Swarming
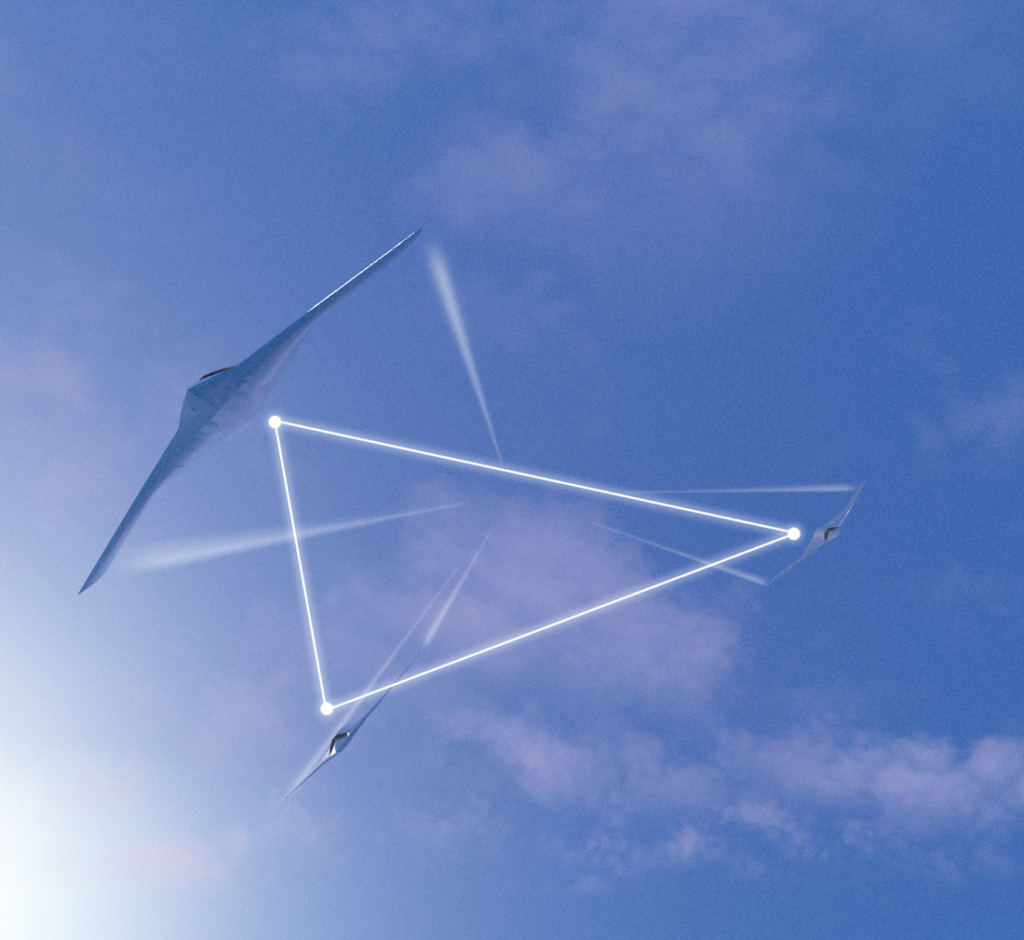
(Image courtesy of UAV Navigation)
Safety in numbers
Nick Flaherty examines new technologies and uses for UAV swarms
There are many types of swarm technologies for autonomous platforms, varying from dramatic displays of hundreds of UAVs to groups of driverless cars, to combined ground, air and sea platforms all working together autonomously. While they take different approaches to implement swarming functions, they highlight the advantages of autonomous systems working in concert.
New uses are also emerging for swarming, such as using multiple, small ground vehicles to replicate the operation of a larger system with more flexibility. In the air, the most obvious implementation is mass display, and the record is 2066 UAVs operating in concert.
The process for creating a show is quite straightforward. First, the design team creates a storyboard timeline showing the desired images and effects. These are then animated in a specialised piece of software that translates them into synchronised flight paths for each UAV. Complete shows are sent to the UAV via radio link from a ground control station (GCS) operated by a pilot.
Some UAVs are designed specifically for such applications, cutting out the cameras and adding high-power LED lights.
The design software lets users select graphics and special effects, and place them in a timeline, similar to those found in video-editing software. It calculates the flight paths of each UAV to ensure they don’t collide in the air, and generates a full 3D render of the show to ensure it looks exactly as intended. Each UAV is sent a unique program and the GCS monitors each one over a local, encrypted network to avoid hacking.
Early shows focused on large numbers as the lights on the UAVs were not very bright and did not accurately hold position. This meant the display was designed so the UAVs would fill a volume of space to illuminate it, and this required many units. Rather than draw a straight line with individual UAVs, a rectangle was drawn and filled with many units.
The latest platforms take advantage of much brighter LEDs and hold their position precisely by supplementing GPS with additional positional data sent from the GCS. This enables more creative flexibility and allows the same effect to be achieved with fewer devices to precisely draw lines in 3D with UAVs.
The more intricate the shape, the more craft are needed to create it. Audience size and viewing distance will also influence how many units are needed for the required visual impact.
The UAVs have multiple radios operating simultaneously away from busy wi-fi frequencies to ensure communications are maintained, even in busy and noisy radio environments.
Operations flow much better when each UAV has a full copy of the show, so they can be used in any takeoff slot. The UAVs indicate when they’re in a suitable location during placement in the grid, and the GCS software automatically checks flight readiness and placement. This makes it easier to swap out any UAV that is not ready for flight.
All of the UAVs coordinate with the global database to synchronise maintenance schedules and perform software updates, enabling processes such as replacing motors and batteries to happen wirelessly. This gives two people just 45 minutes to set up and fly 100 UAVs.
Autonomous swarms
Multi-UAV is an operation where multiple aircraft fly in the same airspace. In such missions, multiple UASs are operated from a GCS, where one or multiple users command and monitor the flight. Adapting the flight-control system on the UAV allows the sharing of state information between the aircraft and the GCS.
This needs to combine the status data of every aircraft during the multi-UAV operation with the traffic-display capabilities and automatic collision avoidance algorithms during the swarming operation to create a co-operative framework.
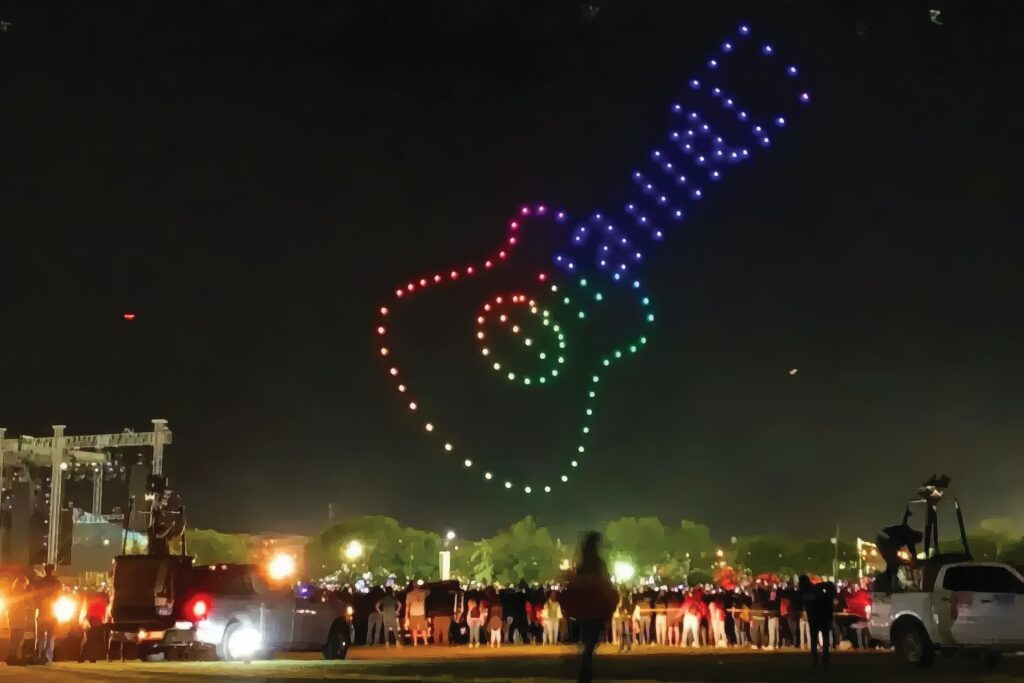
(Image courtesy of Verge Aero)
One important thing is that the objectives of the swarm vary significantly between different users and from mission to mission, so the key technology that goes into the autopilot of a UAV is to have all the elements in a modular framework.
For swarm operation, the autonomous aircraft do not rely on a continuous connection to the GCS, but are coordinating their position in space, and avoiding other aircraft within the swarm and out of the swarm. This can be for quadcopters in a relatively small area of a few square kilometres, or for larger aircraft that could be separated by hundreds of kilometres.
Every swarm needs customisation for integrators and users to introduce their thinking into the system, and the mission-control computer (MCC) in the UAV has evolved to support swarm logic.
As these requirements are evolving rapidly, the design of the MCC must adapt quickly. The radio connectivity needs to be technology agnostic, as this could be cellular 4G or 5G, a local link or a satellite link for longer distances. However, a common theme is to reduce the data-throughput requirements of the link to allow more UAVs to operate in a single swarm. However, swarms can be linked via the GCS. Linking back to the GCS and then to another GCS with another swarm can extend the whole operation across a wider area.
Within the swarm, the autopilot in the UAV runs a coordination algorithm that allows for both complete and distributed operation. Each vehicle can have the flight plan for the whole swarm, or its own portion of the plan. The aircraft coordinate via specific checkpoints within the mission to synchronise the operation, and these checkpoints are automatically generated by the MCC.
This allows an operator on the ground to provide a single command to the system, and from that point the rest of the calculations can be performed onboard, such as instructions to fly relative to a neighbouring UAV. Once it has received that message it knows what it has to do with high-level commands from the ground and the system can manage itself.
There is also the issue of dealing with third-party aircraft in the airspace around the swarm. These can be collaborative aircraft that have ‘no fly’ zones defined around them so that the members of the swarm fly relative to them; for example, with a crewed aircraft whose position is fed into the vehicle-to-vehicle network and shared with all the UAVs in the swarm.
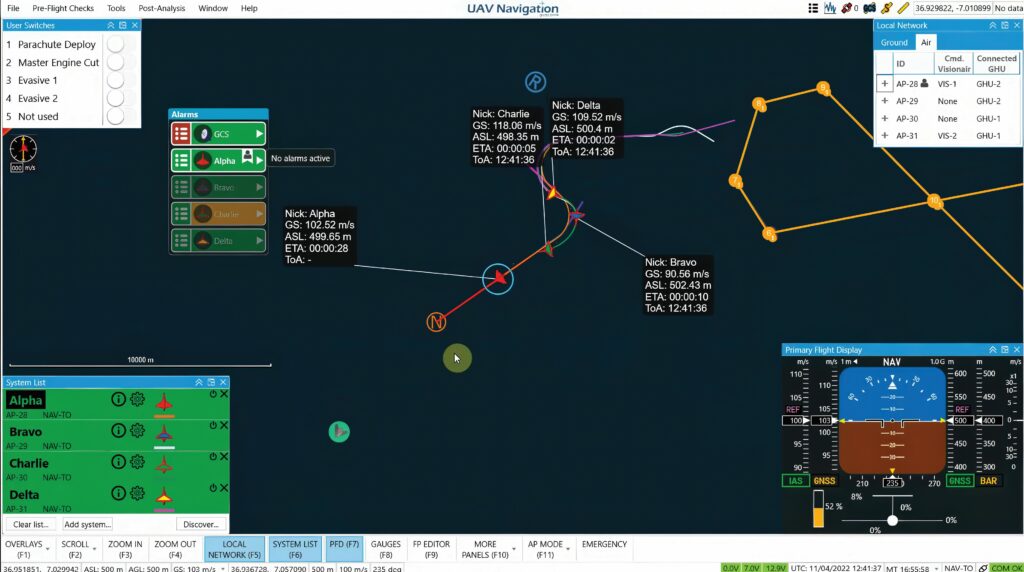
(Image courtesy of UAV Navigation)
For non-collaborative aircraft, the methodology depends on the environment in which the swarm is operating and the sensors the UAVs have, such as cameras. The data from these sensors needs to be fed into the MCC, where it can be shared between the UAVs in the swarm and connect to other GCSs.
The communications link is vital. UAVs could be hundreds of kilometres apart in a swarm and linked by satellites, with several aircraft providing surveillance data and linked back to uncrewed fast jets that can cover a distance of 50 to 60 km in a few minutes.
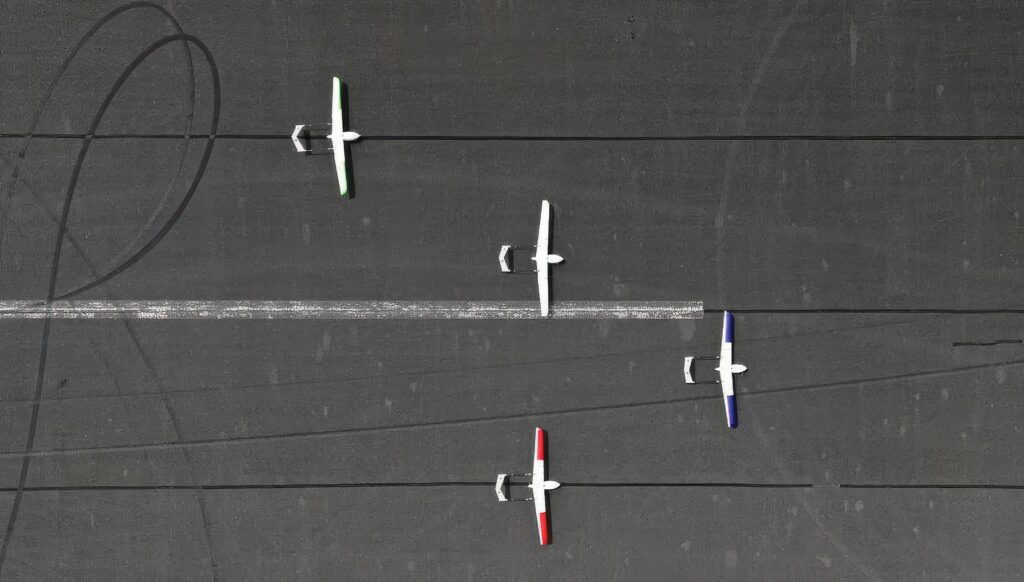
(Image courtesy of Applied Aeronautics)
The key is to know the rate for each type of information. With a swarm the ground operator is no longer a pilot but a mission coordinator, managing 20, 30 or 40 aircraft. The key is to increase the amount of data that can be stored in the UAV and not transmitted to the ground all the time. There also needs to be a way of preventing duplicated data, such as the same data from different UAVs, being transmitted multiple times.
For a heterogenous swarm, such as uncrewed surface vessels (USVs) at sea, the coordination of the swarm is managed by linking the GCSs.
Using a swarm can also improve the resilience of a mission. A mesh network with connections between the UAVs in the swarm allows for any errors in a sensor on one aircraft to be shared with the others, with algorithms using this error data to improve the navigation of the other craft.
There is an advantage in the payloads, which can be distributed between multiple UAVs rather than requiring a larger aircraft. For example, four small, 25 kg UAVs flying together can each carry a payload, rather than one large, 100 kg craft. This can provide more data from different points of view, such as triangulation to find a radio source. Using smaller aircraft of less than 25 kg has less regulatory requirements than larger ones.
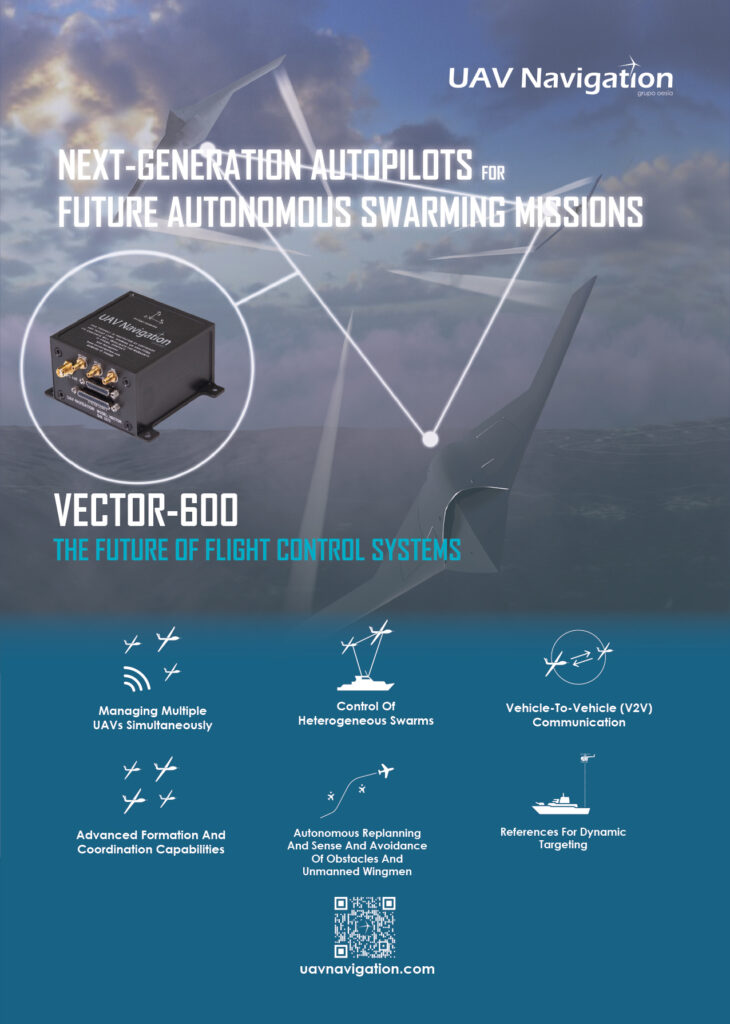
This has been tested in trials of UAVs with a takeoff weight of over 100 kg flying in autonomous formation. They achieved speeds of over 300 kts with separations of less than 200 m.
Another trial in the UK saw four fixed-wing Albatross UAVs teamed to carry out a mission to detect and jam a radio frequency emitting from an enemy target. Each UAV was equipped with a mission system, and it used multispectral machine vision and new search algorithms to respond to its environment, and to the other UAVs in the swarm.
This builds on a series of autonomous flight trials that took place last year using an adaptable autonomy software framework to build intelligent behaviours deployable on multiple platforms.
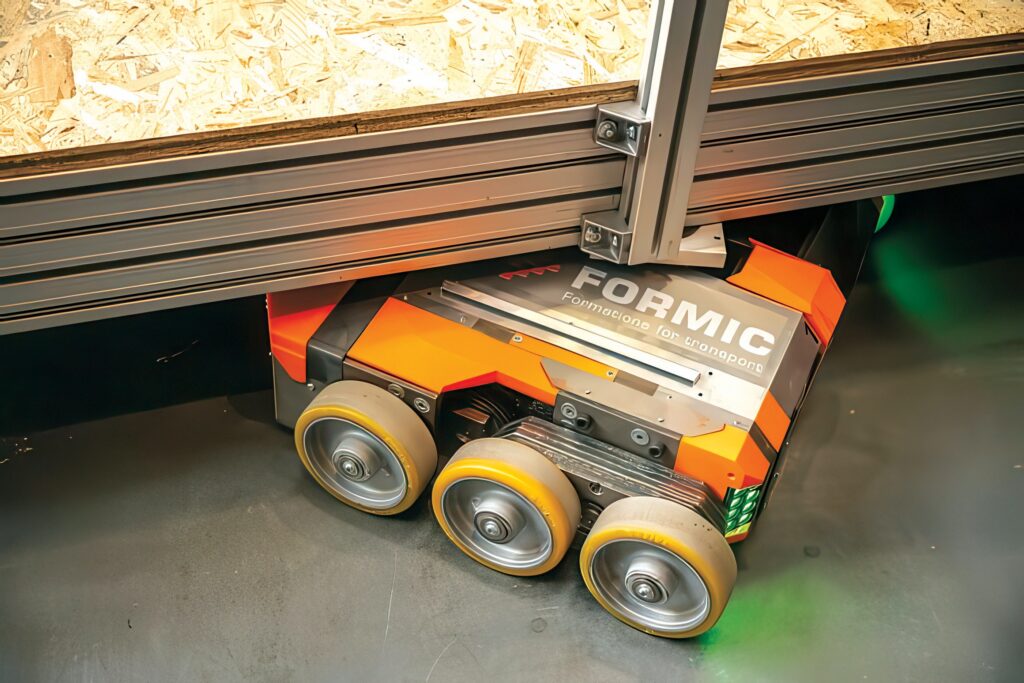
(Image courtesy of Karlsruhe Institute of Technology)
The second-phase trials now include swarming, or autonomous platform-to-platform teaming, which can target a position from a greater range and lets the platforms cover more ground with confidence in target identity and location.
Ground vehicles
Swarms of small, autonomous ground vehicles are being used to replace large production machinery. However, high weight and limited space in plants often make it difficult to assemble and disassemble big machines, or to reposition them, and this is often done by hand using heavy-duty rollers. Now, a semi-automatic transport system operates as a swarm for the replacement of production plant.
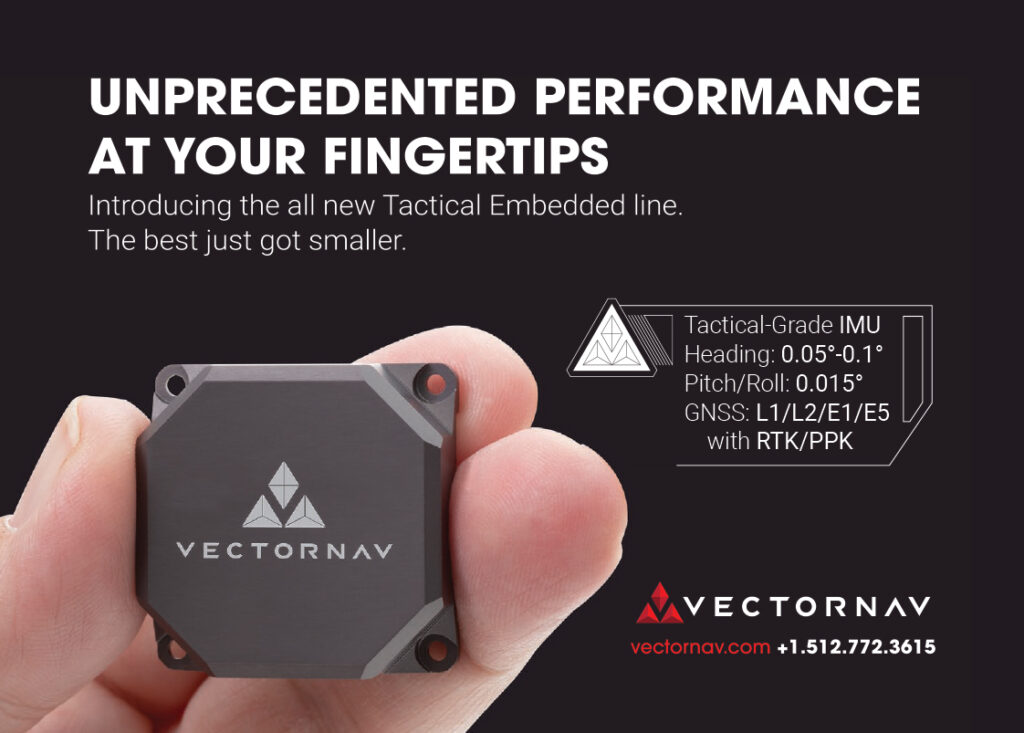
The system consists of several separately-driven vehicles that together can transport a weight of up to 40 t and move it semi-automatically. Up to 15 units can be coupled and use cameras for positioning with radio for synchronisation. The swarm combines the mechanics of the individual vehicles and the control software.
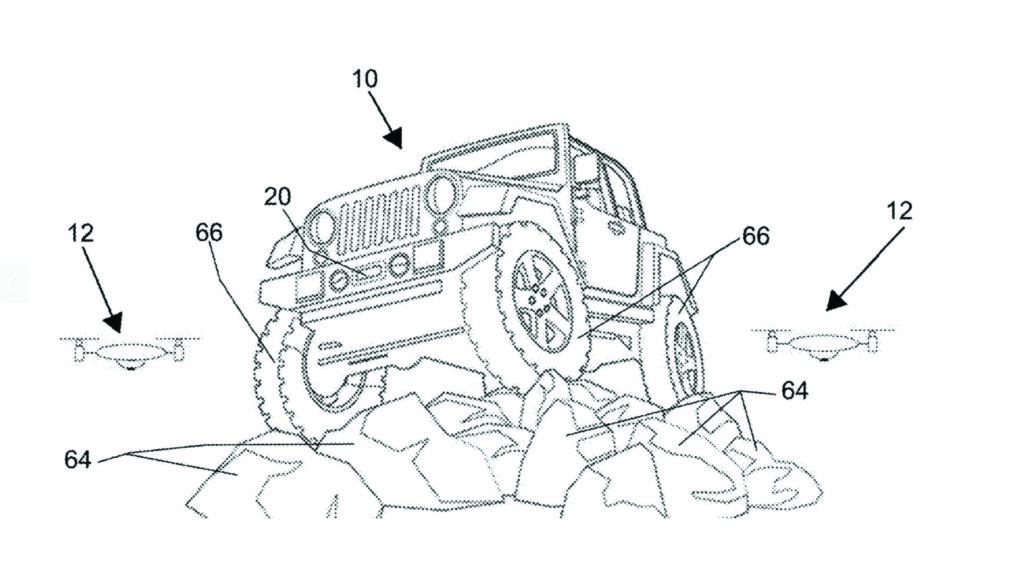
(Image courtesy of Stellantis)
Combining air and ground
A recent patent shows how autonomous air and ground systems can be combined in a swarm. This pairs a vehicle with a commercial off-the-shelf (COTS) UAV that can autonomously follow the vehicle as it navigates various terrains. The key is linking the vehicle’s infotainment system to the UAV, displaying live video and real-time data to the car.
This allows the UAV to act as an intelligent observer, continuously tracking the vehicle’s movements and relaying data that can be used to monitor the surroundings, assess obstacles or even help with navigation. This ‘follow-me’ functionality opens up new possibilities for capturing offroad adventures from a bird’s-eye view, providing a unique perspective on the driver’s journey.
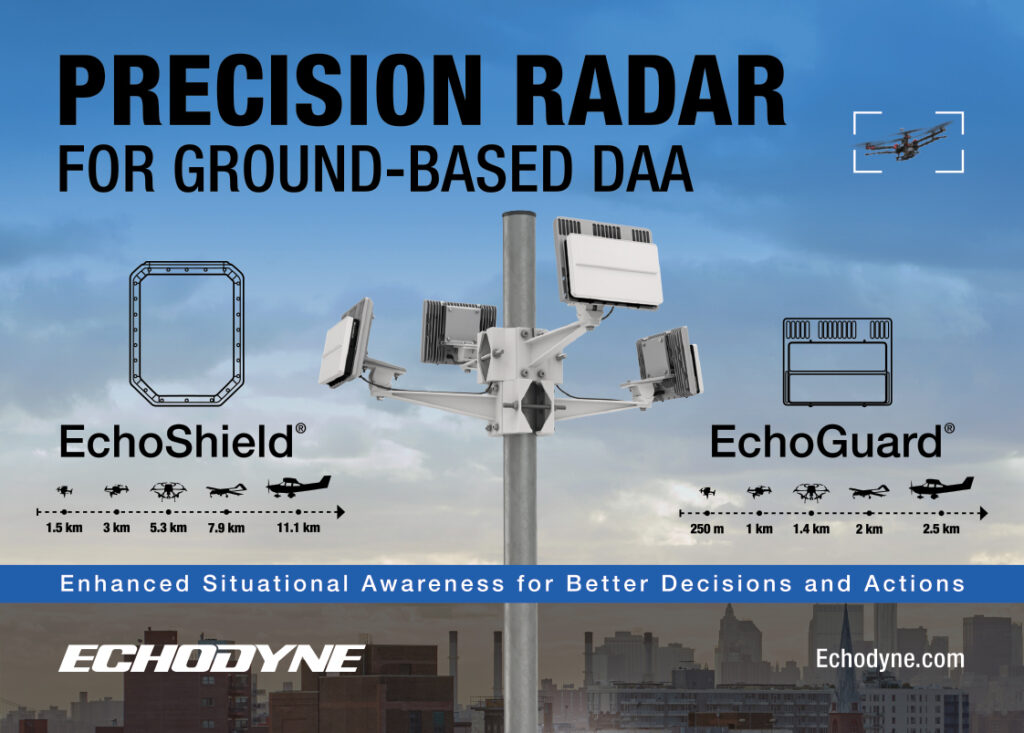
The key to the technology is the way that the UAV and vehicle interact. The UAV uses GPS and a camera to follow the ground vehicle at a fixed distance, adjusting its position dynamically based on the terrain and speed of the vehicle. The data collected by the UAV, such as terrain type, obstacles and weather conditions, is transmitted to the infotainment system.
This can also be used to support more complex offroad scenarios, such as warning of large rocks or sudden drops that might not be visible from the vehicle.
In autonomous mode, the vehicle control system uses data from onboard Lidar, camera and GPS sensors combined with the remote data to control the path and speed. A key factor is that the UAV can be autonomously controlled by the vehicle control system.
When not in use, the UAV is stored and recharged on the vehicle. The base includes a retention mechanism to keep it secure and a power supply to recharge its battery. The technology is proposed for prototypes later this year with a driver, and for fully autonomous offroad driving by 2030.
Maritime
In the oceans, swarms of autonomous underwater vehicles (AUVs) require accurate coordination and positioning to provide detailed surveys of the seabed. To overcome this challenge a sonar system on the surface can link to all of the AUVs in a swarm to provide coordination.
The Autonomous Sparse-Aperture Multibeam Echo Sounder scans at surface-ship rates while providing sufficient resolution to find objects and features in the deep ocean, without the time and expense of deploying underwater vehicles.
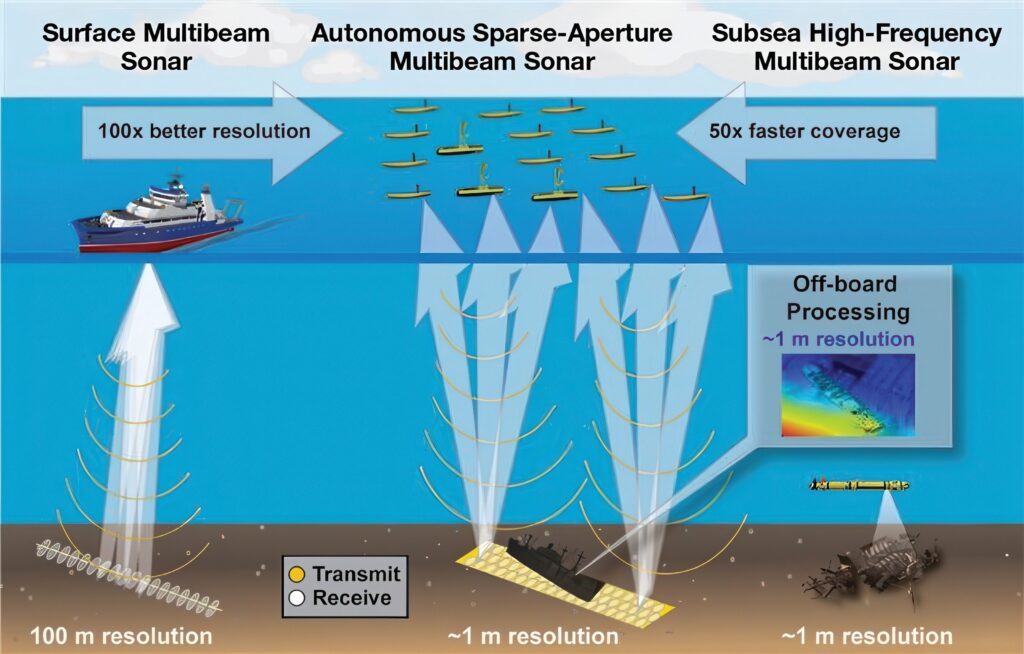
(Image courtesy of MIT Lincoln Lab)
The echo sounder is a large sonar array, using a small set of ASVs that can be deployed via aircraft into the ocean, which can map the seabed at 50 times the coverage rate of an underwater vehicle and 100 times the resolution of a surface vessel. This approach provides the best of both worlds: the high resolution of underwater vehicles and the high coverage rate of surface ships.
Large surface-based sonar systems at low frequency have the potential to determine the materials and profiles of the seabed, but typically do so at the expense of resolution, particularly with increasing ocean depth.
Resolution is restricted because sonar arrays installed on large mapping ships are already using all of the available hull space, thereby capping the sonar beam’s aperture size.
An array of underwater UUVs can operate at higher frequencies within a few hundred metres of the seafloor and generate maps, with each pixel representing 1 m2 or less, resulting in 10,000 times more pixels for that area.
However, this comes with trade-offs: UUVs can be time-consuming and expensive to deploy in the deep ocean, limiting the amount of seafloor that can be mapped; they have a maximum range of about 1,000 m and move slowly to conserve power.
The area coverage rate of UUVs performing high-resolution mapping is about 8 km2 per hour; surface vessels map the deep ocean at more than 50 times that rate.
Instead, a collaborative array of 20 ASVs, each hosting a small sonar array, effectively forms a single sonar array that is 100 times the size of a large sonar array installed on a ship. The large aperture achieved by the array (measuring hundreds of metres) produces a narrow beam, which enables sound to be steered precisely to generate high-resolution maps at low frequency.
However, this collaborative and sparse setup introduces some operational challenges. First, for coherent 3D imaging, the relative position of each ASV’s sonar subarray must be tracked accurately through dynamic, ocean-induced motions.
Second, because sonar elements are not placed directly next to each other without any gaps, the array suffers from a lower signal-to-noise ratio and is less able to reject noise coming from unintended or undesired directions. To mitigate these challenges, a
low-cost, precision-relative navigation system uses acoustic signal-processing tools and ocean-field estimation algorithms. These algos estimate depth-integrated water-column parameters to account for the temperature, dynamic processes such as currents and waves, and acoustic propagation factors such as the speed of sound in the water.
Processing of all of the required calculations can be completed remotely or onboard the ASVs. For example, ASVs deployed from a ship or flying boat can be controlled and guided remotely from land via a satellite link or from a nearby support ship using a satellite link.
With an autonomous support chip, this swarm could map the seabed for weeks or months at a time until maintenance is needed. Sonar-return health checks and coarse seabed mapping would be conducted onboard, while full, high-resolution reconstruction of the seabed requires a supercomputing infrastructure on land.
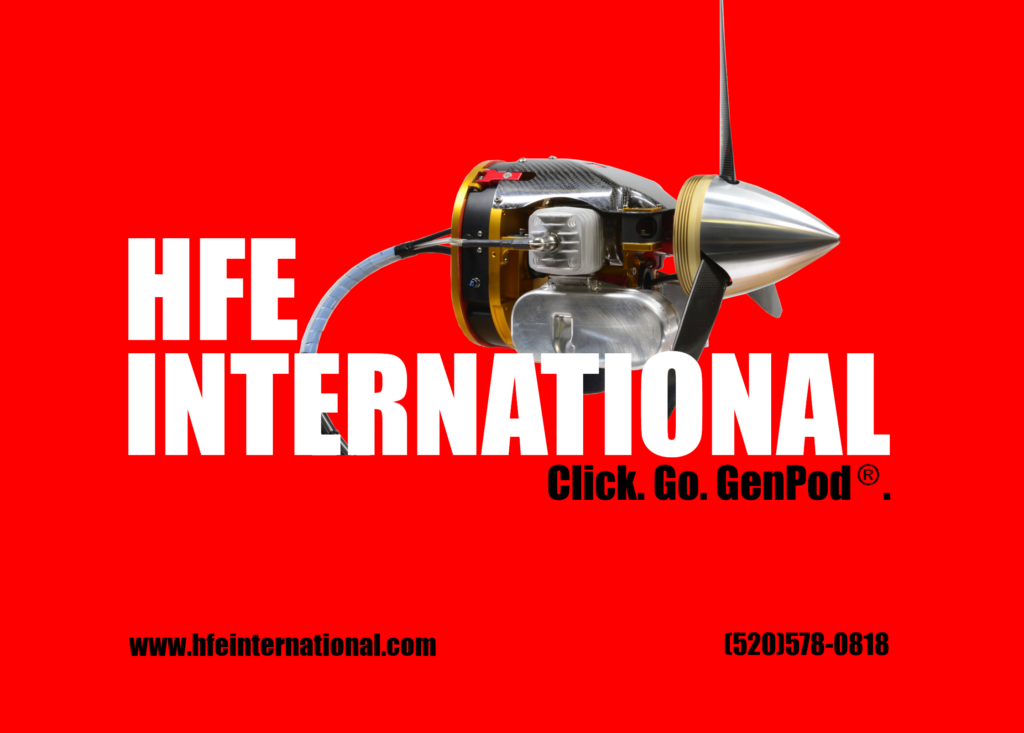
Deploying vehicles in an area and letting them map for extended periods of time without the need for a ship to return home to replenish supplies and rotate crews would simplify logistics and lower operating costs significantly.
An 8 m array, containing multiple subarrays equivalent to 25 ASVs locked together, has been tested, generating 3D reconstructions of the seafloor and a shipwreck.
Space
The first swarm in orbit has been tested using only visual information shared through a wireless network. Robust navigation presents a considerable technological challenge. Current systems rely on the Global Navigation Satellite System (GNSS), requiring frequent contact with terrestrial systems.
The Starling Formation-Flying Optical Experiment, or StarFOX, uses four small CubeSat satellites’ onboard star-tracker cameras to calculate their trajectories, so no extra hardware is needed to implement swarm capabilities.
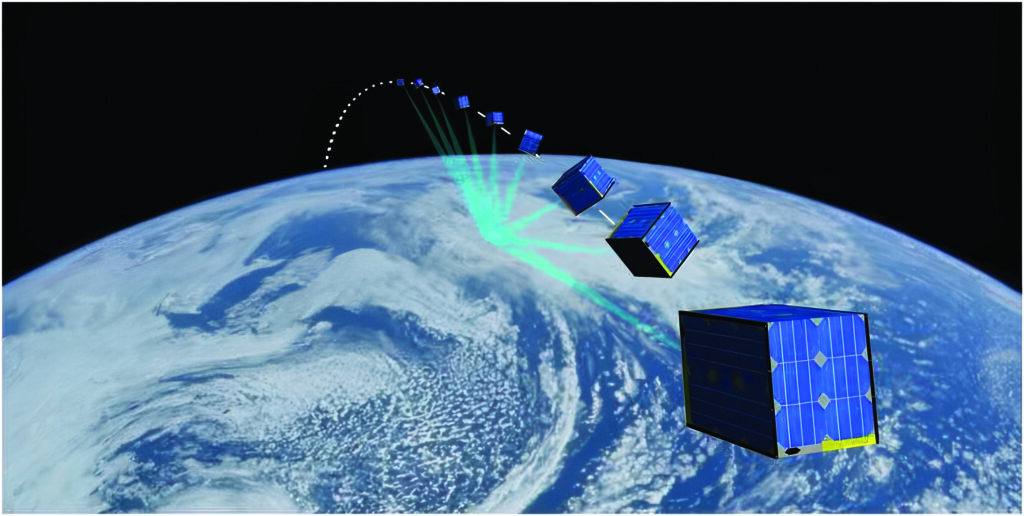
(Image courtesy of Grainger College of Engineering at the University of Illinois Urbana-Champaign)
The field of known stars in the background is used as a reference to extract bearing angles to the swarming satellites. These angles are processed onboard through accurate, physics-based force models to estimate the position and velocity of the satellites with respect to the orbited planet – in this case, Earth, but also the moon, Mars or other planetary objects.
The swarm control system has three elements. An image-processing algorithm detects and tracks multiple targets in images and computes target-bearing angles – the angles at which objects, including space debris, are moving towards or away from each other.
The Batch Orbit Determination algorithm then estimates each satellite’s coarse orbit from these angles. A Sequential Orbit Determination algorithm then refines swarm trajectories with the processing of new images through time to potentially feed autonomous guidance, control and collision avoidance algorithms onboard.
Data is shared over an inter-satellite communications link to calculate robust absolute and relative position and velocity to an accuracy of 1.3% without GNSS using a single satellite. Using data from other satellites in the swarm, this accuracy increases to 0.5%.
AI
Many swarm coordination algorithms are possible with deterministic, rule-based logic, but recent developments in machine learning have enhanced autonomous navigation. AI algorithms help UAVs identify and avoid obstacles, move with autonomy, and make changes in real time based on their environment.
A traditional command-and-control structure has agents organised in a hierarchy and detailed, tactical information is fed up the chain of command. While this hierarchical design simplifies data flow, it is not robust, and it is inflexible when dealing with dynamic scenarios that require rapid reactions from agents.
Centralised control of a swarm requires a hub-and-spoke communication architecture, which presents several disadvantages: it limits the autonomous behaviour of the swarm, it does not enable communication between agents and it allows for a single point of failure in the design.
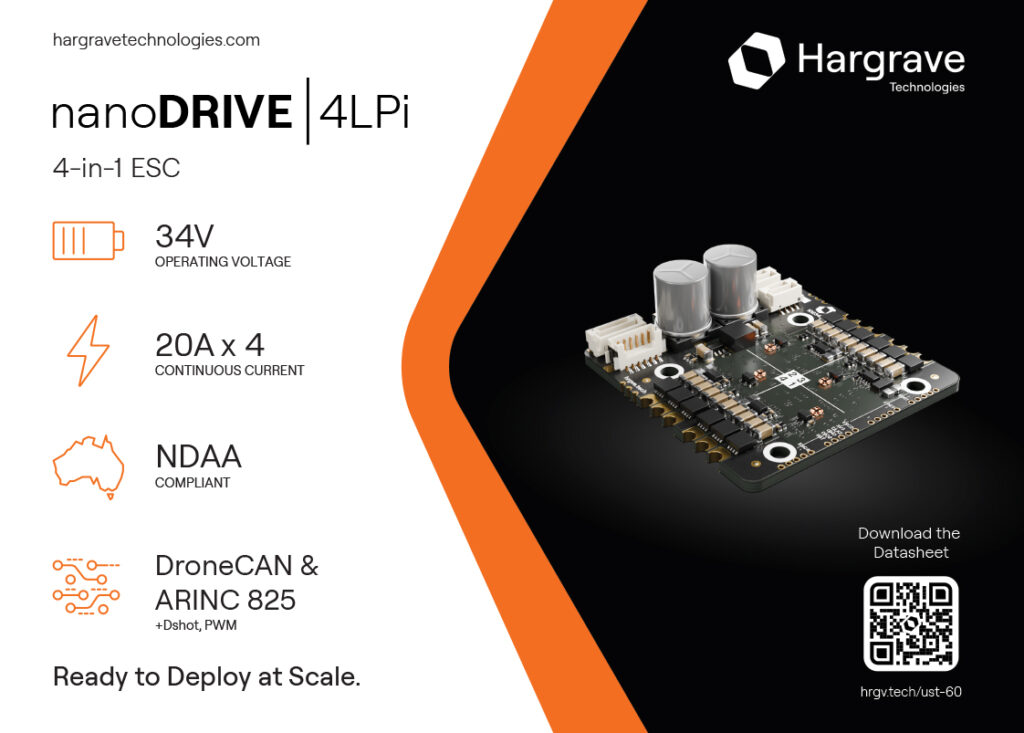
An alternative is a distributed architecture where swarm decisions are made via collective consensus among agents. This type of architecture is robust and scalable, but requires a communication network that will support potentially greater data traffic.
A hybrid of command-and-control architectures can be used to take advantage of the strengths of each one, using a distributed architecture for situational awareness data and an orchestrated architecture for selecting targets.
Finite state machines (FSM) (or finite state automata) have been shown to be effective in modelling multivehicle autonomous, uncrewed system architectures. Within an FSM architecture, each agent operates within one of several defined states at a given time. The trigger events that cause the agent to transition between states are precipitated by environmental conditions it senses or events it encounters.
This type of structure is applicable in developing swarm systems as the states and triggers can be defined deterministically, which is necessary for high-risk mission events. There may be other events, such as searching, where some bounded degree of unpredictability is desired. In those cases, probabilistic FSM can be used by allowing for different behaviour within a state.
AI has been key to the first flight-test swarms of UAVs with different levels of autonomy. Using AI and intelligent agents reduces the cognitive load on operators yet ensures they remain in control, particularly during critical mission phases.
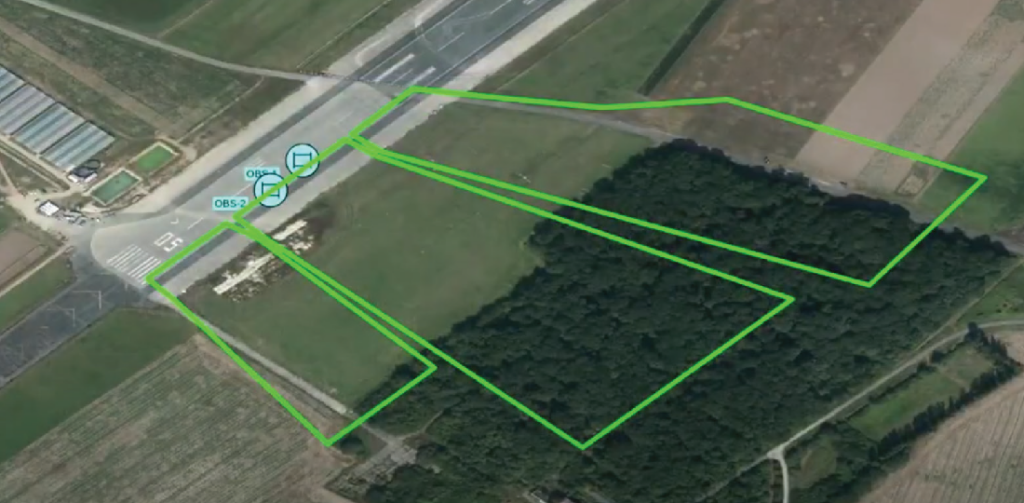
(Image courtesy of Thales)
The control logic for the swarm can use a rule-based system with predictability and determinism, or with an AI framework. If the AI framework is not able to be deterministic, the autopilot has to be able to sidestep the mission-control computer when it has an issue. This requires additional logic that monitors the MCS and on encountering a problem decides whether to go into safe mode to segregate the AI.
Generative AI, such as large language models (LLMs), are used for the human-to-machine interface, giving an operator easier ways to define what they want to do with large numbers of UAVs in a swarm.
Flight tests with nine UAVs connected via 4G cellular have shown that AI can support swarms by allowing operators to adapt the level of autonomy of the vehicles to the operational requirements of each phase of the mission, which also reduces the load on the wireless network.
The UAVs are capable of perceiving and analysing their local environment, sharing target information, analysing enemy intent and prioritising missions. They can also use collaborative tactics and optimise their trajectories to increase resilience and boost force effectiveness.
These use specialised machine-learning frameworks developed specifically for swarm applications. These are optimised for analysis of the data generated by sensors and decision-support pathways for mixed UAV swarms, while addressing the specific cybersecurity, embeddability and system optimisations for smaller aircraft with constrained resources.
All this is driving development of swarm operation in the air, on land and at sea to implement more resilient operations with different types of uncrewed systems.
Acknowledgements
With thanks to Iker Camiruaga and Ignacio Calomarde at UAV Navigation, Andrew March at MIT and Nils Thorjussen and Tony Samaritano at Verge Aero.
Some examples of swarm suppliers
FRANCE
Thales | +1 646 664 0659 | www.thales.com |
SPAIN
UAV Navigation | +34 91 657 2723 | www.uavnavigation.com |
UK
Hadean | +44 203 514 1170 | www.hadean.com |
Saab/Blue Bear Systems Research |
+44 1234 826 620 | www.saab.com |
USA
Applied Aeronautics | +1 512 961 1771 | www.appliedaeronautics.com |
Honeywell | +1 480 353 3020 | www.honeywell.com |
Saronic Technologies | – | www.saronic.com |
Swarm Aero | – | www.swarmaero.com |
Verge Aero | +1 425 556 2900 | www.vergeaero.com |
UPCOMING EVENTS
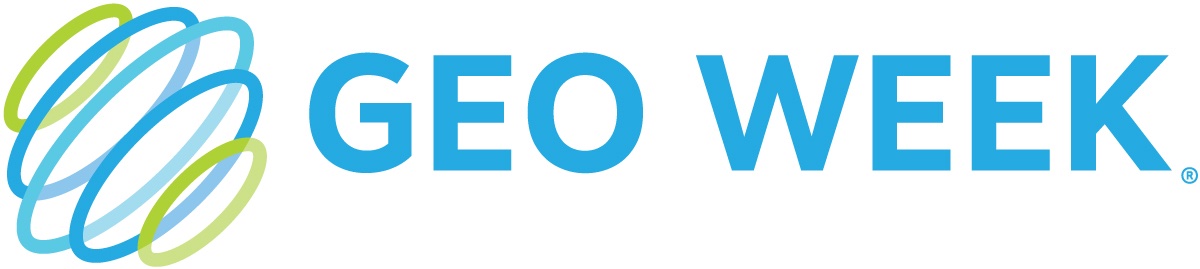
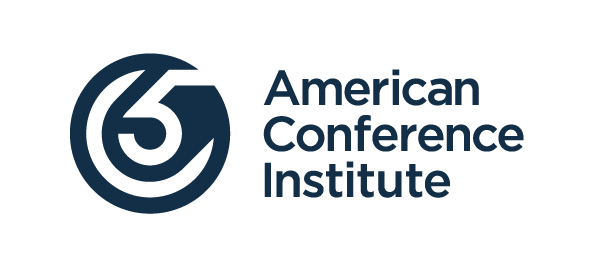
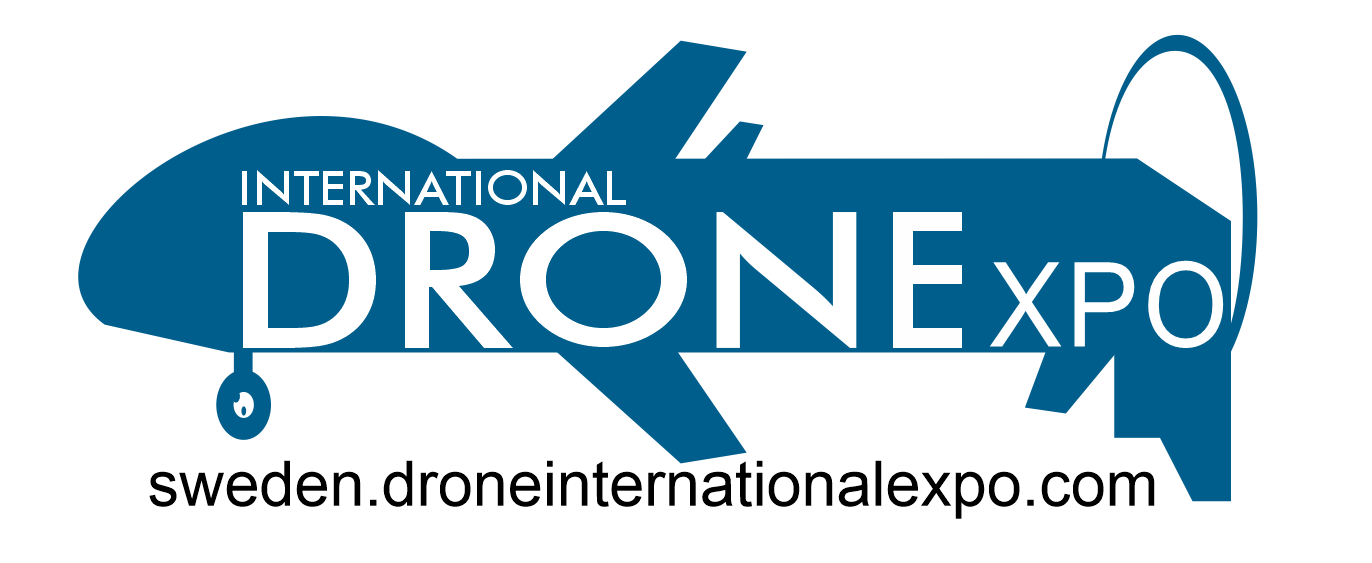
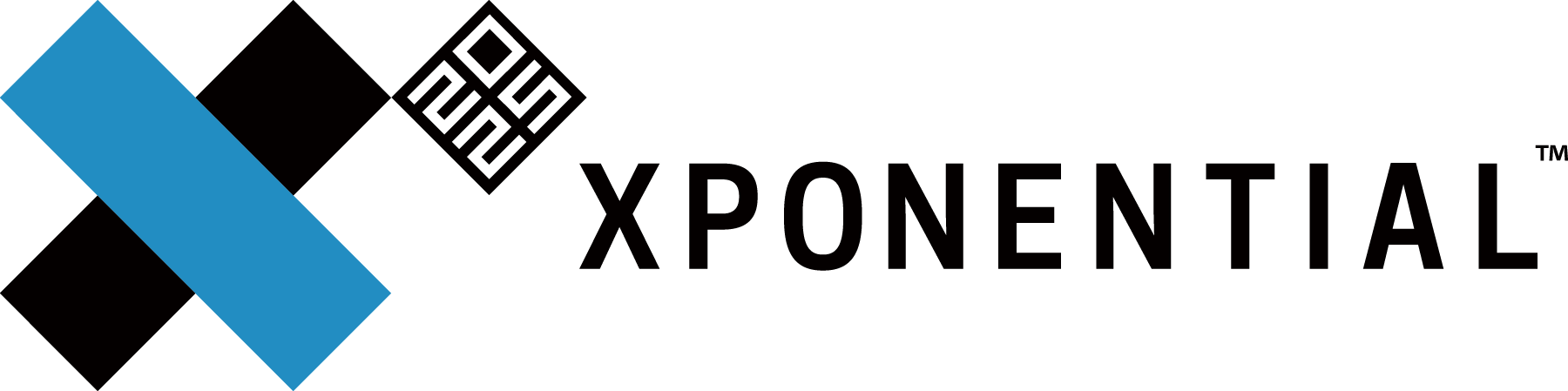
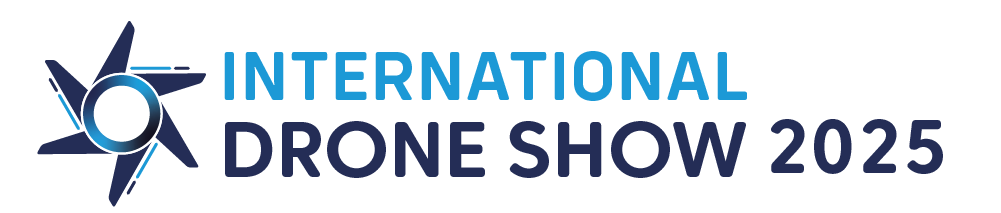