensitivity than LWIR cameras helps give military forces an advantage over their enemies in spotting distances for ISTAR (intelligence, surveillance, target acquisition and reconnaissance). They can also be used to monitor chemicals facilities for leaks, as that creates pressure differentials and in turn temperature differentials that can be seen in the mid-wavelengths (3-8 µm).
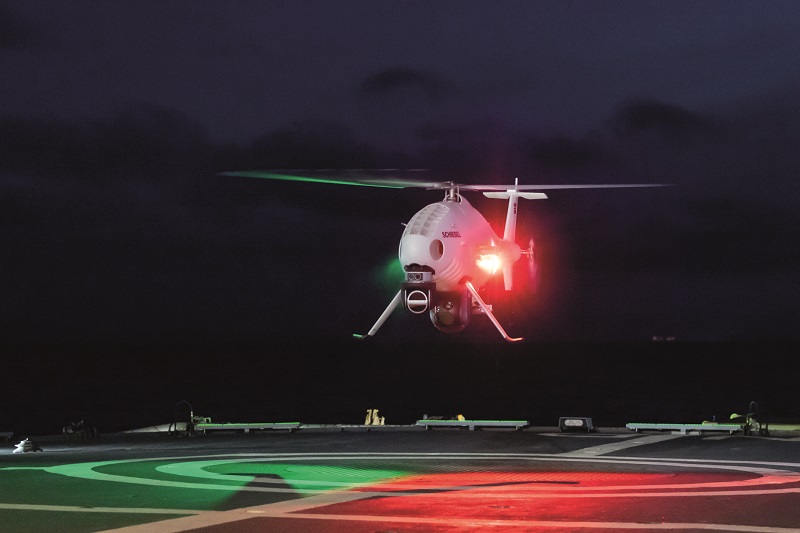
Multi-role sensors
In today’s uncrewed systems market, a single IR sensor is increasingly expected to be used across different missions and applications, often as part of a multi-sensor gimbal or a nadir or oblique-oriented enclosure. It will typically be integrated alongside an EO camera, IR cameras sensitive to other parts of the spectrum, or other devices including laser rangefinders, acoustic measuring instruments and illuminators.
Military integrators for instance now generally demand multi-role EO/IR gimbals for assessing the condition of strategic assets such as bases or ships, search & rescue operations, or day and night border monitoring over water and land. As well as having useful vision in the dark, thermal imagers are also very sensitive to daylight movements of water such as wakes and splashes, so they are proving useful in maritime surveys.
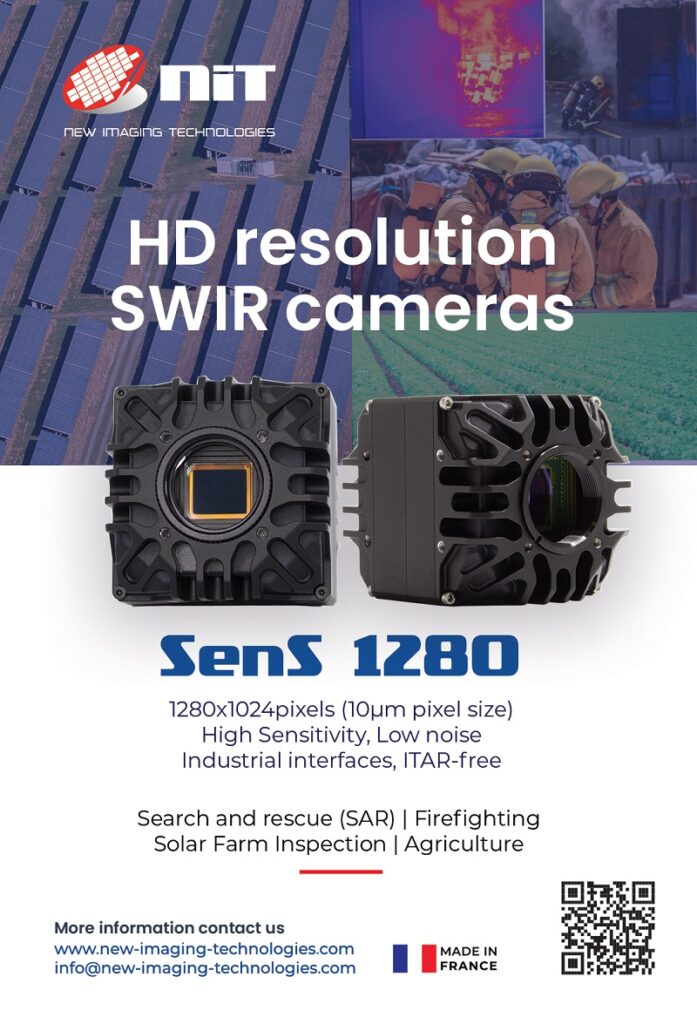
While commercial operators might not think of themselves as being multi-role operators in the way defence users do, many inevitably use their thermal sensors across a similarly broad range of applications. An energy company for instance might perform corridor mapping of stretches of power lines on one day, fields covered in solar panels on another, and a large structure such as a power station on the next.
Similarly, an oil & gas company carrying out autonomous pipeline inspections might need a gimbal with both a LWIR sensor for assessing the condition of a pipeline and an MWIR for searching for leaks and identifying any chemicals being leaked.
Given the high prices of payload sensors, users will often have only one or a few gimbals that they swap across different vehicles to suit the mission. Inspecting tall structures for instance will benefit from using a multi-rotor UAV that can hover, ascend and descend while maintaining a GNSS position.
Long and wide-area surveys are better served by fixed-wing UAVs, but different users will have preferences between, say, a catapult-launched aircraft in need of sensors that can survive the high g-loads and the shock of deployment, or VTOL-transitioning craft that undergoes less shock but needs a lighter gimbal because of the endurance lost during ascent and descent per gram of extra weight.
To serve all these users, payload manufacturers work on cutting out weight wherever they can, by using higher strength-to-weight grades of aluminium and magnesium (and in some cases carbon composites) in structural components in the gimbals and thermal camera housings. They design their products as one-size-fits-all solutions in terms of being both light and strong enough for as many vehicles as possible.
Also, in seeking to cover the different applications and the gathering of different data types at once, multi-spectral imagers are proliferating. As well as the crossover between LWIR and MWIR, combining imagers built for other bands such as NIR (near-infrared) can enable precise temperature readings of very hot surfaces when monitoring solar farms, for example, or detecting bruises or other damage to farm crops.
And while such combinations enable the latest advances in IR sensors to be exploited, arguably most of the more dynamic and important changes in thermal imaging are coming from the accelerating use of edge computing and AI technologies to enhance the value of data gathered from thermal monitoring and inspection tasks.
AI in maritime thermography for instance can distinguish between people, marine creatures and different kinds of boats with high fidelity. Alternatively, in wildfire monitoring, AI can produce geo-referenced maps of fire outlines or hotspots, with thermal imagers able to see where fires are still burning under trees or smoke, or otherwise hidden from the human eye.
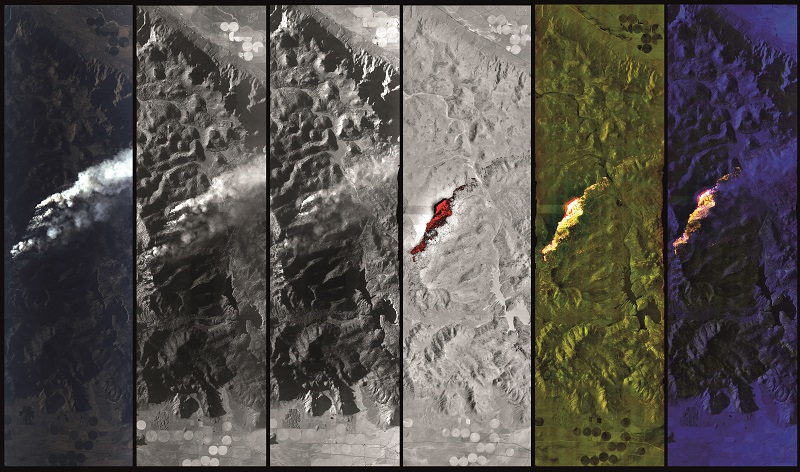
Combining thermal imagers with NDVI (normalised difference vegetation index) sensors can also allow flooding to be mapped autonomously, detecting and drawing lines around the perimeters of where water is present.
Autonomous vehicle applications present a valuable motivator for thermal sensor manufacturers to enhance their AI offerings. In principle, a machine can now be trained to recognise features in thermal imagery faster than a human. Imagery captured in the IR spectra can be invisible to people lacking at least several months of experience in thermal analysis, and by extension an autonomous gimbal can track and react to moving objects quicker than a remote operator, even if the latency in control signals between a UAV and its ground station was lost somehow.
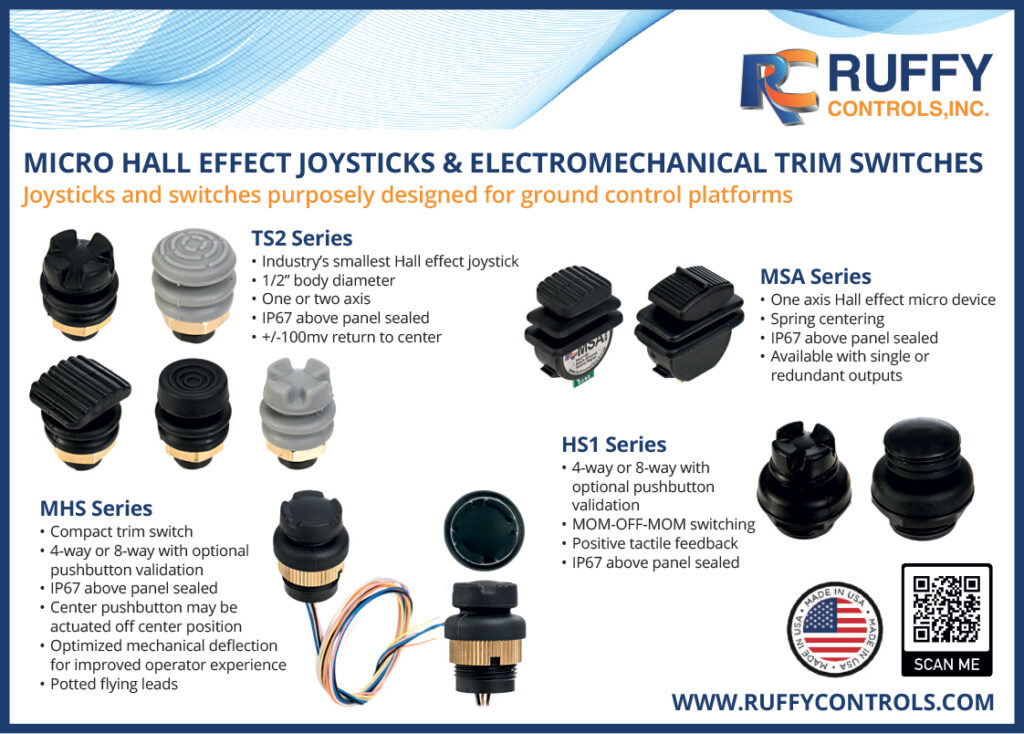
That means gimbals for uncrewed thermographic surveys often integrate powerful GPUs for onboard image processing and AI, with many manufacturers even graduating to the latest SoC (system-on-chip) for providing packages of intelligent, context-dependent capabilities with their sensors.
Coupling them with the imagers eliminates the risk of losing those capabilities if, say, a gimbal is installed in a different uncrewed vehicle without those processors, or if a system that relies on cloud-based processing is jammed or loses its data links for other reasons. It also enables fast processing and outputting of analytical reports that can be shared with other interested parties – a vital feature for disaster response and some defence work.
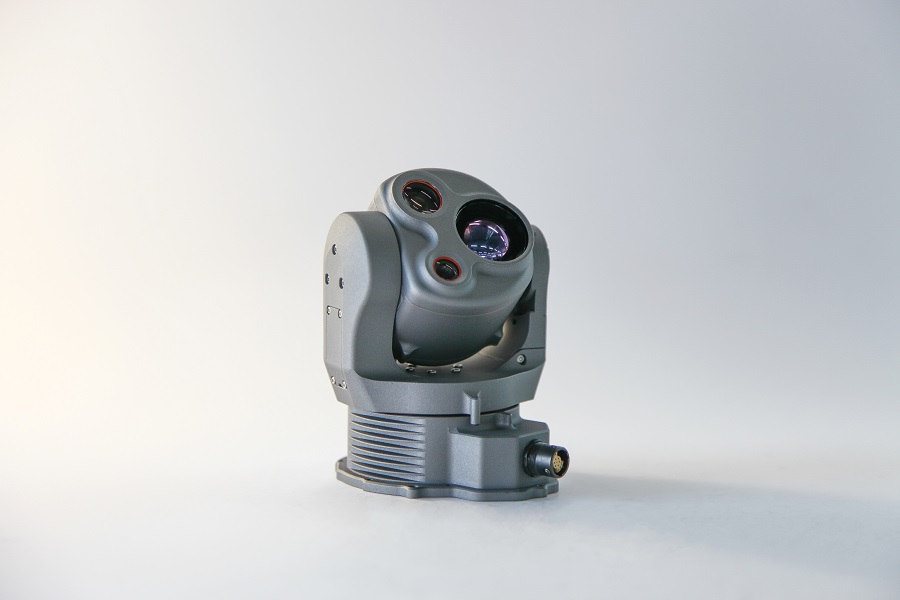
SWaP
While thermal camera r&d continues to develop detectors with higher resolutions than the widespread 640 x 512, and narrower pixel pitches even than the 12-17 µm found in current thermal imagers to achieve better image quality, uncrewed systems engineers are largely demanding thermal cameras with less weight, smaller sizes and lower power consumption, far more than they want improvements in image quality.
This is largely because uncrewed vehicles integrate an increasingly wide array of sensors and electronics.
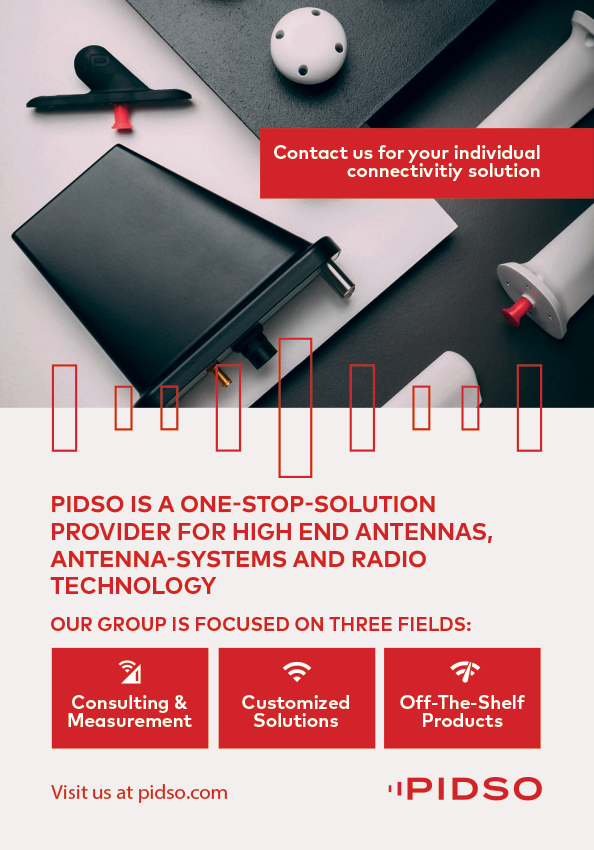
For example, UAVs could soon be expected to carry two EO/IR/laser gimbals, GNSS-IMU navigation systems, optical or radar solutions for GNSS-denied navigation, an SWIR camera for navigating through fog during take-off and landing, multiple radios for redundant real-time downlinking, and multiple transponders for air traffic compliance.
To reduce the SWaP taken up by thermal imagers, companies reserve their r&d resources for developing detectors with narrower pixel pitches. While (as mentioned) a smaller pixel pitch can give better image quality, the more important benefit for system integrators is that, when contrasting two thermal cameras with the same resolution, the one with the smaller pixel can be made lighter and more compact.
Certain applications will prove more suited for thermal cameras with longer range – as mentioned, MWIR cameras are in high demand among military integrators for this reason – but designing cameras for range often drives up the size, weight and power needed for the lens, which is already one of the biggest components in a camera.
An uncooled LWIR camera might be highly sensitive but it will still need a large-aperture lens to achieve long-range sensing. Lenses of f/1 to f/1.2 are often used in LWIRs for uncrewed systems (the f-number being the ratio of the focal distance to the aperture size, and a measure of the clarity and speed of the device).
Cooled UAV MWIR cameras however, being extremely sensitive to minute thermal differentials, can work using lens apertures of f/4 to f/5. That reduction in the size of the lens in turn reduces the size of the gimbal or other multi-sensor housing.
In addition to accounting for a thermal camera’s physical peripherals, some companies are increasing the power efficiency of their thermal cores by reducing the time to first image, known as activation time. As with any vehicle electronics, it is wise to power-down thermal cameras when not in use to save energy and enhance endurance, but it can take several seconds for them to initialise after being reactivated.
Minimising the time to obtain the first image is a critical benefit for uncrewed vehicles and battery lifetimes, potentially adding several minutes of endurance to an otherwise 20-30 minute flight.
Different approaches are taken to solving this challenge, the primary ones being designing the thermal core’s system architecture and hardware components to enable a quick power-up, or optimising the image correction algorithm in the hardware to converge on a reasonable image quality more quickly using the raw thermal data.
Cooled MWIR cameras again have an advantage here over LWIRs. A key hardware element extending LWIRs’ time to first image is their construction around micro-bolometer detectors, which convert IR radiation to a change in electrical resistance in their detectors.
The detectors used in MWIR cameras however measure photons, much like most EO cameras, and convert them into a measurable electrical signal. This conversion is highly quantum-efficient and is hence very fast, enabling their signals to be integrated much faster than the cameras’ frame rates. They can therefore initialise quickly, enabling them to be turned off and on again conveniently during flight.
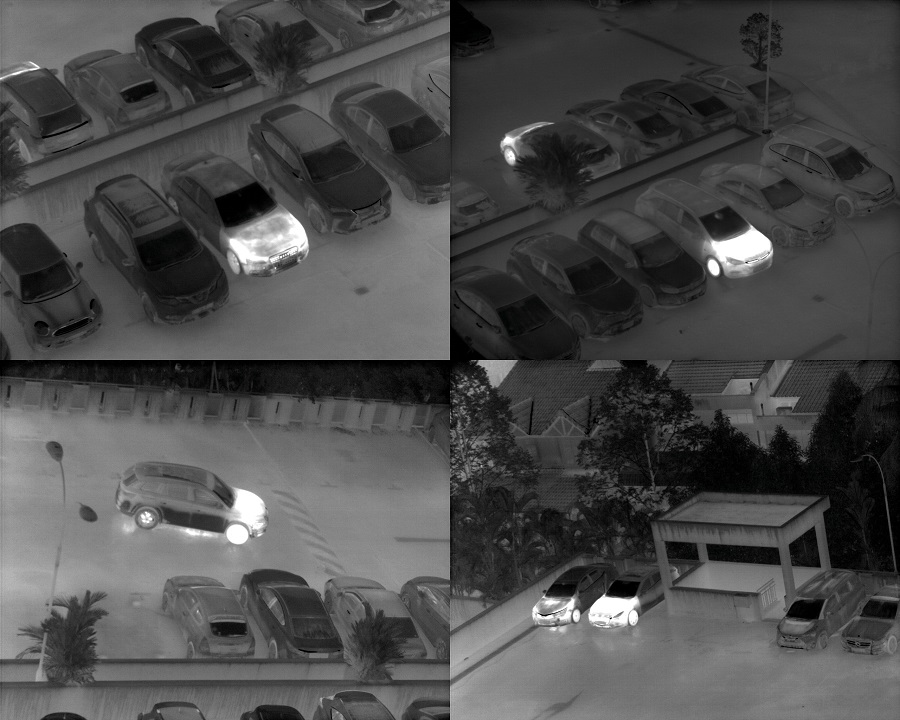
Manufacture and calibration
Thermal cores tend to be designed much like any closely integrated electronic system, so these days their construction and quality control are handled largely by automated systems.
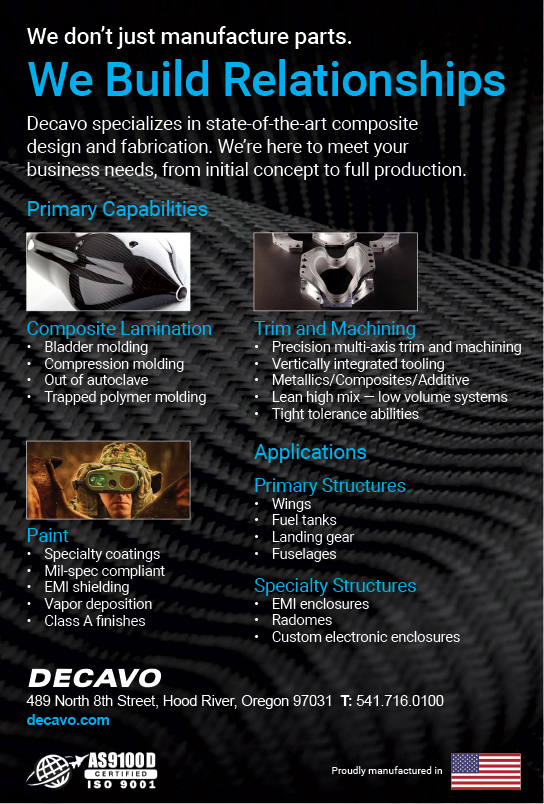
The system’s detector, amplifier and signal processing units are installed on circuit boards, which are then stacked together along with the spectral band filter needed for the customer’s desired functions and connector layouts. The stack is then typically enclosed in a housing, except in OEM integrations.
Once the core is constructed, calibration can begin. This is a process by which the readings from each camera are checked and correlated against known, pre-established temperatures and objects, to tune them for accurate measurements when in use.
The exact process varies between camera manufacturers. In some instances, a black metal plate is placed in front of the sensor to serve as a blank reference image, upon which the sensor’s imperfections can be viewed through the output stream of its photography or video.
Different arrangements of reference objects and other equipment can be applied during the calibration process to account for factors such as the environmental temperatures the camera will be flown in, or the temperatures of objects to be routinely inspected. System integrators must make these requirements clear during discussions with thermal camera suppliers though.
Broadly, testing new designs or periodic samples of cameras off production lines is performed in line with established standards such as Mil-Std 810 for shock and vibration or 416 for electromagnetic compatibility. In future, design compliance with aviation standards such as DO-178C are likely to be increasingly important.
Thermal AI
Although AI as a term has various definitions and categories for thermal imagers it principally means perception-based algorithms such as object detectors, classifiers and trackers.
Thermal imaging also offers the potential for more effective use of such algorithms than when they are applied in optical vision systems, as temperature differentials can more clearly demarcate the boundaries of people, animals, human-built structures and vehicles than colours and lines in visual imagery.
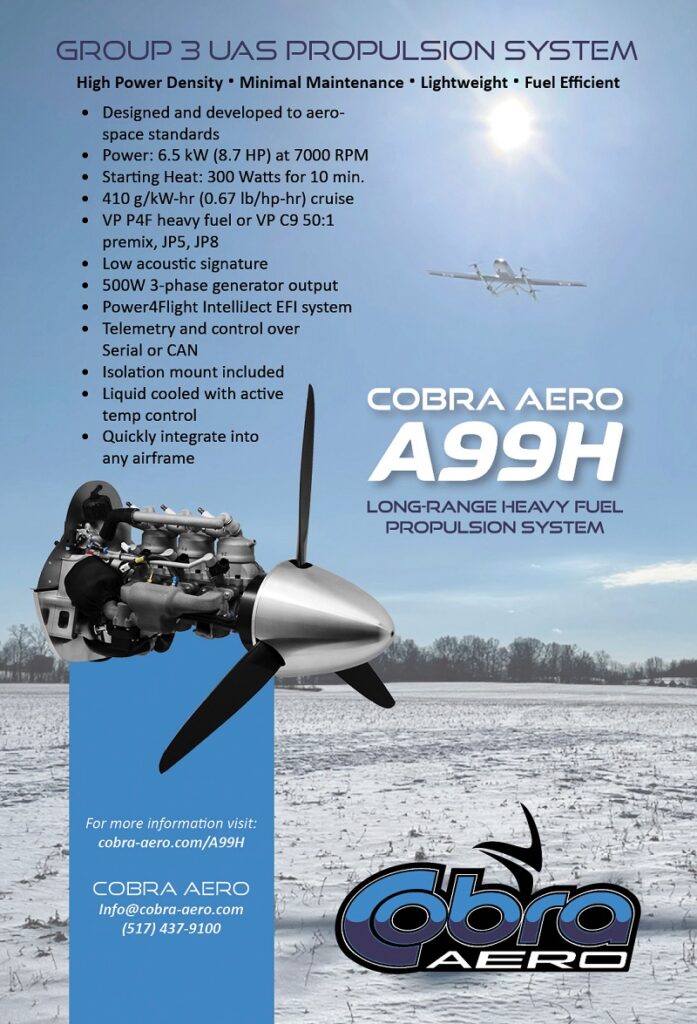
This advantage is particularly pronounced when fog, sunlight or waves obscure the objects in such images, with LWIR being better at seeing through them than EO and MWIR systems.
Developing and training such algorithms through machine learning is expensive, and can take years, but once developed they can be used across different cameras, vehicles and missions to great effect. They offer high rates of success in terms of accurately recognising and tracking different objects, throughout countless possible permutations of thermal patterns, shapes, distances and so on.
As with visual image-based detection, classification and tracking, machine learning for thermal imagery starts with a neural network, which is the framework of an algorithm that can be trained into an application-specific model. They include air-to-ground applications such as security forces monitoring road traffic for a particular vehicle type, or maritime scanning for boats engaged in illegal activities or people who’ve fallen overboard.
They also include driverless mobility applications such as autonomous braking when they detect and recognise the thermal signatures of pedestrians or animals on the road ahead.
After selecting a neural network, training data must be fed into it. This data is often difficult to acquire, especially for thermal AI models.
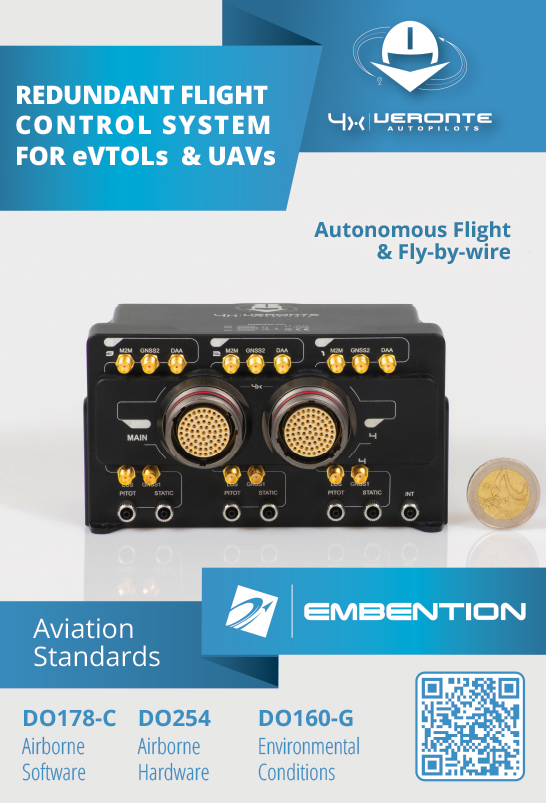
A few datasets are available as open source downloads, with potentially millions of thermal images to train networks in recognising and extracting features from application-specific targets. However, the quality of one image dataset versus another’s can be a huge differentiator in the consistency and accuracy with which object recognition, classification and tracking is performed.
The uncrewed industry’s leading providers of intelligent thermal imaging solutions therefore generally began their AI development programmes years before the datasets existed, so they have trained their models on unique ones tailored to mission-specific use cases.
Building up such a dataset can be lengthy and expensive. For instance, to train a UAV gimbal to detect and classify any type of vehicle or lifeform on the ground while in flight can require taking millions of images from the air – not just in LWIR and MWIR, but also in EO, as the latter can help confirm correct classifications by the model during development. As most users will use EO and IR simultaneously, it is good practice to train AI to use both at once and in equal measure.
The result of all this should be several million annotations – images with bounding boxes or polygons around the target inside each image, with a machine-readable label indicating exactly what the target is. A company with such a dataset can search for groups of features such as annotations of cracks or dents in metal structures, and rapidly train a blank neural network into a mission-specific AI model that can be embedded on an EO/IR gimbal’s SoC.
It is worth noting here that the widespread training of AI algorithms using 640 x 512 resolution LWIR image datasets is a major reason why there isn’t stronger demand for higher resolution thermal cameras. As autonomous recognition, classification and gimbal tracking of different objects can now be accomplished with a success rate close to 99% using streams of 640 x 512 thermal photography and video, there is no significant pay-off to be derived from higher resolutions in terms of vehicle intelligence or capabilities; the few remaining percentage points of accuracy can be gained through algorithmic optimisations.
Right now, the roadmap for thermal AI lies not so much in further development of the AI algorithms but in identifying new and valuable ways of using them, as well as updates of the software to keep them compatible with the latest SoCs, particularly for multi-role uncrewed systems that need multiple simultaneous analytic capabilities per mission.
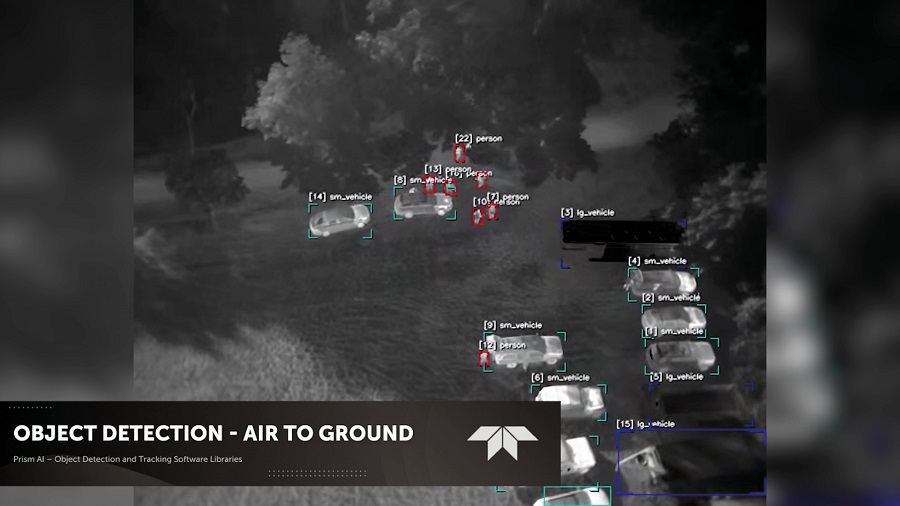
Edge computing
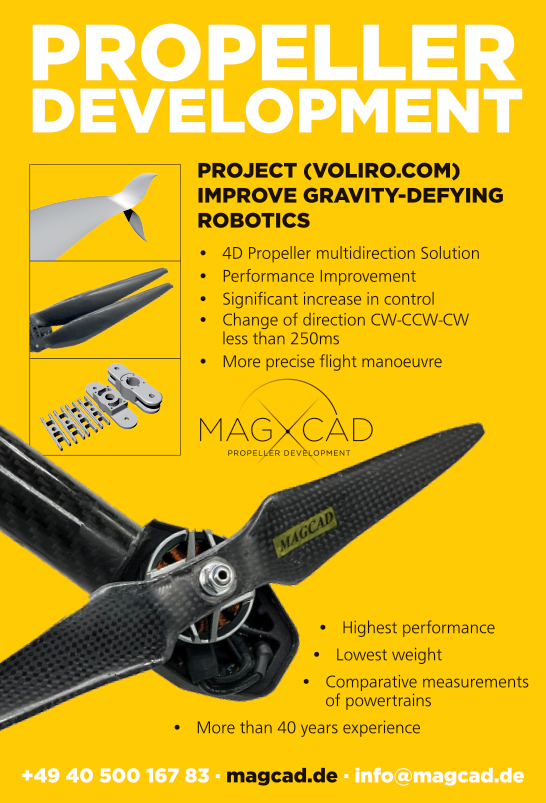
Companies such as Qualcomm and Nvidia stand out among thermal imaging and other sensor suppliers for the SWaP-optimised SoCs they produce. These generally combine a GPU with a CPU, memory, comms, power management and other systems in a tightly integrated form suited for packaging inside a gimbal without it presenting a glaring point of mechanical or thermal vulnerability.
High computing power is critical for real-time processing and analysis of thermal monitoring data, given that modern IR scanners and video cameras are capable of frame rates of 30-60 fps or higher. Popular platforms for thermal image processing include Qualcomm’s RB5, based on its QRB5165 processor, which is capable of up to 15 trillion floating point operations per second (Tflops).
Among other components it includes eight of the company’s Kryo 585 CPUs, each being a 64-bit system with up to 2.84 GHz clock speed, as well as its Adreno 650 GPU, which is built for up to 343 Gigaflops of performance in 64-bit operations, or up to 1372 Gflops in 32-bit operations.
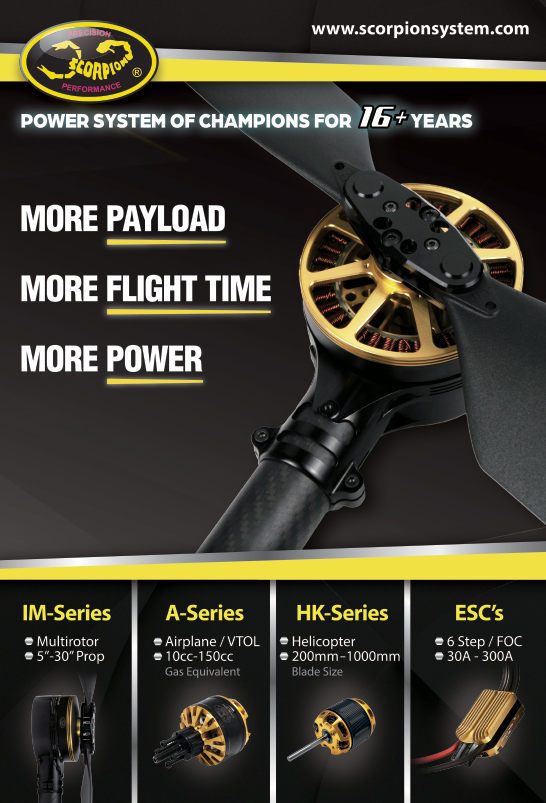
In the future, Qualcomm platforms are expected to remain prevalent in turnkey thermal imaging payloads, particularly with newer generations of the RB5 being released at the time of writing. However, thermal systems developers plan to integrate Nvidia platforms as it issues more powerful SoCs and because some engineers prefer working within the Nvidia ecosystem.
For reference, the next generation of the Qualcomm RB5 is expected to perform nearly 50 Tflops, such is the necessary degree of processing power needed for running the kinds of computationally intensive perception software models that are now rapidly becoming a mainstay of thermal imaging products for uncrewed systems.
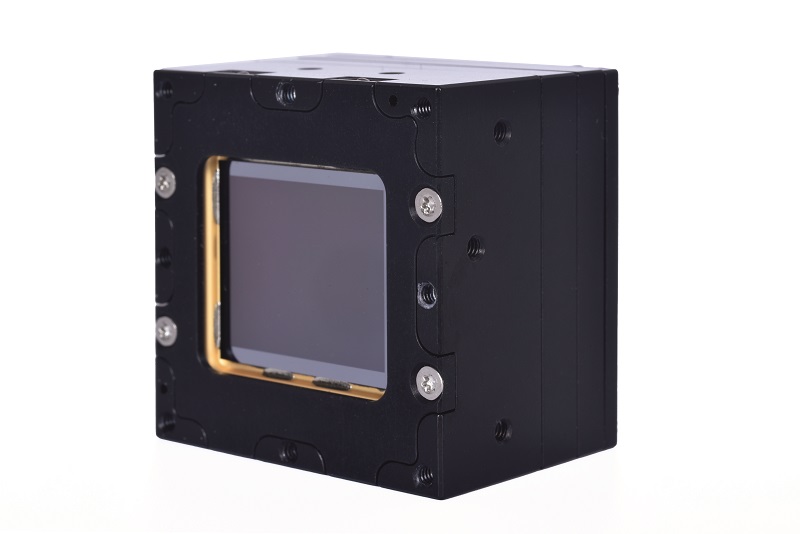
System integration
As coupling a SWaP-optimised thermal camera with a powerful edge computing system becomes increasingly important for maximising the capabilities and actionable information that can be derived from a thermal core, it is increasingly common for thermal core manufacturers to engineer their products with an array of interfaces, to make it easier for system integrators to connect and implement edge compute components seamlessly.
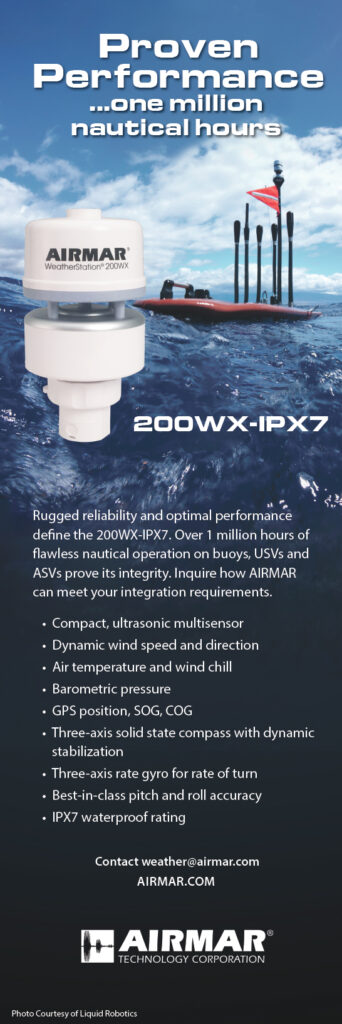
For example, the MIPI-CSI2 camera interface is gaining widespread popularity as a protocol for connecting image sensors to processors. It costs less than other interfaces, is relatively easy to program, and uses minimal CPU resources or power to implement. This latter property also means MIPI ports produce little heat, so they don’t overburden the thermal core’s cooling system.
Thermal cores built with numerous MIPI, Ethernet and serial ports make life far easier for thermal camera and gimbal engineers. With so many interfaces ready to use, complete imaging systems can be scaled up or down to suit the extent of edge processing and AI for different users and markets.
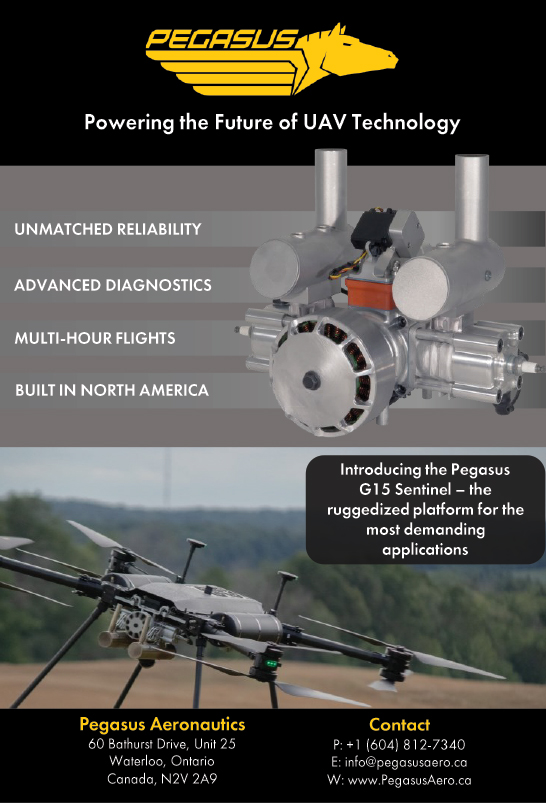
Interface-heavy cores can also be integrated with processors for fusing thermal data with other sensors more easily. In driverless car applications for instance, thermal video might need to be tightly fused with 3D Lidar point clouds, EO video, radar scans, inertial data and GNSS data in real time, to calculate steering and braking outputs, at least as fast if not faster than the 400-600 ms reaction times of human drivers.
Future prospects
While the industry’s new-found drive for SWaP-optimised thermal cameras might not seem groundbreaking, given how widespread it is across uncrewed vehicle subsystems, it is important to note that only 4-5 years ago, there was no major drive to make thermal sensors smaller or lighter to meet the constraints of uncrewed vehicle integrators. The onus was almost entirely on the customer to make enough room in their vehicle.
The SWaP aspect is a recent phenomenon, so it could be a few years before the associated wave of r&d into how thermal cameras can be shrunk down, lightened or made more power-efficient starts to bear fruit, in the form of a new generation of miniaturised LWIR and MWIR cores.
In the meantime, the thermal sensor industry is likely to grow more intertwined with the edge computing industry through their common interest in the uncrewed and autonomous vehicle markets. Parameters such as the data bandwidths, frame rates, and mechanical and thermal properties of IR cameras will become important in selecting processor cores, memory,
I/Os and board-level components, as the SoC gradually takes its place over time as a primary component of a complete thermal imaging system.
Acknowledgements
The author would like to thank Art Stout and Dan Walker at Teledyne FLIR, Matt Lynaugh and Adam Lapierre at Overwatch Imaging, Frederic Aubrun and Marc Larive at Exosens, Adam Svestka at Workswell, and Evert Van Schuppen, Ryan Carter, Robert Grew and Bernie May at AVT Australia for their help with researching this article.
Some examples of thermal imaging manufacturers and suppliers
AUSTRALIA
Applied Infrared Sensing | +61 1300 557 205 | www.applied-infrared.com.au |
AVT Australia | +61 265 811 994 | www.ascentvision.com.au |
CZECH REPUBLIC
Workswell | +420 725 877 063 | www.workswell-thermal-camera.com |
ESTONIA
Threod Systems | +372 512 1154 | www.threod.com |
FRANCE
Exosens | +33 55 616 4050 | www.exosens.com |
Merio UAV Payload Systems | +33 428 3700 27 | www.merio.fr |
New Imaging Technologies | +33 1 64 47 88 58 | www.new-imaging-technologies.com |
Thales | +33 157 7780 00 | www.thalesgroup.com |
GERMANY
Kappa Optronics | +49 5508 974 0 | www.kappa-optronics.com |
ISRAEL
Controp | +972 9744 0661 | www.controp.com |
NextVision Stabilized Systems | +972 77 534 2041 | www.nextvision-sys.com |
Ophir Optics | +972 2548 4444 | www.ophiropt.com |
SOUTH KOREA
Unetware | +82 02790 7830 | www.unetware.com |
THE NETHERLANDS
Dronexpert | +31 630 303 015 | www.dronexpert.nl |
UK
BAE Systems | +44 1252 373232 | www.baesystems.com |
Flytron | +44 1227 634434 | www.flytron.com |
Micro-Epsilon | +44 151 355 6070 | www.micro-epsilon.co.uk |
Thermoteknix | +44 1223 204000 | www.thermoteknix.com |
USA
AeroVironment | +1 703 418 2828 | www.avinc.com |
CACI | +1 406 333 5010 | www.caci.com |
Infrared Cameras | +1 409 861 0788 | www.infraredcameras.com |
Obsetech | +1 628 900 3018 | www.obsetech.com |
Overwatch Imaging | +1 541 716 4832 | www.overwatchimaging.com |
PrecisionHawk | +1 844 328 5326 | www.precisionhawk.com |
Sierra-Olympia Technologies | +1 541 716 0015 | www.sierraolympia.com |
Sightline Applications | +1 541 716 5137 | www.sightlineapplications.com |
Teledyne FLIR | +1 503 498 3547 | www.flir.com |
PrecisionHawk | +1 844 328 5326 | www.precisionhawk.com |
UAV Propulsion Tech | +1 810 441 1457 | www.uavpropulsiontech.com |
VT MAK | +1 617 876 8085 | www.mak.com |
UPCOMING EVENTS
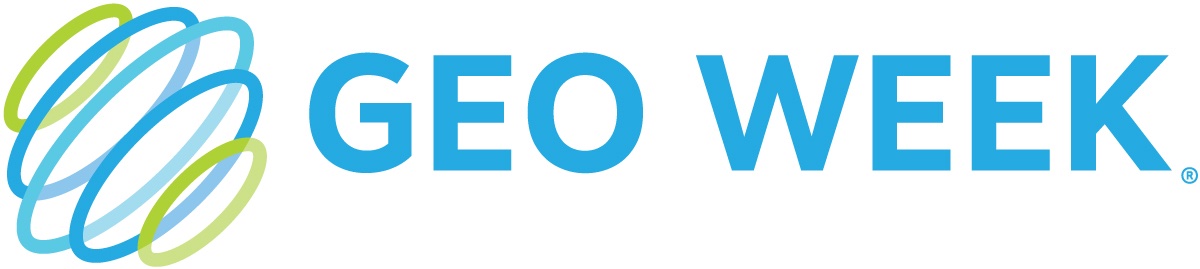
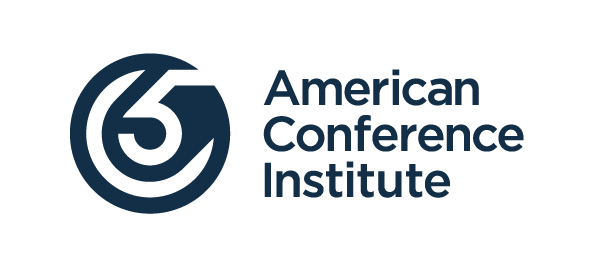
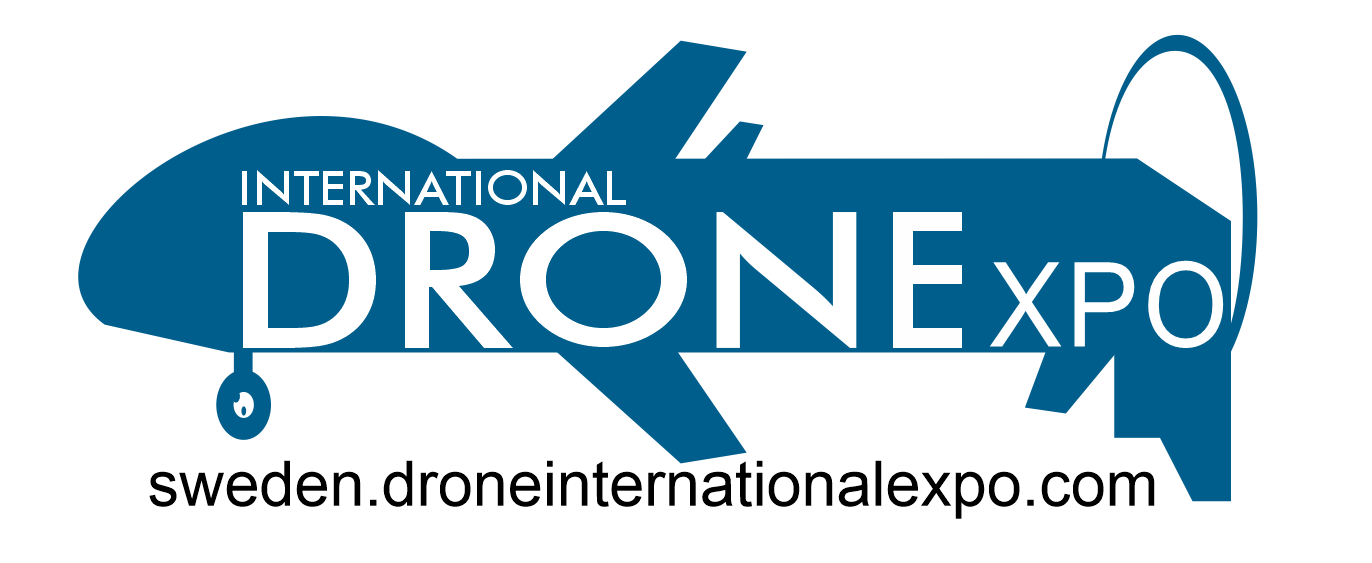
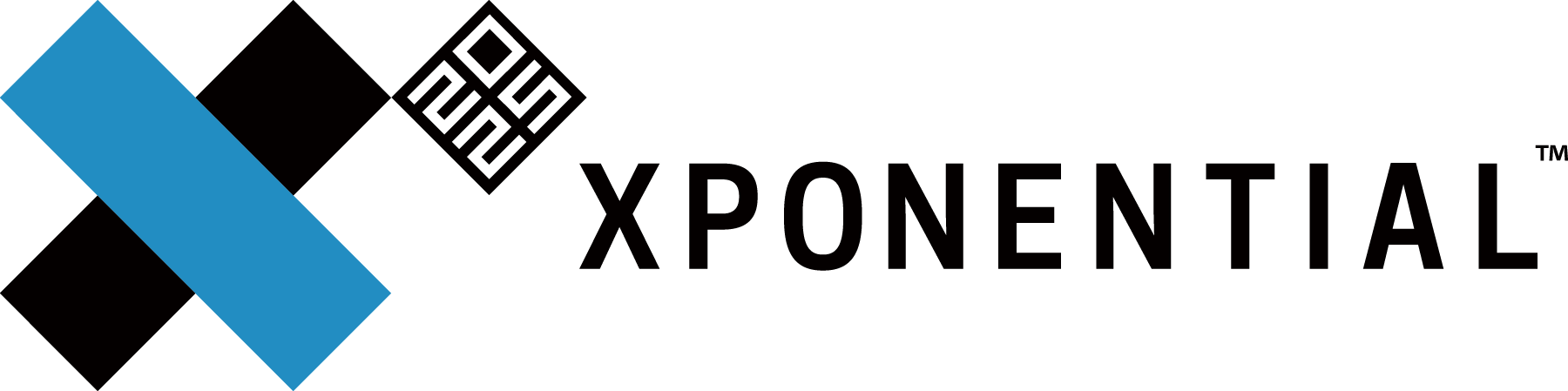
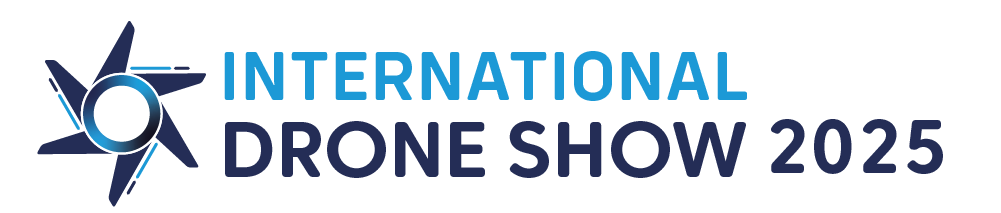