Radio and telemetry
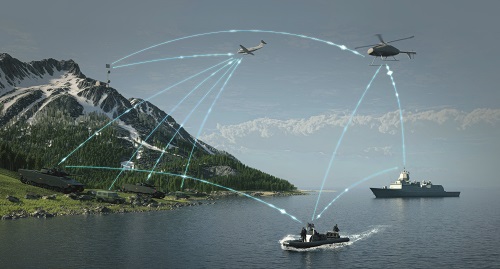
(Image courtesy of Radionor)
New radio tech promises to take uncrewed systems further on less energy, Nick Flaherty reports
Radio connections for uncrewed systems are going through significant changes. Cognitive radio (CR) is increasingly being adopted, using software-defined radio (SDR) to analyse the local spectrum and find areas that are clear, reducing the power required and extending battery life.
Mobile ad-hoc networks (MANETs) are being used with swarms of UAVs to enhance radio connectivity, sharing data about the spectrum across a wide area.
New, lightweight antenna designs with multiple feeds and beamforming are also improving the performance of radio links in the air, allowing for longer range connections beyond the visual line-of-sight (BVLoS) or reducing the power needed for close work.
Meanwhile, machine learning (ML) and artificial intelligence (AI) are being adopted in radio systems to boost cognitive architectures and the efficiency of connections, especially for high-bandwidth video.
At the same time, millimetre-wave radio technology (mmWave) is maturing to provide higher-bandwidth video links that are less susceptible to interference. The technology is maturing to be cost effective for vehicles on the ground and for high-altitude pseudo satellites (HAPS), where power is a critical consideration.
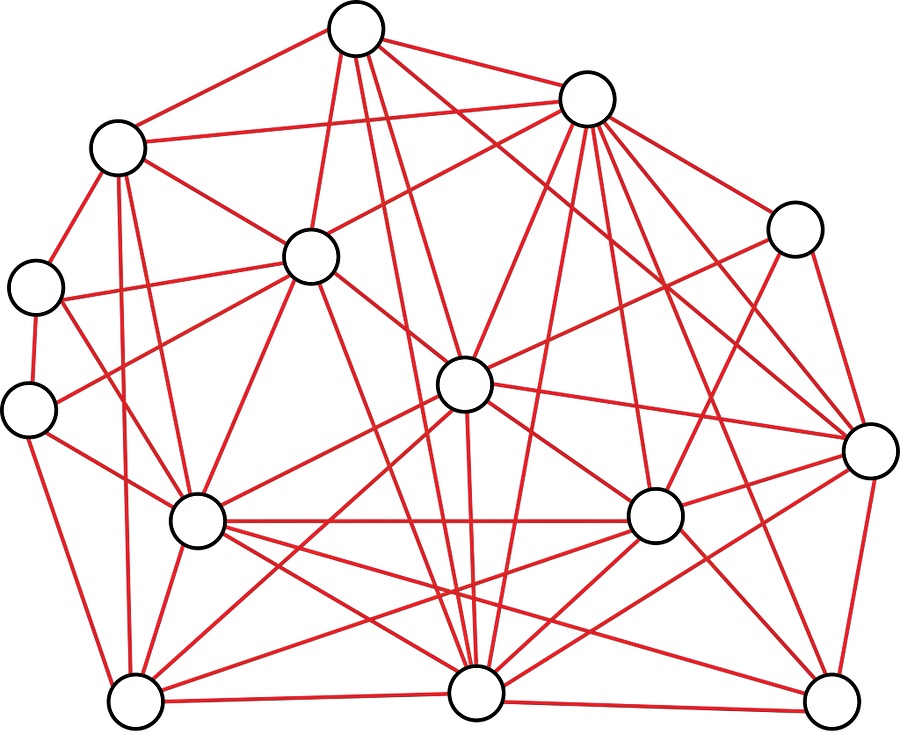
MANET
A mobile ad-hoc network (MANET) is a data network suitable for voice, data and video traffic. It is ad-hoc because it is not reliant on any pre-existing infrastructure. Data traverses the system by ‘hopping’ from one network node to another until it reaches its destination.
Instead of using a centralised router, each node participates in routing traffic by forwarding data for other nodes. The determination of which node forwards the data is a dynamic decision made by an algorithm based on a number of variables, which include the availability of nodes in the network – which is often the number of UAVs in a swarm – the status of the radio link and any environmental interference, as well as the signal-to-noise ratio (SNR).
Many MANETs are self-forming and self-healing. If any nodes in the MANET become unavailable for any reason, the algorithm running on the remaining nodes will reroute traffic seamlessly via the most efficient path.
A MANET differs from the more static mesh network, which is more reliant on a fixed infrastructure. Typically, a mesh network has one node designated as the ‘Master Node’ to maintain the entire system. This Master Node distributes the network addresses to all other nodes in the network and monitors the flow of traffic to decide on the most efficient route.
The whole mesh network is dependent on this Master Node. This creates a single point of failure, however, and restricts the flexibility of the system in the air if one UAV has to act as the master.
In contrast, a MANET is entirely dynamic and uses an adaptive routing approach. There is no Master Node to manage the network. All of the nodes in a MANET collaborate collectively to route traffic and maintain a robust link, making it more resilient than a mesh network and less prone to failure.
MANETs have been developed that can operate on 3 x 3 multiple-input multiple-output (MIMO) antennas for high-performance data rates for aerial systems, delivering over 120 Mbps. The MIMO antennas allow three different signals to be received at the same time, with algorithms to cancel out any differences in timing or reflections of radio waves that can reduce the efficiency of a link.
However, the development of lightweight MIMO antennas for UAVs and HAPS platforms is a key challenge, and this is discussed below.
A MANET typically operates at the OSI Layer 2 level, the same layer as a network switch, with the ability for each node, or UAV, to optimally configure its transmission parameters.
This provides the user with a ‘transparent network’. The IP address scheme of the MANET doesn’t have to be the same as that of the devices connected to it, providing an almost plug-and-play network. This simplifies the process of adding UAVs to a swarm in the air, supporting multicast traffic where data is sent to all the nodes in the network.
There is no limit to the number of nodes in a MANET. During real-world testing of a MANET radio in 2018, a flat network of 320 MANET nodes was successfully evaluated, with plenty of overhead for further expansion. However, the practical size of the network depends on the data being transferred and the range.
MANET radio transceivers have been designed into a number of current UAV systems. These devices replace separate, specialised equipment and reduce size, weight, power consumption and cost, enabling increased flight time and releasing valuable payload to embed other sensors. Each system installed with an embedded module extends the MANET’s capability, allowing users to access services such as video or data on any node.
One embedded module uses a built-in Android system that enables the direct installation of applications on to the radio, turning a single radio into a pilot system that is able to fly or drive multiple uncrewed systems. This allows a group of UAVs to operate, communicate and swarm on a common network.
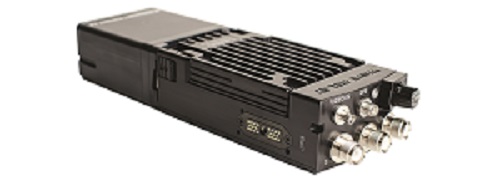
(Image courtesy of Steatite Communications)
MIMO
Another technique employed in MIMO technology is space-time block coding (STBC).
Space-time block coding, which works in conjunction with spatial multiplexing, is a MIMO technique used to transmit multiple copies of the same data across the three antennas and exploit the various received versions to improve the reliability of data transfer.
Every time a radio frequency (RF) signal encounters a building, wall or other obstacle, it basically inverts and fades. These constant reflections and refractions of the RF signal are a form of interference, and each reflection reduces its overall clarity.
The redundancy of a MIMO antenna system results in a higher chance of being able to use one or more of the received copies to correctly decode the received signal.
Space-time coding combines all copies of the received signal in an optimal way to extract as much information from each of them as possible. This allows system developers to trade off the range of the links, the data rate and power consumption, depending on the mission profile.
Antenna diversity
The ability to make use of multiple antennas in a MIMO system, each one at a slight angle, provides increased performance and resilience – this is commonly referred to as antenna diversity.
Antenna diversity benefits a datalink by giving the antenna a different ‘viewpoint’ to its peers. While antenna 1 might be susceptible to interference because of its angle (polarisation), antennas 2 and 3 might not be as influenced by interference because of their slightly different polarisation.
This is key for UAV radio designs as there are many sources of environmental RF interference operating in the same frequency space as the transmitter and receiver, over which the operator has no control.
This interference is inevitable and not something that can be prevented easily. The RF spectrum in a particular airspace is rarely exclusive and has to be shared with other users, such as mobile phone network operators, TV broadcast operators and wi-fi hotspots.
This interference is likely to vary in different scenarios. In some environments, vertically polarised antenna may be more susceptible to interference than horizontally polarised ones because, for example, there may be lots of other vertically polarised transmitters and receivers within the local vicinity.
In this case, a MANET radio can be fitted with two vertically polarized antennas, with a central, horizontally polarized antenna. Spatial multiplexing and space-time block coding means the same data is sent three times (once per antenna) and re-assembled at the receiving end of the datalink.
If there is interference on the horizontally polarised antenna, data sent via the vertically polarised antenna will still have a very good chance of being received, even if the UAV is moving around. In field tests, having an antenna combination such as this has proven to almost double the bandwidth of the datalink.
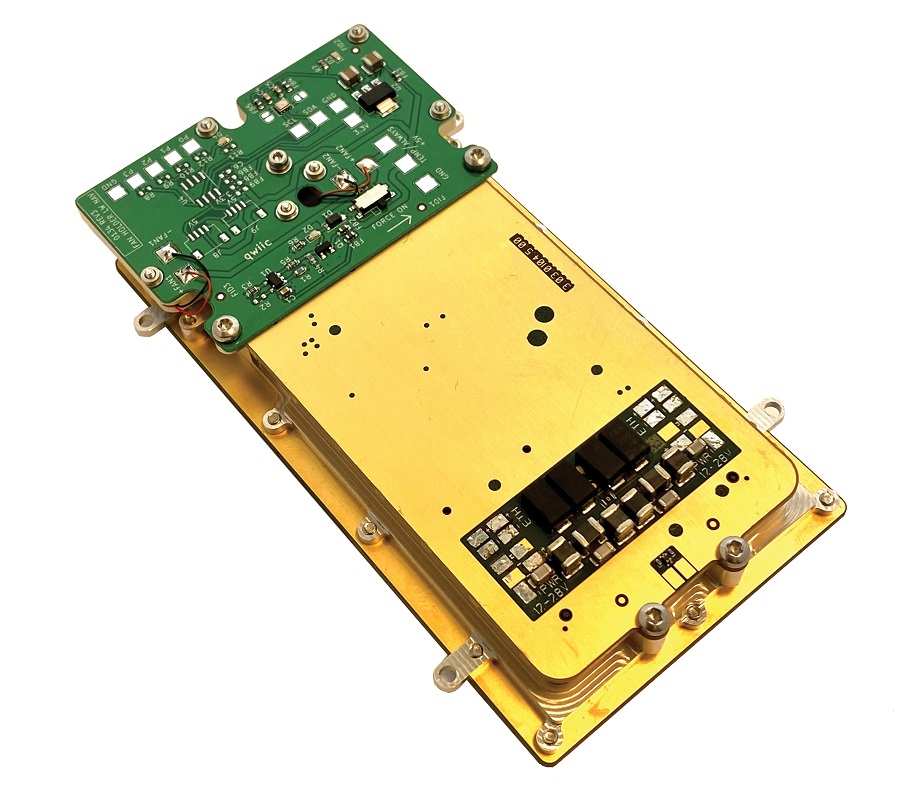
(Image courtesy of Radionor)
Beam steering
An alternative approach is to use a tracking antenna with beam steering. This technique uses multiple antenna elements to direct a signal to a specific fast-moving UAV in the air, and even swap from one UAV to another instantaneously. This boosts the range between two nodes when using a tracking antenna to over 400 km.
A conventional, motorised tracking antenna system has a narrow antenna beam that is steered in the direction of interest, where it focuses energy. This increases operational range, but because it is locked with a motorised system, it can only operate as a point-to-point link.
The motorised tracking antenna also has a limited rotation speed, and this problem is enhanced when tracking a fast-moving platform such as a UAV or USV on water.
In contrast, an electronically steerable radio system can change the direction of the antenna at high speed, enabling a single ground antenna to operate several UAVs simultaneously. This point-to-multipoint functionality provides connectivity to every unit in a network, even if all the units are moving.
The digitally steerable beam constantly optimises the direction of transmission and reception several thousand times per second. The electronic steering involves no moving parts and enables the ultra-narrow beam to be steered instantly in the right direction to communicate with several fast-moving objects.
This enables an ultra-long-range tactical data link with point-to-multipoint ability, together with high broadband capability, even when tracking multiple fast-moving platforms, such as UAVs in a swarm or USV on the water.
The number of transceivers defines the antenna gain for the range and the narrowness of the beam. A tighter beam has more signal margin and therefore provides a higher data rate.
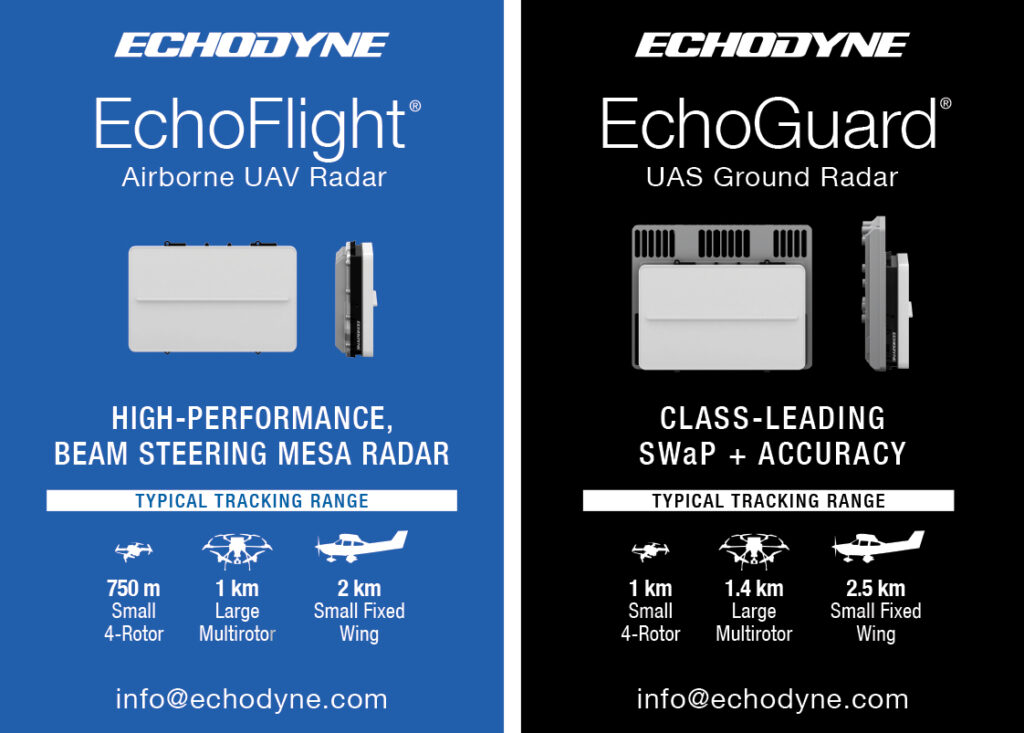
Spiral antennas
Customised spiral antennas are used in a wide range of demanding environments, providing almost frequency-independent performance over wide bandwidths with broad beam widths.
The purity of circular polarisation creates outstanding left-hand circularly polarised or right-hand circularly polarised antennas. High-volume spiral antennas have been developed with two-arm spiral versions, while custom-made, four-arm spiral versions provide higher sensitivity.
The spirals have a compact, lightweight design, formed by placing the spiral on top of a cylindrical absorber-filled cavity, and they can be provided with a protective, lensed radome covering if required. They can be used as single elements, in arrays or as feeds in reflectors.
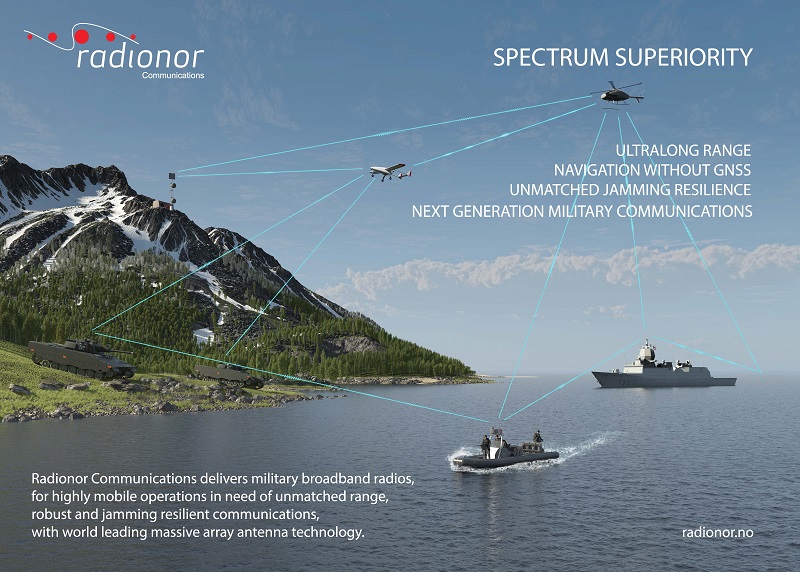
AI for radio
Another new field of ML is federated learning (FL), which was proposed by Google in 2016 and is designed to support network systems with decentralised data. It aims to train a highly centralised model on devices sharing decentralised data without the need for this data to be sent to a local shared unit, essentially running ML algorithms with decentralised data architecture.
This is of particular interest for UAV swarms and their radio connections through a combination of mobile edge computing (MEC) and non-orthogonal multiple access (NOMA). In theseUAV-NOMA-MEC systems, UAVs are equipped with computing capabilities, and they can be swiftly deployed for situations where terrestrial MEC servers are overloaded or unavailable.
Operational control of UAVs often relies on wireless communication, which introduces difficult challenges for spectrum allocation and interference cancellation.
Developments such as fog computing use these techniques to achieve higher capacity than conventional communication networks by jointly optimising user association, power control, the allocation of computing resources and location planning.
In AI algorithms, the network parameters need to be shared with other intelligent agents or network models. For AI-enabled UAV-NOMA-MEC, the transmission of network parameters in AI algorithms and wireless signals need to be jointly optimised, so this is leading to the development of a unified design for AI and wireless transmission systems.
AI is also used to improve the control of a swarm of UAVs to optimise the radio links.
The proposed solution supports the swarm of UAVs during their flight by maintaining their topology in an energy efficient manner. This uses the principles of a mathematical model called the Reynolds’ Boids model, which looks at the alignment, separation and cohesion of the swarm.
The effectiveness of this approach has been successfully tested in simulation with AI frameworks to improve reliability, low latency, handover and path planning.
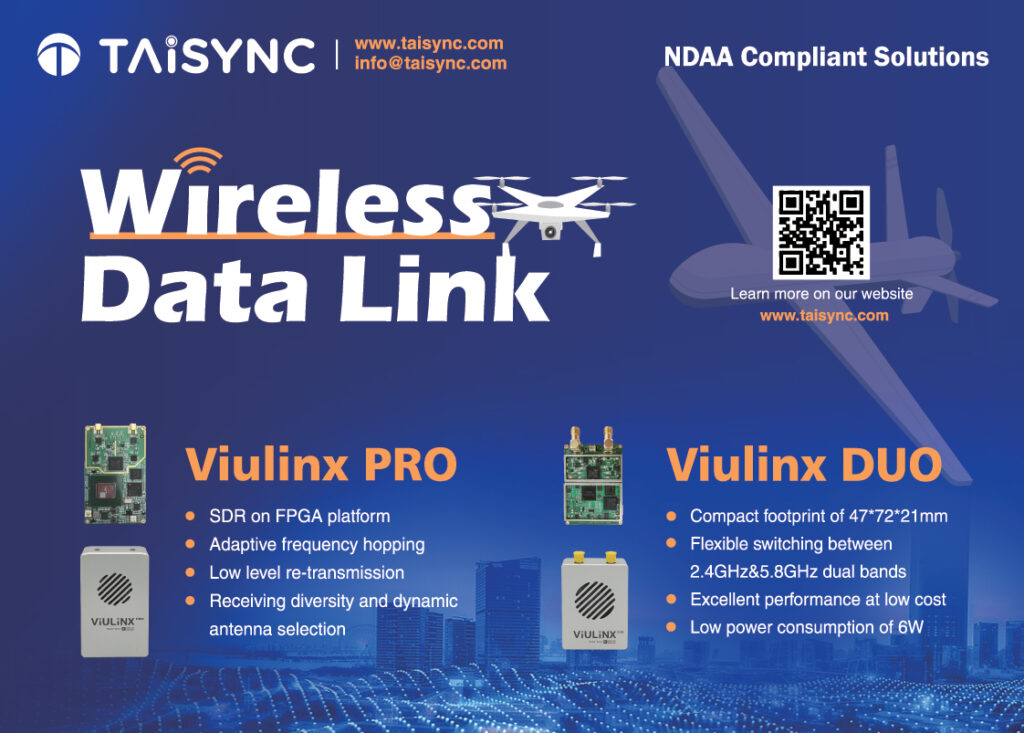
Cognitive radio
The European Telecommunications Standards Institute (ETSI) defines CR as a radio that can sense and understand the radio environment and policies, and monitor usage patterns and users’ needs; autonomously and dynamically adapting according to the radio environment, so that it can achieve predefined objectives, such as the efficient utilisation of spectrum; learning from the environment and results of its own actions, so it can improve its performance.
Essentially, it is an intelligent SDR, using spectrum sensing to identify the available spectrum and detect sources when operating in a licensed band. Spectrum management elects the best available channel, coordinates accessibility to the available channel with other users, and vacates the channel when a primary user arrives.
Although CR devices might represent a computational overload for UAVs, they could be capable of reducing energy consumption. Because UAVs operate in overcrowded spectrum bands, they are susceptible to a high number of lost data packets, increasing the need for packets to be retransmitted using more energy.
As a result, the energy consumed for packet retransmission may be greatly reduced when a UAV is equipped with a CR.
However, a CR requires joint optimisation with medium access control (MAC) and physical layers. For example, a UAV with a CR user senses different channels simultaneously and uses some idle ones for data transmission. The more channels the CR device can use, the more efficient its bits-per-joule ratio throughput. The bits-per-second ratio also increases with the number of channels used.
This has led to the development of the UAV-assisted cognitive radio (CR) network as a promising technique to address spectrum congestion issues in swarms. However, its performance can be severely affected by the blocked LoS channel in its air-to-ground (A2G) links.
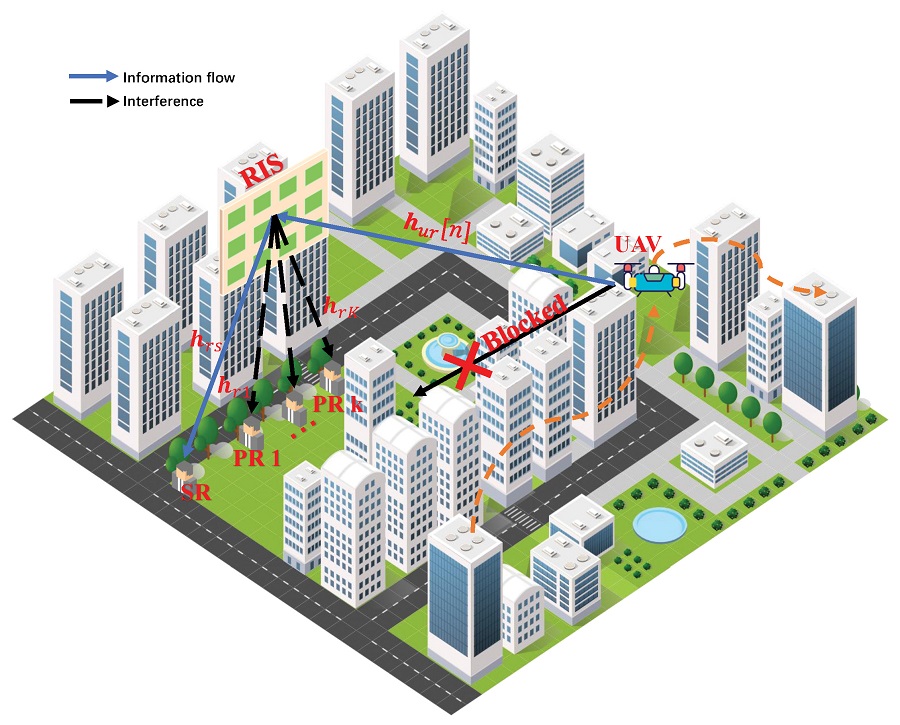
(Image courtesy of Zhejiang Sci-Tech University)
Reconfigurable intelligent surfaces
Using reconfigurable intelligent surfaces (RIS) can help to reconstruct reliable links in UAV-assisted CR networks.
As the name implies, the RIS is a surface that can be changed to reflect radio waves in different ways, and it is usually passive. It is often built from a metamaterial with tiny structures that redirect radio waves in a similar way to a beam-steering antenna by changing the phase relation of the signal.
This allows signals to be directed around buildings or other blocks, reconstructing suitable wireless propagation channel links that a CR can identify and tap into by adjusting its phase shifts to boost performance.
A RIS-based resource allocation method significantly improves energy efficiency by reducing losses. The RIS can reconfigure the wireless channel link between a transmitter and a receiver through controllable intelligent signal reflection, creating a virtual LoS link to bypass any obstacles between the transceivers, which helps to improve the wireless link performance.
The RIS can be deployed in UAV-assisted CR networks once the A2G LoS links have been blocked, and it allows mobile CR users to establish temporary or emergency communications via the cascaded channel links reconstructed by the RIS.
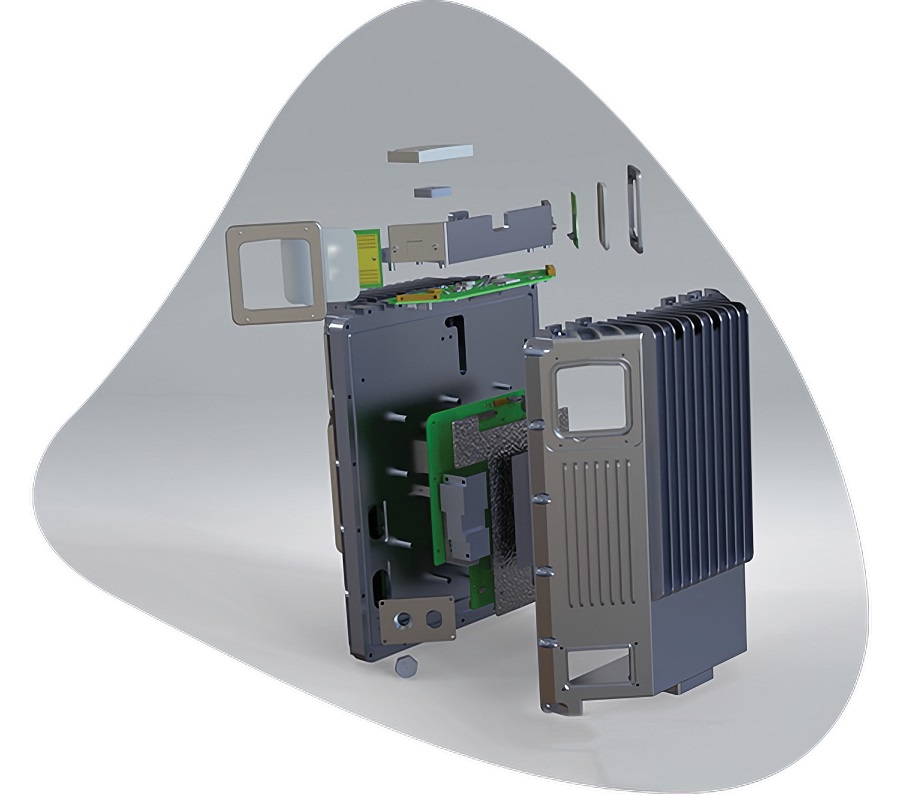
(Image courtesy of Blue Wireless Technologies)
Cooperative spectrum sensing
Building on CR, cooperative spectrum sensing (CSS) involves multiple users reporting the channel data to a fusion centre to improve the network links. The high computing overheads of this approach use a lot of power, however, limiting its use in UAVs.
Instead, a virtual CSS uses a single UAV to conduct CSS while following a circular flight trajectory in the air and acting as the fusion centre. This uses sensing and data-transmission periods that are further divided into mini-sensing slots.
In the virtual CSS, UAV performs local sensing decisions in each mini-slot and accumulates them for a final decision.
The proposed virtual CSS scheme exploits sequential decision fusion (SDF), which sequentially adds individual mini-slot decisions coupled with ML to inspect flight conditions and reconfigure mini-slot periods dynamically. The technique adapts to varying levels of UAV flight velocity, moving radius, detection-probability demand and channel SNR.
There are also opportunities to optimise network performance on the ground. For UAVs using 5G cellular networks, a new AI model could reduce the network resources required by 76%, compared with Open Radio Access Network (O-RAN) systems, and use less energy.
This technique mathematically models the network, using AI to find the best way of allocating computing power across it with only a small additional computational cost. The model could be adapted for other scenarios, including helping UAVs to conserve battery life.
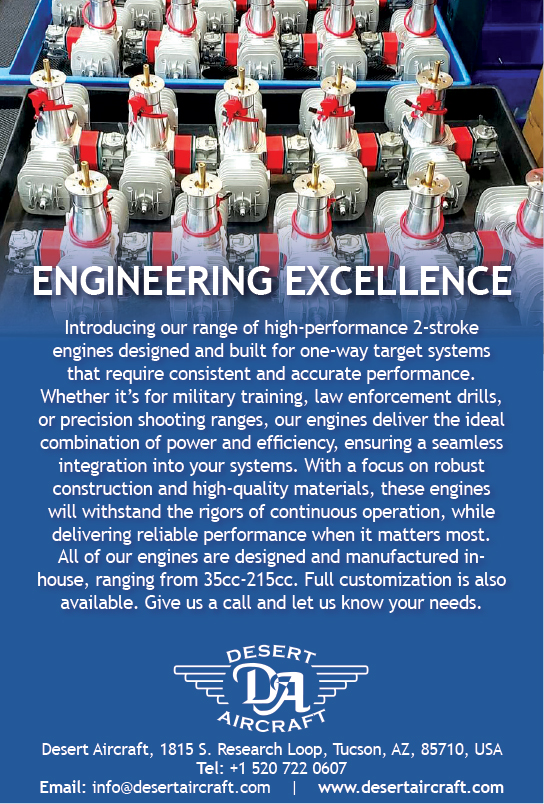
Handling interference
Interference management is a key consideration of any wireless communications system, and with UAVs, it also plays a big role in determining system performance.
A deep reinforcement approach has been developed to address interference, whereby each UAV in a swarm will act as a player and decide on its own path while minimising the latency and interference for the ground network.
The UAVs are used to assist in the integrated access and backhaul (IAB) cellular in-band networks, and to reduce interference at various levels of networks.
When it comes to interference management, power control is another feature that cannot be ignored. A machine-learning approach can manage overall network interference by controlling the UAV’s power and location.
Ground vehicles
For uncrewed ground vehicles used by the military, mmWave is gaining acceptance as the frequencies at 76 GHz are hard to locate and intercept. The latest systems use four radios and two modems for MIMO.
Correcting for the Doppler effect in moving vehicles is vital and the digital signal processing in the modem needs to take this into account. This is even more complex when dealing with multiple signals through the MIMO system with slightly different phases.
Initial rollouts for mmWave military systems use a mesh network with stationary receivers, but the next development will be a peer-to-peer mobile network where the ground vehicles connect to each other with AES256 encryption.
This requires correction for the Doppler effect in all of the different vehicles, as well as a flat Level 2 self-organising network with beam steering to link them together, which is a higher computational load. Current systems can connect vehicles across distances of 5 km with data rates of 100 Mbps using a link budget of over 12 dB. While more power is available for ground vehicles than aerial systems, the four-radio mmWave system has a relatively low power consumption of 30 W.
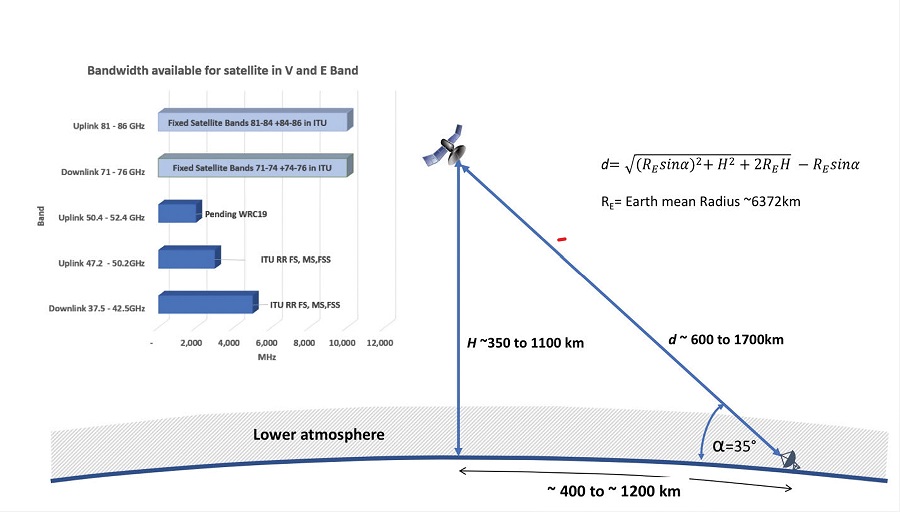
HAPS
This is also attractive for HAPS systems that will adopt mmWave in the next two to three years, which will also require beam-steering and MANET technologies.
The unlicensed 57-71 GHz band is available in North America and 59-63 GHz is available globally, with the upper bands above 64 GHz less susceptible to absorption by oxygen. Either band can be used to connect HAPS aircraft as the absorption issues are a lot lower at an altitude of 70,000 ft, enabling links of up to 100 Gbps.
The 2019 World Administrative Radio Conference (WARC) agreed on the 38-39.5 GHz mmWave band, which can also be used for HAPS systems, providing a bandwidth of 1-2 Gbps.
Rain attenuation is one of the key factors to consider. For a HAPS UAV at 20 km, the footprint can reach 70-80 km in diameter, and the link length will typically be about 40 km at an elevation of 30°. For most of North America and Europe (Zone K), where rainfall is around 42 mm/hour, attenuation at 16-17 dB/km in the mmWave band is significantly higher than for the currently used bands below 50 GHz.
As antenna sizes are smaller at higher frequencies, the signal margins at 48 GHz and 86 GHz are similar for the same antenna size and channel bandwidth, with the data rate of over 10 Gbps available at the E-band using 256 QAM modulation.
This is substantially higher than for the lower bands, due to the greater available channel bandwidth. Even if forced to drop back to older QPSK modulation to improve the signal margin, a 2.5 Gbps data rate can still be achieved.
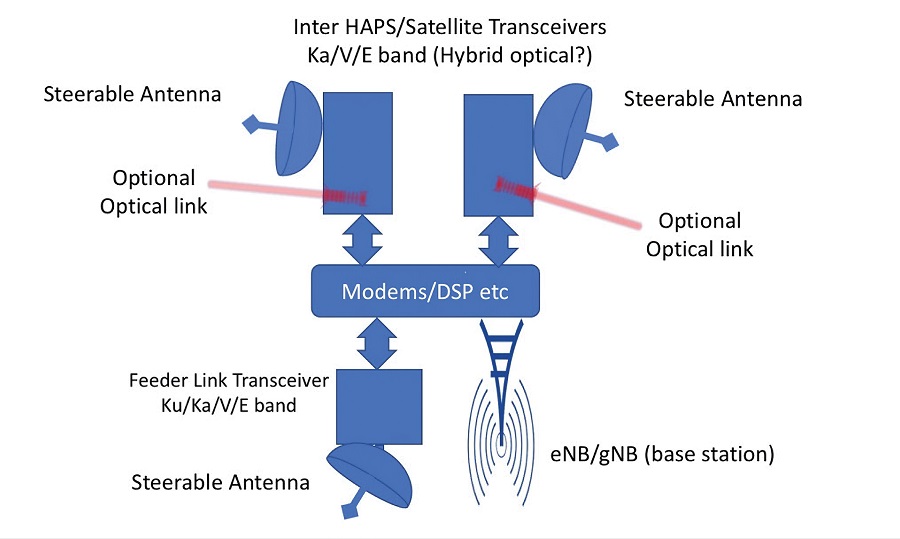
Conclusion
A range of radio technologies are boosting the performance of uncrewed systems, both in the air and on the ground. Electronic beam steering can link multiple platforms in the air, or on the ground with mmWave, and the computing power is enabling more complex algorithms.
At the same time, cognitive radio is being combined with federated machine learning algorithms and reconfigurable intelligent surfaces to provide more information on the radio environment and optimise bandwidth requirements, redirecting beams, extending battery life and boosting mission times.
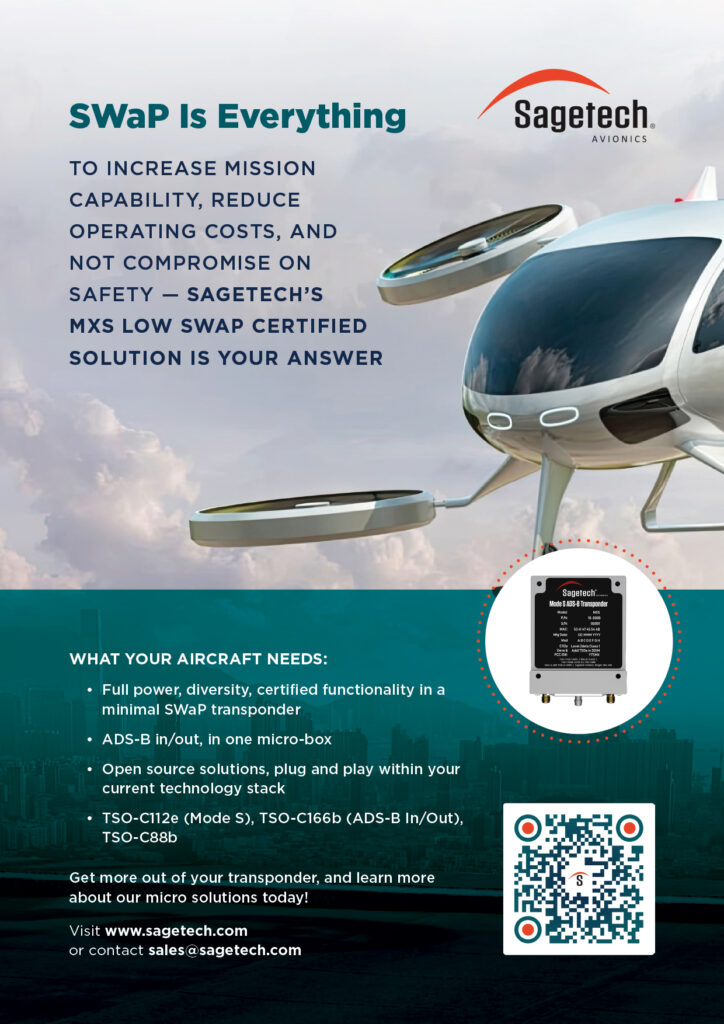
Acknowledgements
With thanks to Atle Sægrov at Radionor, Mark Barrett at Blu Wireless Technologies and Mike Geen at Filtronic.
Examples of radio and telemetry suppliers
AUSTRALIA
Cohda Wireless | +61 8 7099 5500 | www.cohdawireless.com |
CANADA
Peraso Technology | +1 (416) 637 1048 | www.perasotech.com |
Siyata Mobile | +1 757 278 5000 | www.siyatamobile.com |
CHINA
Quectel Wireless | +86 21 5108 6236 | www.quectel.com |
ISRAEL
Autotalks/Qualcomm | +972 9 886 5300 | www.auto-talks.com |
NETHERLANDS
Ampleon | +31 24 355 2666 | www.ampleon.com |
NORWAY
Radionor | +47 72 81 05 00 | www.radionor.no |
Sivers IMA | +46 8 703 6800 | www.siversima |
TAIWAN
Unex Technology | +886 3 657 8188 | www.unex.com.tw |
UK
Blu Wireless Technology | – | www.bluwireless.com |
EnSilica | – | www.ensilica.com |
Filtronic | +44 1740 618 800 | www.filtronic.com |
Steatite Communications | +44 1527 512 400 | www.steatite-communications.co.uk |
USA
Airlinx Communications | +1 888 224 6814 | www.airlinx.com |
Analog Devices | +1 781 329 4700 | www.analog.com |
Commsignia | +1 650 843 9186 | www.commsignia.com |
Harris | – | www.harris.com |
Honeywell | – | www.honeywell.com |
Movandi | +1 949 922 1707 | www.movandi.com |
Silvus Technologies | +1 310 479 3333 | www.silvustechnologies.com |
Texas Instruments | – | www.ti.com |
TrellisWare | +1 858 753 1600 | www.trellisware.com |
Triad RF Systems | +1 855 558-1001 | www.triadrf.com |
Qualcomm | – | www.qualcomm.com |
UPCOMING EVENTS
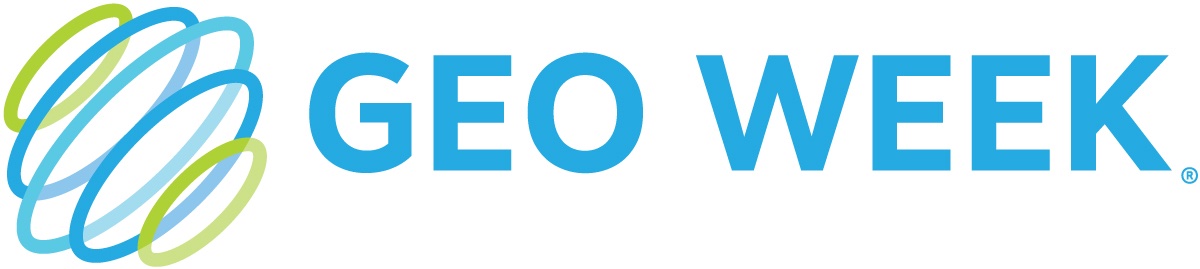
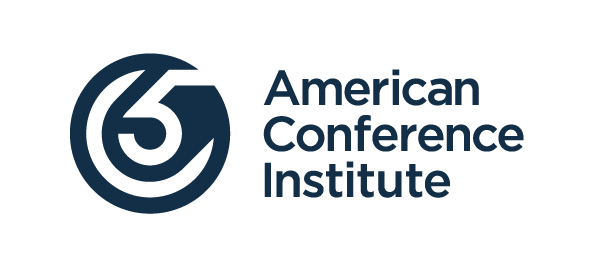
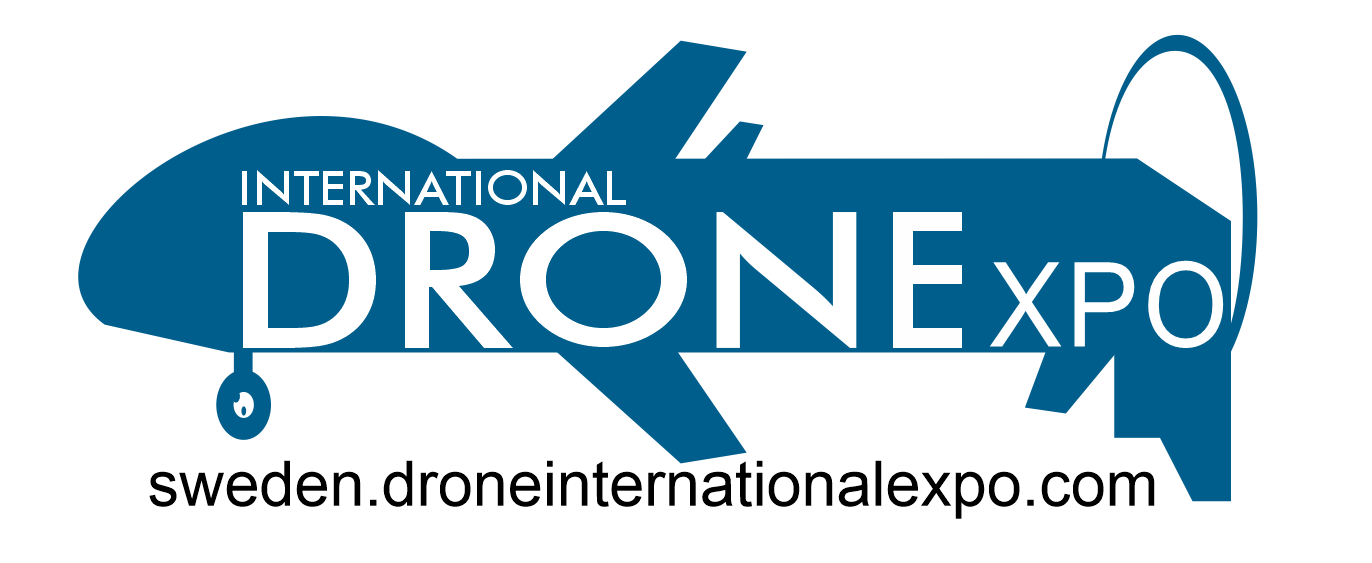
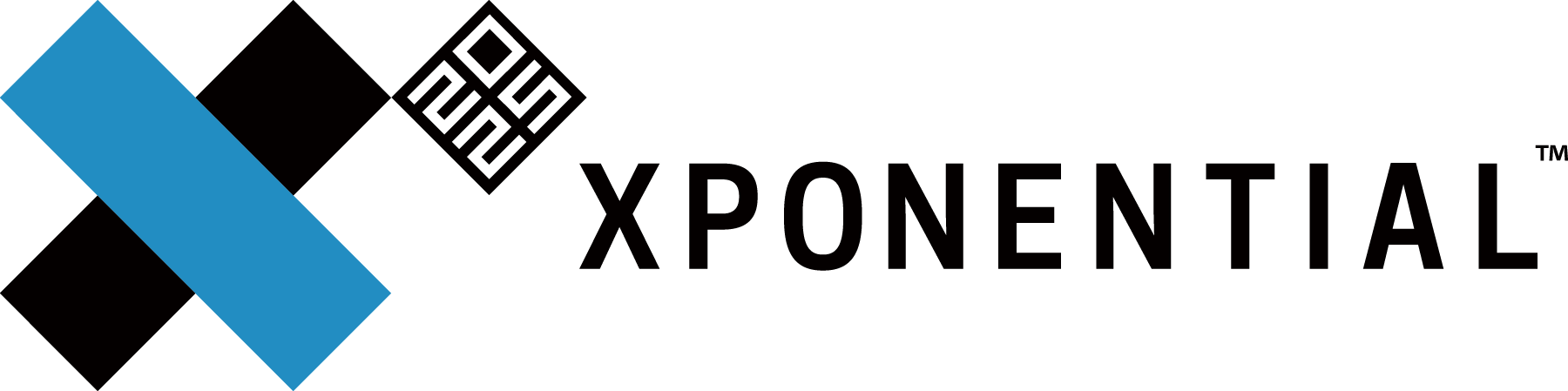
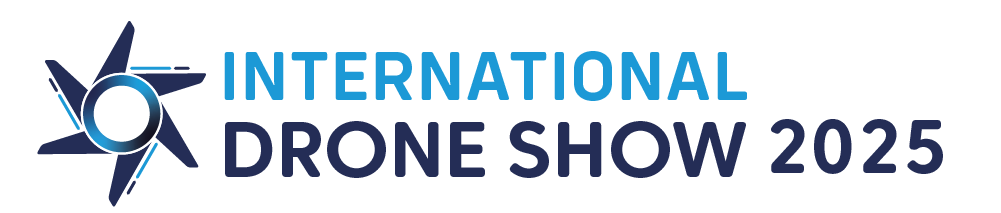