LOXO Alpha & Digital Driver
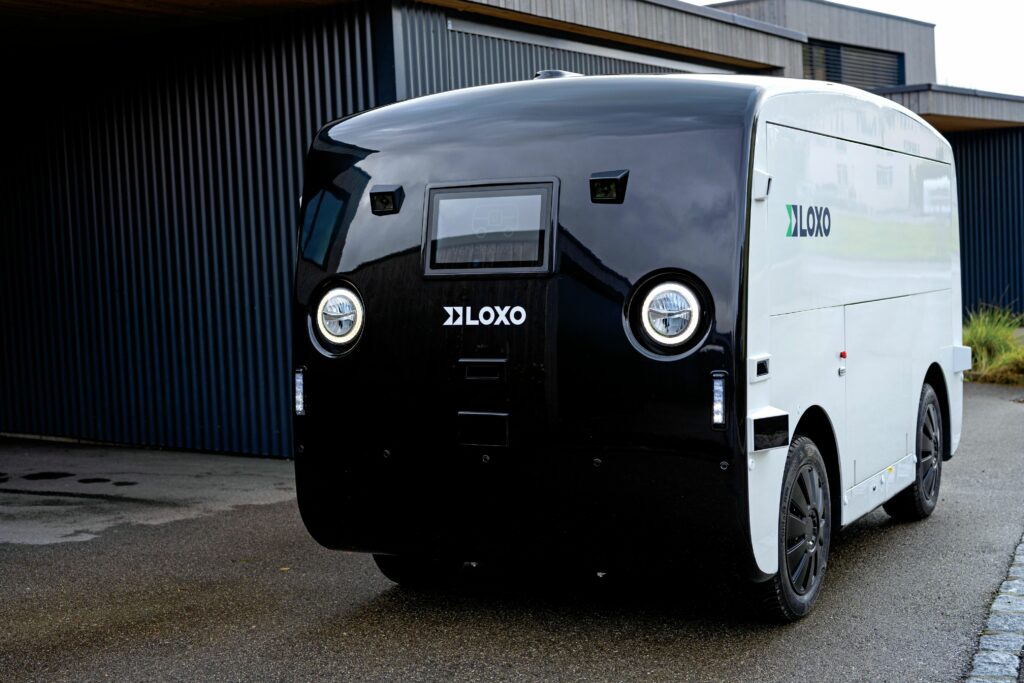
(All images courtesy of LOXO)
Rules of the road
Stalwart dedication to functional safety regs and bleeding-edge AI has brought about this company’s unique self-driving technologies, as Rory Jackson reports
As far as harsh proving grounds for autonomy go, Switzerland sets the bar high. A mountainous nation, many Swiss highways and other intercity roads pass through tunnels that blot out GNSS signals and create cramped, traffic-congested spaces that are deeply unforgiving to driving errors.
Even in the cities, tunnels remain widespread, with the Swiss Confederation’s city planners having eagerly distributed them. These, along with the frequently inclement weather causing risks such as tyre slip, sensor degradation and moisture ingress, are some of the principle challenges that Switzerland-headquartered LOXO has had to overcome in creating its self-driving road technologies.
As LOXO’s CEO and co-founder, Amin Amini, explains: “Switzerland has dozens of kilometres of highway tunnels, although as a company developing B2B self-driving systems, primarily aimed at last-mile and middle-mile delivery, we just have city tunnels to deal with.
“But, here in Bern, where we’re based and where we operate, our test vehicles have to drive through three specific and very lengthy tunnels on a daily basis just on their standard trial route. We would not have made it far as a company at all if we’d never learned to empower autonomous driving with something other than GNSS.”
Forced to tackle some of the most hazardous physical and environmental hurdles right from its starting line, LOXO has engineered a number of novel, software-based approaches aimed at overcoming key problems (not only within its own surroundings, but perennial across the self-driving world) and enabling the SAE’s Level 4 of autonomous self-driving intelligence.
These software solutions are enshrined within the LOXO Digital Driver (LDD). Similar to the Kodiak Driver product from Kodiak Robotics in the US (see issue 48), the LDD can be applied to virtually any road vehicle with the appropriate sensing, computation and by-wire control systems, and it is being exercised in real-world logistics applications.
While LOXO is not a vehicle manufacturer, it drew notable attention in December 2022 with the unveiling of the LOXO Alpha, a compact, self-driving electric truck that was purpose-built for driverless last-mile deliveries.
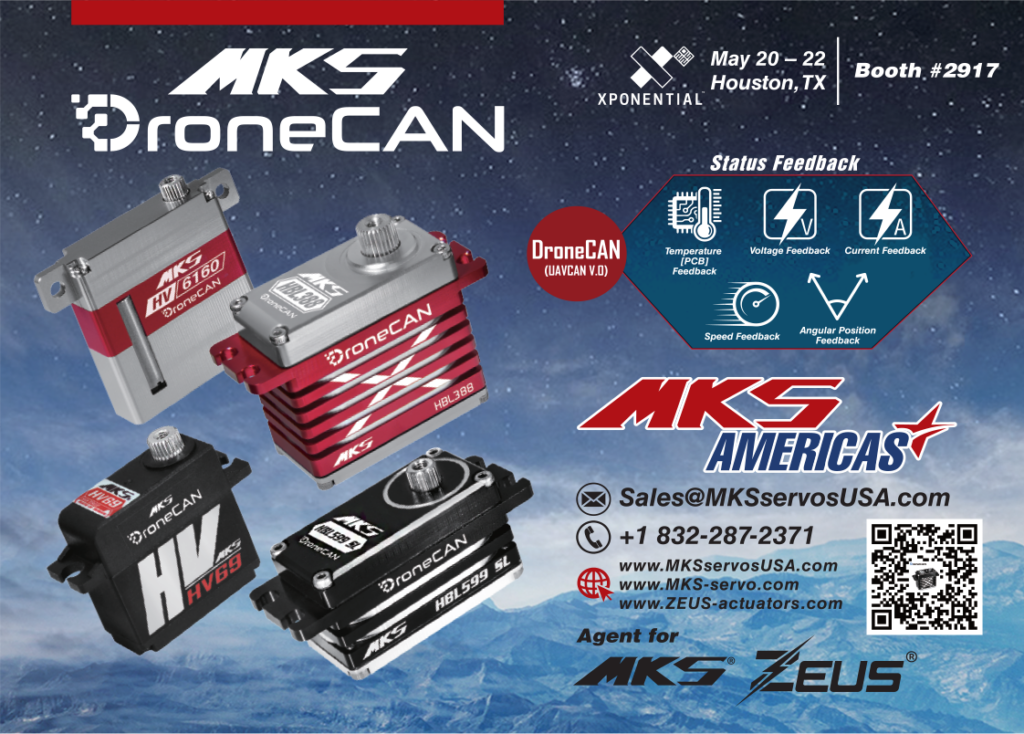
The uncrewed truck is powered by the Digital Driver, integrating a hardware stack which includes solid-state Lidar, radar, cameras, ultrasonics and redundant computers. Around that time, the Alpha was authorised to operate on Switzerland’s public roads, becoming the first autonomous Swiss vehicle to do so.
The Alpha has henceforth served as a crucial demonstrator platform, not only in service and operation trials in key partnerships such as that between LOXO and Migros – the largest retail company, supermarket chain and employer in Switzerland – but also as a safer, more acceptable self-driving technology.
Functional safety
Prior to the founding of LOXO in 2021, Amini and one of his fellow co-founders, CTO Claudio Panizza, had gained extensive experience as specialists in systems engineering and functional safety for the automotive industry. Both had worked at safety research centre ROSAS (Robust and Safe Systems), and Amini also co-founded CertX, which is now one of the world’s few accredited certification bodies for functional safety in cybersecurity, autonomous systems and AI robustness.
During that time, they were tasked with evaluating a number of autonomous solutions entering Switzerland from France, China and elsewhere, alongside many innovations from closely related areas of automotive technological development. Of particular note was how startups were struggling to achieve compliance with safety certification requirements; solutions arriving at Amini and Panizza’s doors were often three to four years of intensive re-engineering away from becoming anything certifiable.
“If you build an automotive system, and you don’t have a functional safety expert standing next to you taking part in the system design and engineering, you can easily wind up making something that ostensibly works great only to discover at the certification phase that it doesn’t work from a safety perspective at all,” Amini explains.
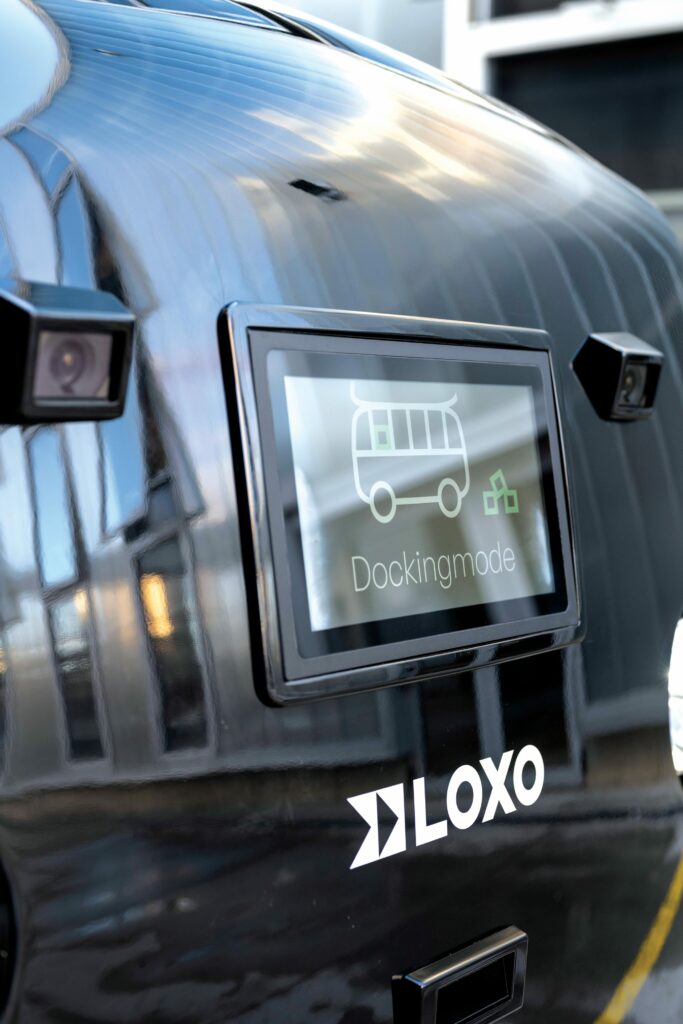
“After experiencing exactly that several times in evaluating these sorts of projects, we came to the conclusion that self-driving systems were quite far from having the performance required for us to be able to give the safety assurances needed for them to operate safely on Swiss roads.”
Shortly thereafter, starting in 2018, the pair became involved in an academic research project, aimed at determining how safety could be assured in autonomous driving systems through extensive self-driving performance tests, analytics and software engineering.
“Getting safety-certified without considering functional safety during the design and engineering phases means you need to make iterative changes on your architecture, which entails changing at least some portion of everything down to base code and retesting everything from scratch,” Amini says.
“It’s very, very expensive and time-consuming; hence our academic research was strictly focused on creating a system that would self-drive very proficiently and so pass – hopefully in one shot – all the requirements of functional safety regulations. That focus is exactly why the LOXO Alpha gained approval to operate on public roads. We accounted fully for functional safety during the engineering phase before going into prototyping.”
A big reason why the term ‘functional safety’ comes up so frequently in discussions of automotive certifiability (particularly for autonomous systems) is that ISO standards on functional safety, such as ISO 26262, cover around 3500 requirements and 180 engineering methods for hardware, software, system engineering, production, tooling, management systems and more.
“So you see, it’s tough. If we are being realistic, designing your vehicle with one safety engineer next to you actually won’t guarantee certifiability at all, because there’s no practical way one engineer can master 180 engineering methods. That fact made me very grateful to work both pre-LOXO and today with Claudio, whose specialisations complement my own quite nicely,” Amini says.
After three years, those involved in the research project were satisfied that the solutions developed through their r&d could bring a significant boost to the road safety of autonomous vehicles.
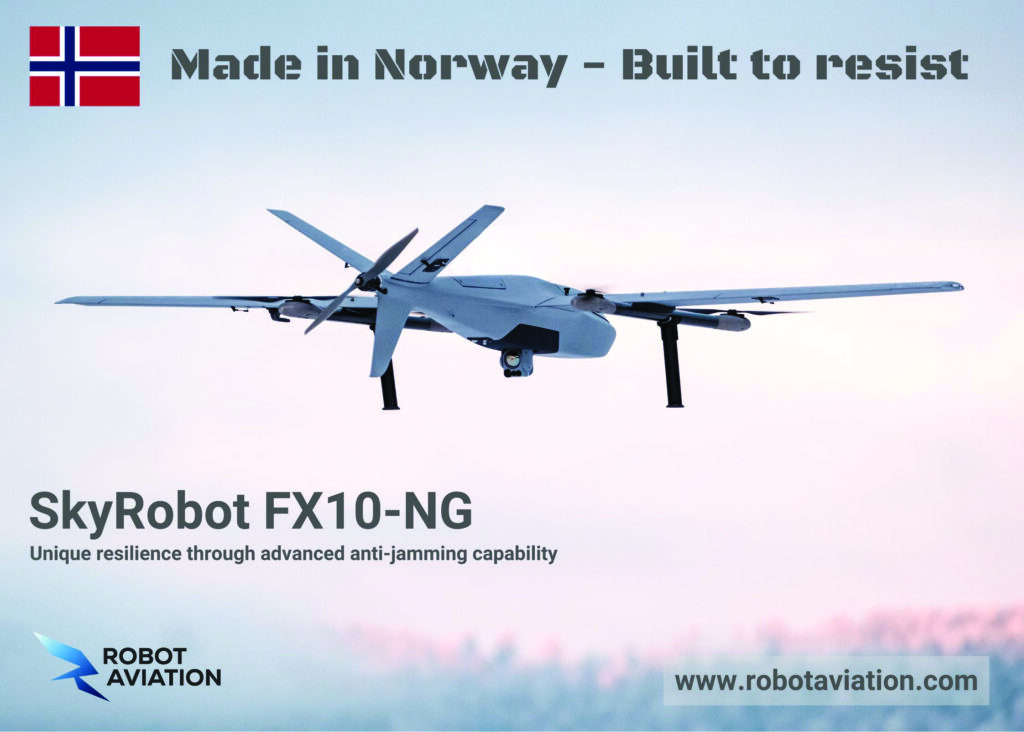
“That opened our eyes to the business opportunities this presented. Theoretically, a vehicle powered by the software we had developed would be proficient in transporting people or goods, able to travel at highway speeds or low inner-city speeds – many different applications,” Amini says.
“After some further research on this front, we felt there was an especially large space in Europe for autonomous goods delivery solutions, so we incorporated LOXO with that specific focus in mind, beginning the development of LOXO Alpha around that same time.
“But, even before that, we’d already been in discussion with some customers, such as the aforementioned collaboration with Migros, who after understanding our technology gave us the opportunity to do our pilot project with them.
“That meant a lot to us, as we became the first company in Switzerland to put a fully uncrewed, self-driving vehicle on Swiss public roads, and it also introduced our prototype vehicle to the public, many of whom really liked the design and gave good feedback on it.”
Shape of Alpha
The body and subsystems of the LOXO Alpha are mounted on a proprietary chassis supplied by an unnamed Chinese manufacturer. As a low-speed vehicle meant for operating within city limits, aerodynamics have played little to no part in the shaping of the body.
Instead, aesthetics were prioritised as a vital step towards public acceptance of the autonomous courier, especially at a time when public concerns and tension regarding self-driving systems are rising. Readers may recall how crowds in San Francisco surrounded and burned down a Waymo-owned autonomous Jaguar I-PACE in February 2024, followed by a flurry of videos being published on social media of Waymo cars making errors on public roads.
“Our goal with the outer body was to come up with a design – especially for the first driverless deployment of our technology – that was a bit cute, if I can say that,” Amini muses.
“Very intentionally, the vehicle seems to have a face, one that looks friendly, and its shape and size are unimposing. We surveyed those interacting with the vehicle, and concluded that 96% of people find it pleasant, convenient and easy to interact with,” he says.
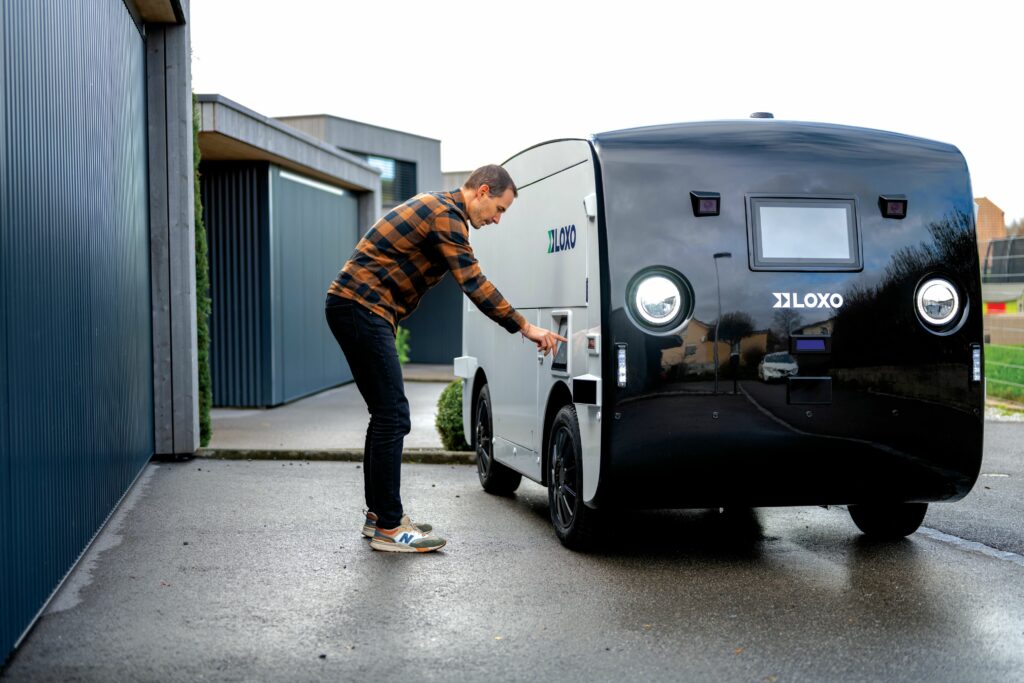
“Lastly, something else I’ve never liked about the majority of autonomous road vehicles is that their sensor deployments are often incredibly ugly. Their Lidars and cameras are bolted on, but not truly integrated into the body, so they look quite alien to the uninitiated, so we focused very much on integrating our sensors inside the body, such that most of them are barely visible unless you stand very close to it.”
The LOXO Alpha measures 3.5 m long, 2 m tall and just under 2 m in width, with an empty weight of 800 kg and a carrying capacity of 350 kg. Multiple concepts for the size and volume of the vehicle were drawn up in the design phase, with the eventual dimensions of the Alpha selected to cover the average distances, cargo quantities, and trips per day common to European last-mile deliveries, with LOXO’s prospective customers providing extensive input on that market research.
Similarly, the battery was sized to enable the typical ranges of last-mile deliveries, with up to a 100 km range between charges from the uncrewed vehicle. Within its weight category, the Alpha can drive at up to 45 kph. Throughout the pilot project with Migros, a 30 kph speed limit was observed for safety reasons.
Identifying and prioritising these various configuration and performance requirements occupied the first three to four months of r&d in 2021, alongside significant software development (given that LOXO is principally a software company and not a hardware provider).
“Because of this focus on software, the Alpha’s body and compartments have been built on a pre-existing chassis platform, which meant we didn’t have to develop and engineer a whole platform ourselves, and we already knew which suppliers were the right ones because we’d already looked into it and trialled some components during the research project,” Amini recounts.
A few months of integration took place after the evaluation of requirements as components arrived in-house and were assembled together. These included the body panels made from a fibreglass epoxy composite, chosen as a less expensive and complex alternative to carbon.
Fibreglass is also used for its reasonable mechanical stability, as well as its aesthetics (Amini notes such qualities are why fibreglass is increasingly used in the automotive industries). Additionally, the lack of access to overseas material suppliers during the COVID years limited the availability of cost-effective carbon composite during prototyping.
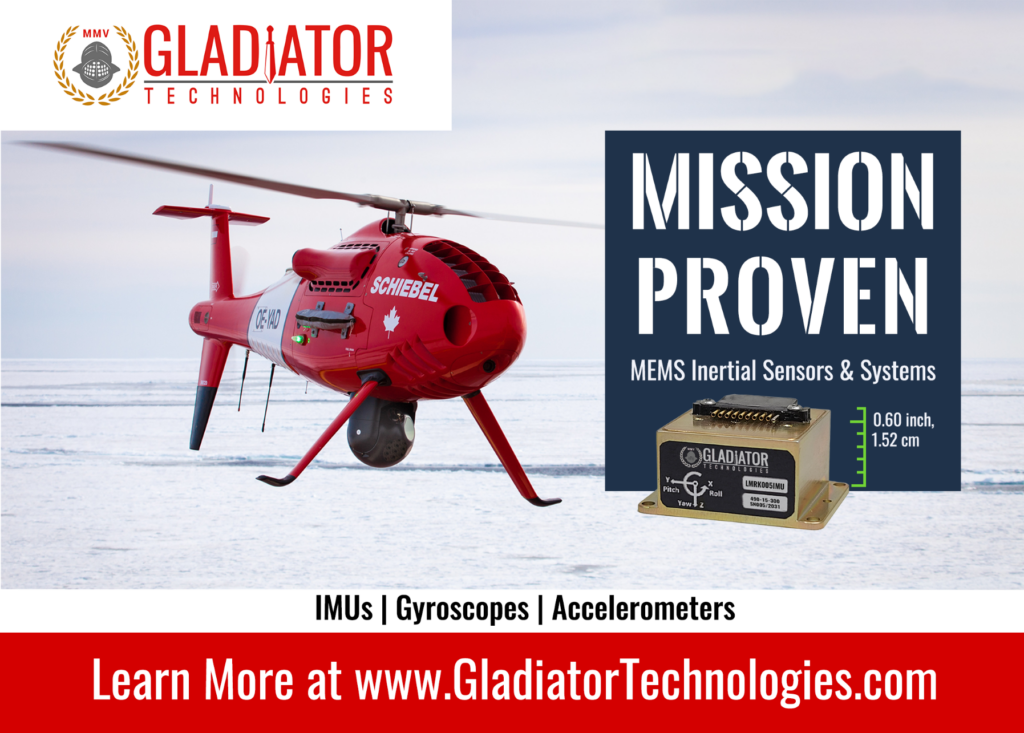
The body and electronics move around on a powertrain featuring four in-wheel hub motors, with this configuration enabling redundancy in propulsion, as well as in braking and front and rear steering. Each motor’s design comes with in-built suspension, through which it integrates with the chassis.
The battery is charged over six hours via an AC connection, this having been a key market requirement. European customers and operators of last-mile delivery services expressed to LOXO a dislike for the high battery replacement costs associated with fast charging (and such services only operate in the daytime, so slow charging during the low-tariff nighttime hours is strongly preferred).
Integrating sensors, computers and software took the lion’s share of work, followed by roughly six months of testing and another six months of work to achieve road authorisations (making about 18 months of r&d to get the prototype Alpha ready for operation).
Sensor complement
The bill of sensors has been painstakingly crafted and laid out around the vehicle to satisfy functional safety requirements from the beginning (rather than making the error of leaving functional safety as something to be achieved later on), with Amini estimating that LOXO must have had discussions with every possible supplier of the sensor technologies used on the Alpha.
“As a key part of that we went straight for solid-state Lidars instead of conventional Lidars, which spin their lasers 360° using a rotating mirror,” Amini says. “The principal issue with the mechanical design of those latter, standard Lidars is that, in an automotive context, road vehicles can frequently suffer a lot of shaking and vibration.”
Such conditions, in LOXO’s experience, can throw off point measurements far enough that spinning mirror Lidars may not consistently achieve automotive functional safety ratings. As a result, the company has installed three InnovizOne Lidars from Innoviz Technologies on its vehicle.
As well as that company implementing ISO 26262 in the software of its Lidars, ASICs, detectors, MEMS and other hardware from their early development stages, Innoviz has created its software in compliance with ASPICE (Automotive Software Process Improvement and Capability dEtermination) standards, and it has selected its supplier manufacturers to meet the IATF 16949 standard on quality requirements for automotive management systems.
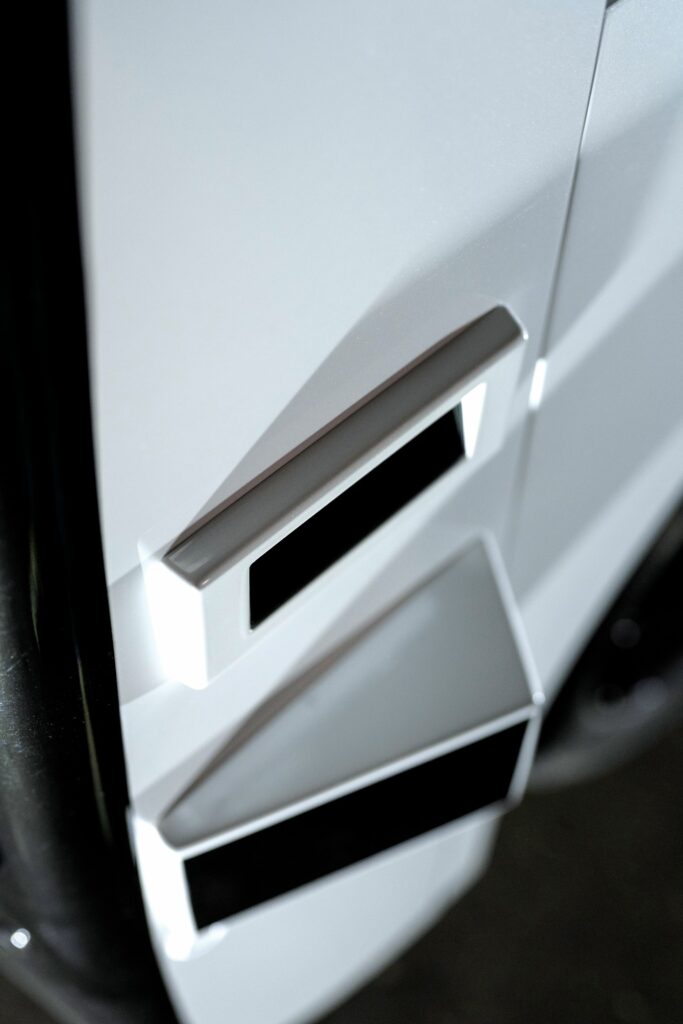
The InnovizOne Lidar measures 45 mm high, 111.4 mm wide and 97.9 mm long, and it has a 115° maximum horizontal FoV (with up to 25° in the vertical plane). It operates with a detection range running from 1 -250 m away, an angular resolution of 0.1° x 0.1°, and a frame rate of either 10 fps or 15 fps (as pre-configured by the end-user).
Additionally, its developers say it measures points with no gaps between pixels, with multiple reflections per pixel, and with a pre-configured “region of interest” in the centre of its FoV (measuring 20° wide by 8° tall), in which laser energy is focused to increase the detection range (although the resolution is kept uniform over the vertical FoV to avoid missing key data at the edges, such as at road level or above the vehicle).
Meanwhile, a set of five 4D imaging radars has been integrated, as an accurate and fast solution for tracking the position and velocity of objects around the vehicle, deemed critical by LOXO (and many others) for automotive safety.
Five cameras are installed concentrically around Alpha; the cameras integrate a protective glass layer over their lenses which is continuously heated to provide an anti-icing function helpful for getting continuous clear vision in the cold climates of Alpha’s trials and operations to date.
“We have effectively no blind spots around Alpha, thanks to how we’ve mounted the sensors. And given that it’s most critical for safety rating the vehicle that we cover the frontal area in which it spends the most time driving, our sensor arrangement overlaps the Lidar, radar and camera detections such that they provide three layers of redundancy in the forward direction,” Amini says.
“In terms of how we’ll do the sensors on converted vehicles: we showed a Volkswagen ID Buzz cargo van we nicknamed ‘Mathilde’ in Bern last year, which integrated our self-driving software stack as well as a sensor complement almost exactly the same as Alpha’s. There were only small differences in the radar, camera and Lidar arrangements.
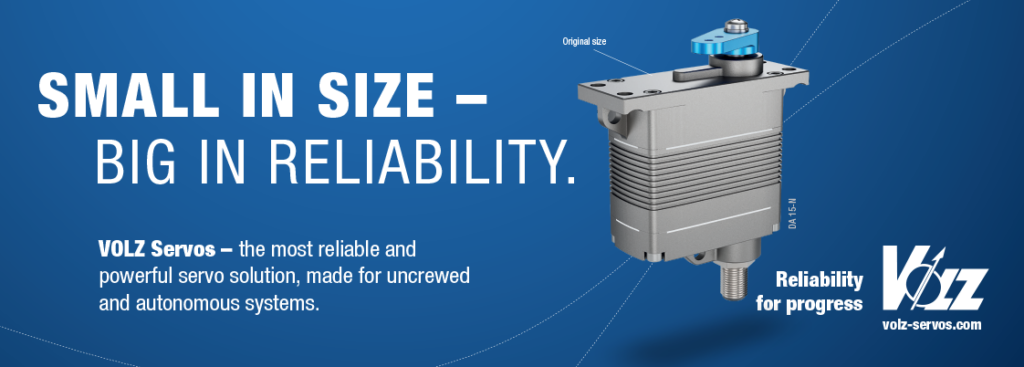
“Specifically, we didn’t change or install the radar because the ID Buzz already has its own, the cameras are positioned a little higher because the van’s body is bigger than Alpha’s, and there is a fourth Lidar installed on the back, again to ensure effective coverage around a vehicle of the ID Buzz’s size.”
The last perception sensors onboard are ultrasonics, integrated about the vehicle for low-speed, energy-efficient detection of surrounding objects during parking manoeuvres.
For conventional localisation input, Alpha integrates satellite navigation receivers with both differential GNSS and RTK GNSS available. The former provides higher accuracy than standalone GNSS thanks to some corrections in real time, and the latter provides the highest possible accuracy to centimetric levels (as many of our readers will know).
Beyond all of these sensors, significant further processes and technologies onboard feed into the LOXO Alpha’s localisation methods, as the company explained to us.
End-to-end AI
In our past investigations of self-driving road vehicles, we have seen vehicles and software that used forms of simultaneous localisation and mapping (SLAM), combining multiple low-level sensor inputs to understand their location and surroundings.
We have also seen perception systems using machine vision based on convolutional neural networks (CNNs) and image data-based training to recognise and classify objects, placing annotated bounding boxes around them to signify image recognition and segmentation to developers and remote monitoring staff. The latter perception system will also sometimes inform a separate onboard localisation system when recognising landmarks or clusters of structures specific to known streets.
Both systems are well-established, and are often used to inform additional onboard algorithms for path planning and control, as is the case in the LDD and the Alpha. However, certain perceived insufficiencies drove LOXO to develop a third, more unique component of its driving intelligence, which typically takes precedence in self-driving decisions.
“If you engineer your perception, localisation and path planning as separate input streams, you’ll eventually have to integrate and harmonise for the control system, and this is where problems start to appear,” Amini says.
“In software engineering, integrating different functions is the hardest challenge. It can cause lots of safety issues because you might have multiple different AI models and hence conflicting sets of logic, and it’s not always clear in the first place what the best way to combine differing AI models is. You can wind up losing considerable vehicle performance because engineers are forced to program the vehicle to constantly fall back on the safest of its available movement or braking options due to the lack of confidence in the data and decisions that get through.”
Therefore, while both SLAM and a conventional perception algorithm trained through a CNN are used in the LDD and Alpha, LOXO has also engineered an autonomy approach it refers to in-house as End-to-end, which its systems use for their primary navigation and control intelligence, thereby avoiding the hamstringing of vehicle performance that may otherwise have been incurred.
This approach is centred around an AI model called LOXO Fuser, which consumes the raw, unprocessed sensor data inputs and uses them to output vehicle commands. Rather than the model being based on separate localisation, perception and path-planning algorithms, its workings have been trained and optimised using transformers (also called transformer neural networks or TNNs).
“ChatGPT is well known to many now – the ‘T’ stands for ‘transformers’. They are a fairly new mathematical discipline, now being used across the world of AI. Their working principle is that they operate using a multi-head attention mechanism, or attention models, which allow us to identify which items of incoming information are the most important ones in a given context,” Amini explains.
“In the case of autonomous driving that means pixels of sensor data can be marked or weighed against each other for relative importance. Compare that to conventional autonomy software, which gives equal importance to every single pixel. Through our transformers, our LOXO Fuser model has been able to learn, with increasing aptitude, what information is most important – and thus merits the most attention – throughout different driving scenarios.
“So long as we define the driving contexts effectively, the model will continue to apply the smartest and most appropriate autonomous driving behaviours in response to each situation, as detected by the sensor architecture.
“When ChatGPT seems to proffer apt witticisms and even empathise like a human would, it’s because its makers trained their models with their transformers to pay attention to the most important details of the information being fed into it. Likewise, in autonomous driving, End-to-end means the LDD will always heed things like lane markers before noticing clouds in the sky.”
Broadly, the architecture of the transformer used by LOXO consists of two main layers: an encoder, which processes input vectors composed of the raw sensor data coming in from around the vehicle, and a decoder, which decodes relevant and actionable detail from the encoded information generated by the encoders in order to correctly allocate attention, and subsequently output decisions.
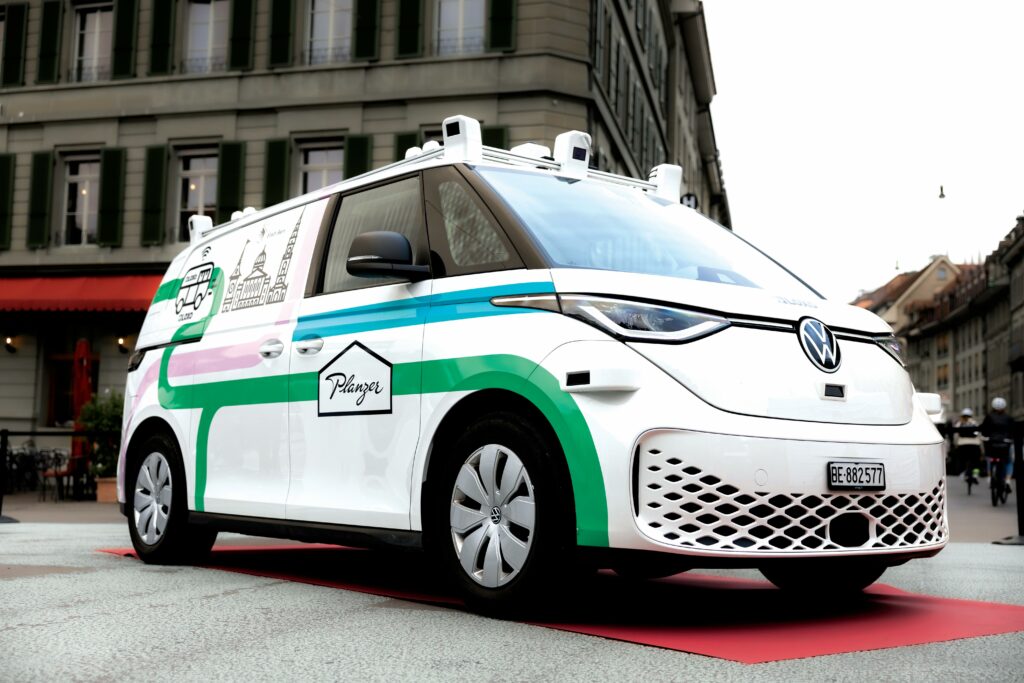
The encoder-decoder transformer model works functionally and quickly enough that it bypasses some of the issues that can arise from using CNN-based perception, such as when objects are not correctly categorised or segmented, or recognised as facing a particular direction, and the vehicle makes a wrong driving decision.
By using just the raw inputs, and focusing attention based on critical factors such as velocity, proximity and placement, the Alpha successfully focuses its processing power on avoiding collisions, regardless of what categories nearby objects might fall into.
Training the transformer
Information on which pixels matter more than others was imparted to LOXO Fuser during the training phase, with the model consuming annotated data in a fashion not dissimilar to CNNs and other deep-learning architectures.
This began with LOXO’s engineers repeatedly showing road and traffic scenes to the model, with annotations of pixels corresponding to such key items as lane markers, traffic lights, road signs, cars, pedestrians and pre-assigned parking places for offloading deliveries, such that Fuser learned how to implicitly read the context of the specific situation around the vehicle before gradually learning how to read and react to more hazardous situations.
The training data was amassed by LOXO and some key collaborators, including public transport companies who agreed to let LOXO integrate sensors onto its vehicles for collecting pixels on their day-to-day routes, enabling vast quantities of data covering different levels of traffic and countless sporadic events on both roads and pavements. Meanwhile, LOXO’s engineers drove sensor-equipped road vehicles of their own to collect training data, including outside transport routes and hours.
“You never know when you’ve gathered enough data, but it’s very important to note that transformer models like the one we use are much more intelligent than classic CNN-type deep-learning models,” Amini says.
“That is to say, they’re much faster, and if you give both a CNN and transformer the same quantity of training data to consume, typically nowadays you will find the transformer gains much more intelligence than the former.”
Safety software
Despite all the advantages of the transformer-based LOXO Fuser technology for autonomous driving and quick, apt decision-making, LOXO notes that the AI model is not conducive to effectively demonstrating compliance with functional safety standards such as ISO 26262 to regulatory and certifying bodies (those bodies being especially strict in Europe).
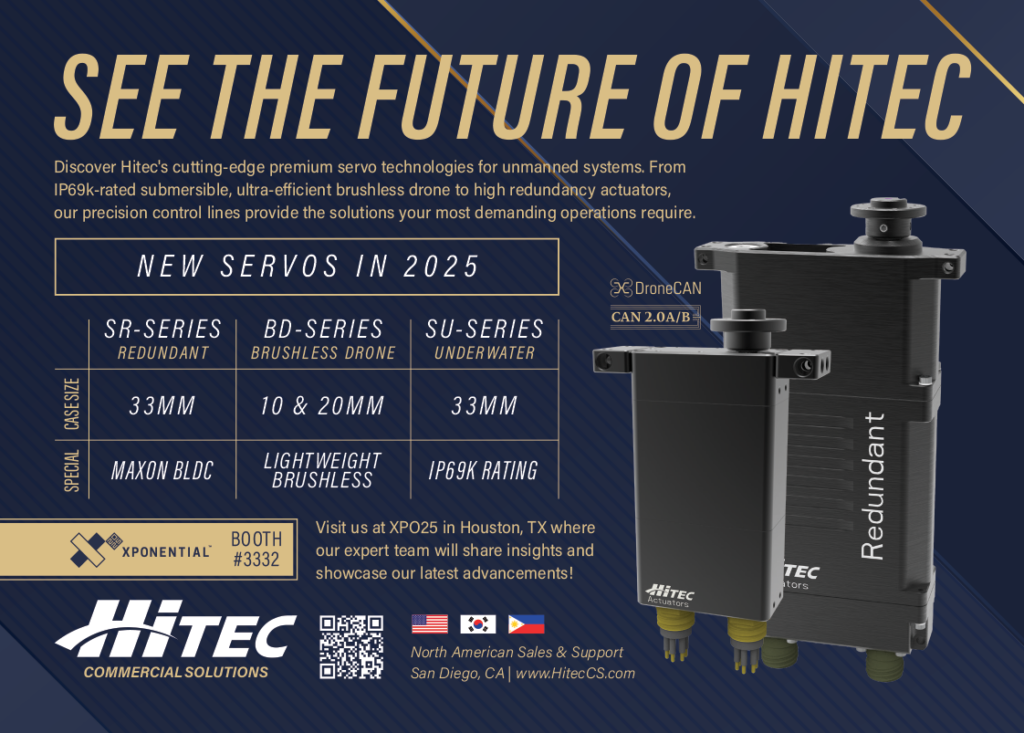
“That’s because around 40% of the functional safety requirements are, by the way they’re defined, not presently applicable to our AI model. Therefore, we’ve developed another subsystem in parallel with LOXO Fuser, which we call LOXO Guard,” Amini says.
“LOXO Guard is purely deterministic software, which takes responsibility over implementing functional safety requirements. It doesn’t do the same tasks that LOXO Fuser does; instead, it monitors the latter’s command outputs before they get sent to the main control unit on the vehicle in a fashion that meets the outstanding regulatory definitions laid out across those functional safety requirements that the Fuser doesn’t precisely capture.”
LOXO Guard additionally uses the onboard, CNN-based perception system in standard operations as a valuable point of reference, against which the LOXO Fuser’s decisions can be validated.
In addition to LOXO Fuser, the company has developed its own supervision software (analogous to GCS software systems we have previously featured), to cover the remaining, outlier situations that the Alpha and other LDD-powered self-driving vehicles may not know how to handle.
Using this software, a remote supervisor can monitor the vehicle’s surroundings and activities, and send decisions if needed. As a reminder, conventional image and point-cloud data are still generated by the sensors onboard the vehicle. These can be viewed by the supervisor at their station in real time, except for whatever glass-to-glass latency is incurred. As standard, LOXO’s vehicle architecture includes a modem with simultaneously operable 4G/5G radios, with multiple network providers and an embedded computer for dynamically switching between providers and cellular links to ensure optimal coverage and bandwidth.
“However, as an added safety measure, the final decision is always taken by the vehicle, so when we say the supervisor can send decisions to Alpha, we’re not talking about remote driving or teleoperation,” Amini notes.
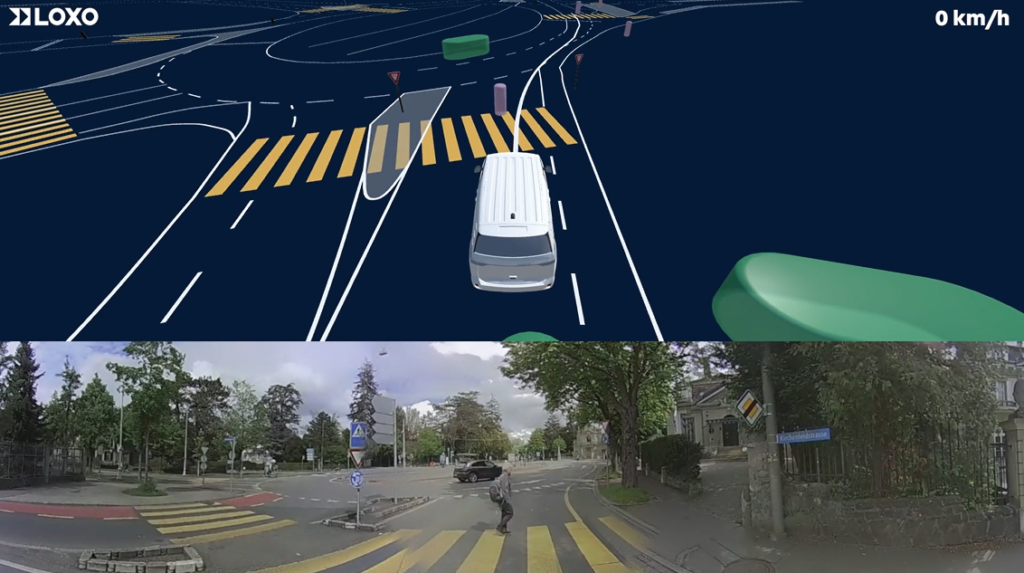
“The supervisor is essentially proposing manoeuvres for the vehicle to consider, based on the sensor information and all other vehicle telemetry and subsystem health data, which they receive in real time back at their station.
“Those manoeuvres can include emergency braking, avoidance and so forth, but if the vehicle has determined that the proposed manoeuvre presents more risk than is acceptable, it can refuse the decision.”
This ability prevents errors by supervisors – or ill-intentioned attacks by hostile agents – from manipulating Alpha and LOXO-modified vehicles into unsafe movements. Readers may recall that WeRide (issue 58) also engineered the intelligence on its RoboBus and in its remote monitoring software, such that the ultimate decision-making power lay with the autonomous vehicle and its main computer.
“As a key part of preventing errors in our code, we used some solutions from the company Vector in Germany to demonstrate that if you’re compliant with functional safety standards, your code needs to be tested according to the specific, relevant standards, and it takes certified and very, very sophisticated tools to validate your source code this way,” Amini adds.
SLAM
LOXO’s SLAM algorithm has been developed in-house to form a robust third layer in the company’s strategy for software redundancy and diversity in its autonomous driving.
The main principle of LOXO’s SLAM is that data from the Innoviz Lidars, while also transmitted to the two separate and highly different deep-learning-based systems, travels further downstream to a sensor-fusion engine, which combines the three InnovizOnes’ data with IMU information (for velocity and attitude parameters) into a single, 3D point cloud, indicating the vehicle’s position within its surroundings.
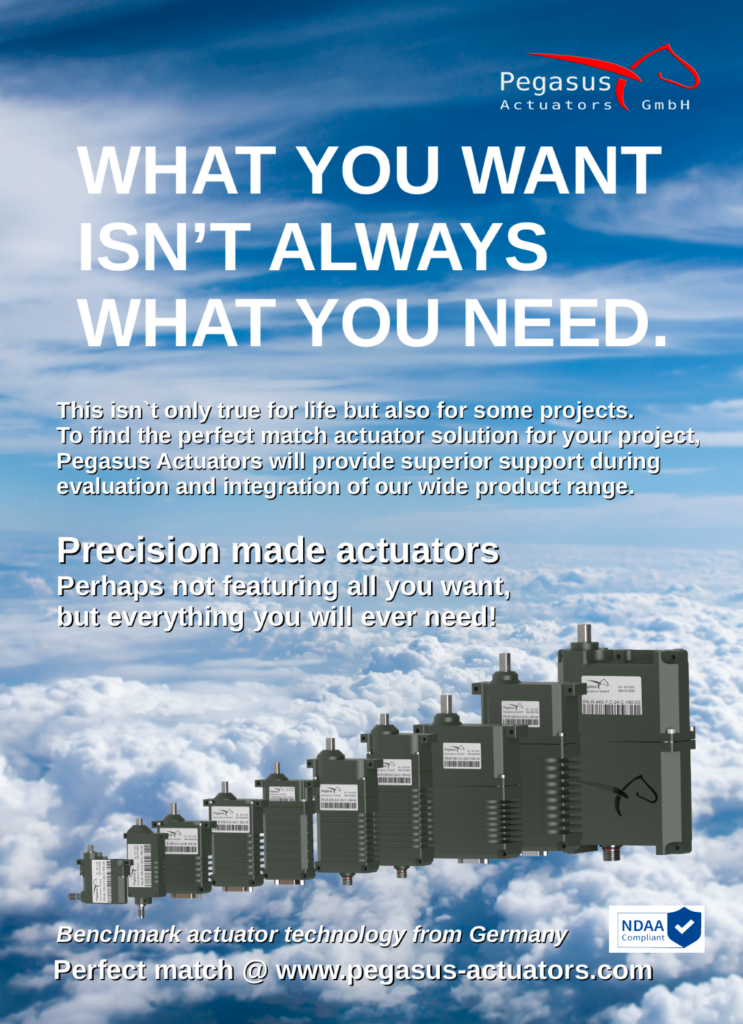
To create its Lidar SLAM model, LOXO learned and took inspiration from a range of papers, both recently published and long established, before using this to write its own code in C++. Key items written into the model’s algorithm included high-fidelity tracking of how points within the point cloud moved from one moment to the next, and real-time monitoring to prevent error accumulation.
“SLAM error minimisation also depends on the quality of your sensors, how well you can fuse the sensor data in a time-synchronised way, whether you’ve integrated powerful and redundant automotive-grade edge computers, and how much you’ve optimised your software, such that it monitors, reacts and even fails over to redundant modules in real time, and so that it doesn’t consume too much power for other onboard functions to continue effectively,” Amini notes.
“Downstream from the sensor-fusion engine, we have a combinator engine that combines our RTK- or D-GNSS data with our Lidar SLAM to further detail the vehicle’s localisation information. We had tried visual SLAM in the past, based on camera data, and it does work, but Lidars are inherently the more powerful sensor, especially with the model we use, so Lidar was the better choice for functional safety.”
While the Lidar SLAM and RTK-GNSS provide different and redundant sources of localisation information, the combinator provides a very precise location fix while also tracking for potential errors in either stream if mismatches occur, and it hence carries intelligence for deciding if the vehicle should switch between one channel or the other.
Switzerland’s plethora of tunnels provide the most obvious reason for why LOXO programmed the combinator module to know when to switch from one localisation stream to the next: in any GNSS outage, the Alpha will rely on Lidar SLAM. However, the high precision and fast update rate of the RTK-GNSS means it still plays an important role in LOXO’s localisation, particularly as its accumulated errors over time will naturally be the lowest of any onboard sensor (especially as Alpha’s low speeds and frequent stops between deliveries minimise the chance of GNSS error).
To refine its AI models – SLAM, transformer and CNN-based – LOXO used multiple simulation methods, feeling that no single simulation software could be free of weaknesses and so using only one would mean limitations in how far it could validate the models.
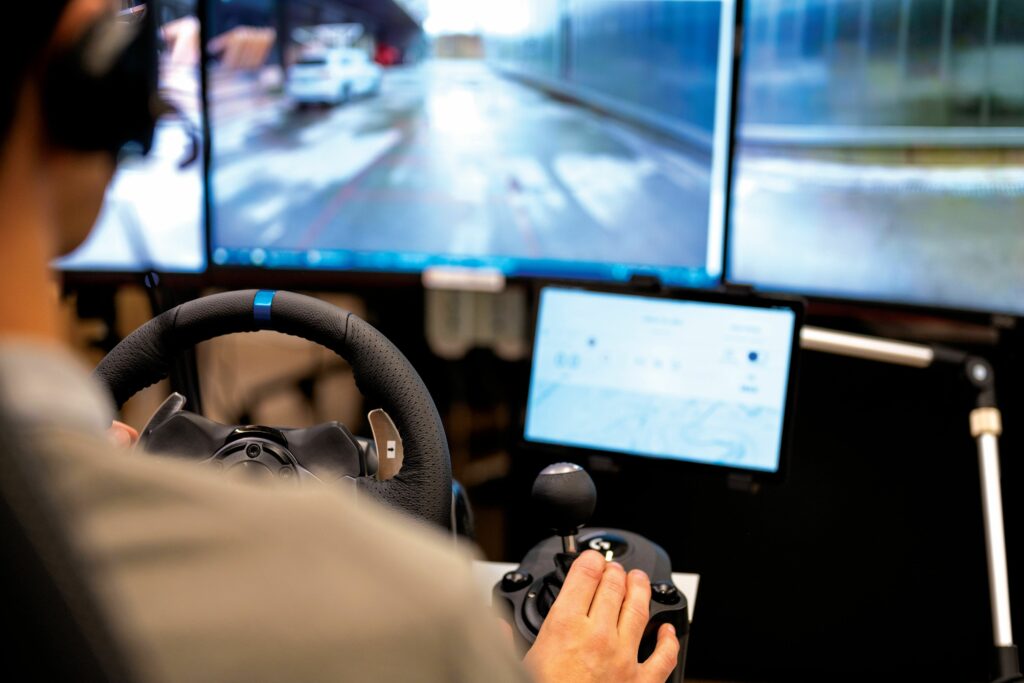
making, although ultimate authority lies with the vehicle to prevent unsafe interventions
However, most validations of the Lidar SLAM and Lidar-derived elements of the deep-learning-based autonomy algorithms took place on permitted roads (including on an automotive proving ground near LOXO’s headquarters and on the roads around the innovation park where its HQ sits) due to the considerable limitations of point-cloud simulation technology at present.
Two computers plus one
Besides three redundant streams of navigation software and four redundant motors at the output end, LOXO’s vehicle architecture centres around what is effectively dual redundant computer architecture.
“The first of the two computers can be thought of as our high-performance computer, and the second is there to execute the functions under LOXO Guard, which pertain to functional safety requirements, analysing incoming streams of data from the first computer to check and approve LOXO Fuser’s decisions against information output by the other two software AI models,” Amini says.
While the exact models of computer installed will vary based on the project budget, as well as exact customer operational and fleet requirements, the former of the two computers is typically based on an Nvidia GPU-powered solution, such as the Jetson Orin, to achieve the high computing power and performance needed for LOXO’s AI models.
The company also notes that some hardware suppliers now provide the latest Nvidia autonomy computers within automotive-grade hardware modules, built to be compliant with (and hence certifiable to) ISO 26262, and therefore present great promise for future integrations and collaborations.
“Meanwhile, we use a range of certified, automotive-grade computers for running safety-critical functions, which are available commercially on the market,” Amini says.
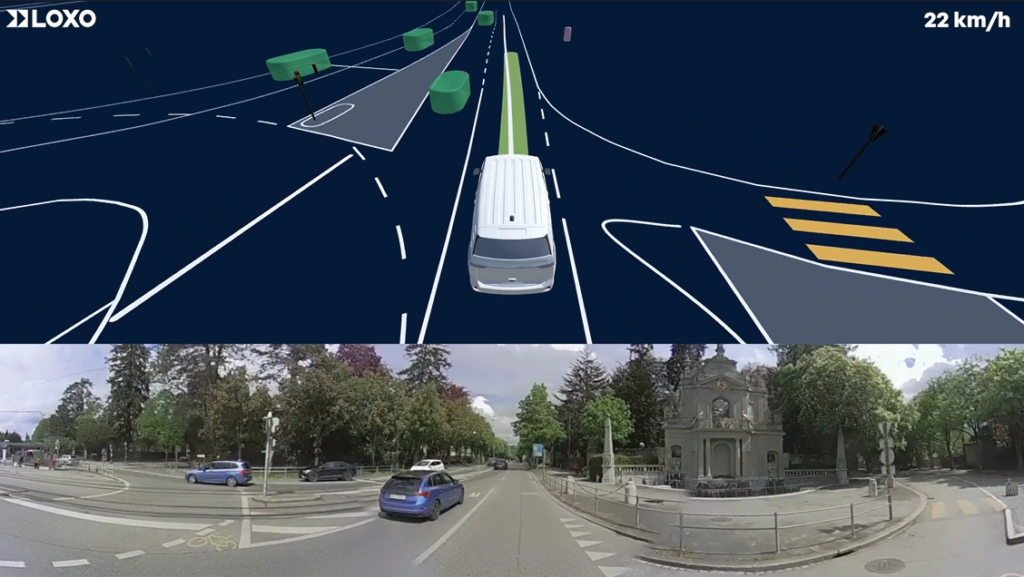
“Finally, we have a third computer, which essentially monitors the health of the two primary operating computers to ascertain that they’re functioning and interacting correctly.
“If one or both are not doing so, that third computer can take over the self-driving functions in a degraded mode. That mode consists mainly of just using the Lidars and a very slow driving speed to pull over and get off the road safely.”
Although the computer software described in this feature may sound complicated, the architecture’s middleware is built around the now-familiar ROS and ROS-2, with LOXO planning to migrate fully to ROS-2 in the near future.
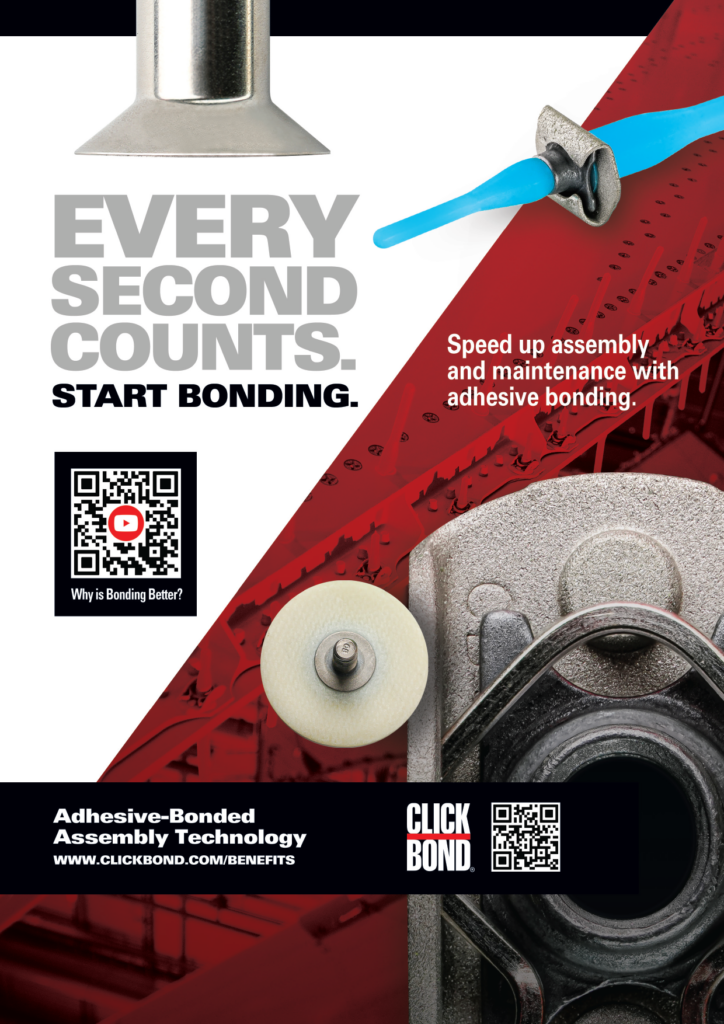
As one may expect, all data signals throughout the vehicle are transmitted over a network built on CAN 2.0b, being the most widespread safety-rated automotive standard, as well as an efficient tool for prioritising the delivery of data critical to safe driving.
Beyond valley and mountain
In late 2024, LOXO successfully launched its newest project area in Bern, for goods delivery with Swiss transportation and logistics firm Planzer. The Swiss autonomy company says this project area constitutes the largest testing zone (by geographical area) to date for an autonomous road vehicle in Europe, encompassing a street network totalling roughly 67 km of Bern’s challenging public roads and tunnels.
The pilot project is slated to run for two years, principally using one of the aforementioned Mathilde vehicles to autonomously deliver packages across multiple middle-mile routes to 14 distributed micro-hubs. From those, the packages will be transferred manually to smaller electric vehicles for their last-mile delivery.
Mathilde will, notably, be as uncrewed as Alpha in this project, fitting the SAE Level 4 bill closely; that is, working with no onboard safety driver and practically full autonomy, but within a defined and geo-contained area.
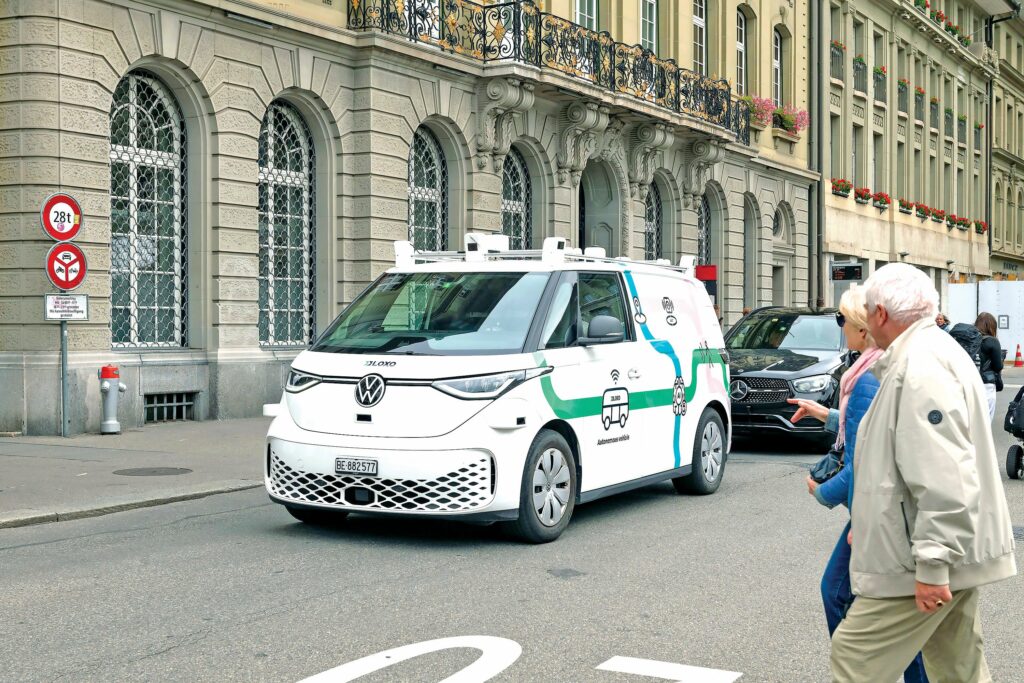
If the collaboration successfully improves the efficiency of Planzer’s logistics (including emissions reduction, given that increasingly more uncrewed vehicles in operation seem to expend less energy than their crewed counterparts), Planzer and LOXO will expand the project to include other cities.
In the meantime, LOXO has also opened its first subsidiary outside of Switzerland in Munich, the company having identified Germany’s middle-mile logistics market as being worth hundreds of billions of euros, and the country’s legislative environment being progressive towards the further development of self-driving vehicles. With new regulations on automated driving expected to be published later this year, one can no doubt expect LOXO and its vehicles to remain ahead of the game.
Key specifications
LOXO Alpha
SAE Level 4 autonomy
Battery-electric
Four in-wheel hub motors
Dimensions: 3.5 m x 2 m x 1.9 m
Empty weight: 800 kg
Cargo capacity: 350 kg
Range: 100 km between charges
Maximum speed: 45 kph
Operating speed: 30 kph
Some key suppliers
Lidar: Innoviz
Lidar: Additional companies
Certification advisory: CertX
Code testing services: Vector
High performance computers: Nvidia
Maintenance and repairs: Noviv Mobility AG
UPCOMING EVENTS
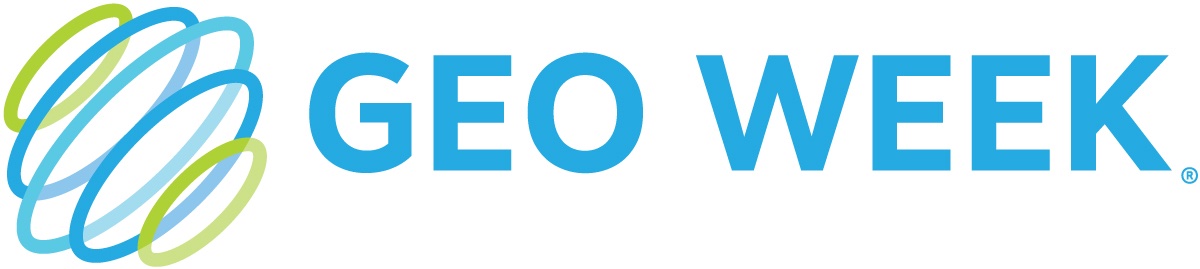
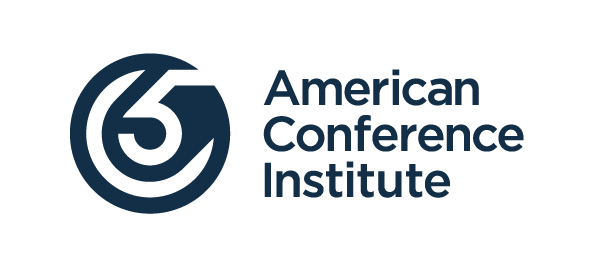
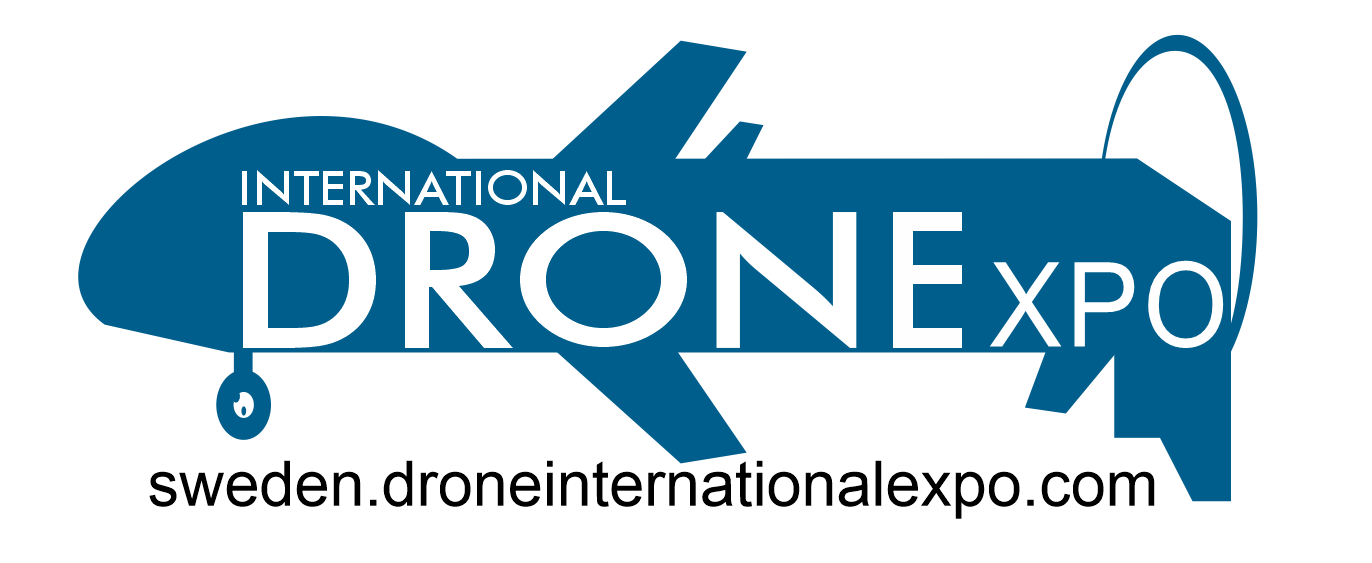
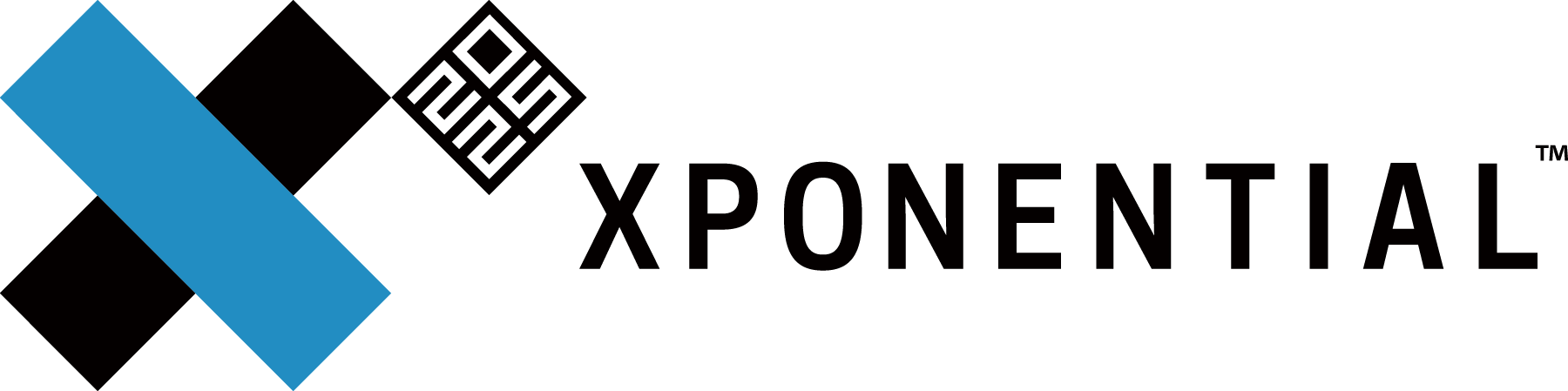
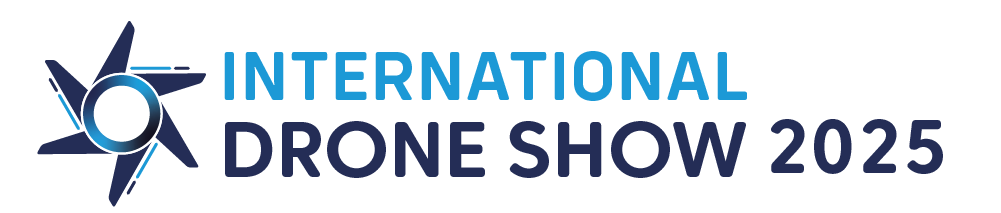