Dr. Cara LaPointe
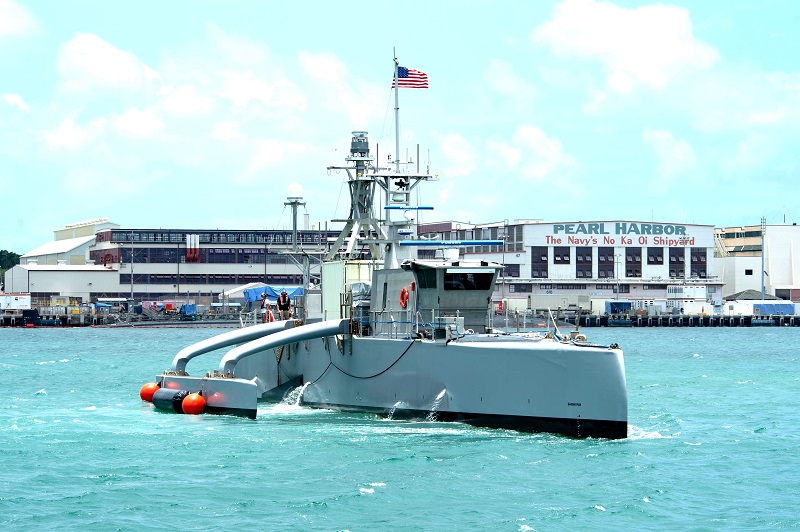
The co-director of the Johns Hopkins Institute for Assured Autonomy tells Peter Donaldson about how to gain society’s trust in AI and uncrewed systems
A question of trust
There can be few more ambitious goals than ensuring the safe integration of AI and autonomous systems into society. In essence, that is the mission statement of the Johns Hopkins Institute for Assured Autonomy (IAA) and, by extension, of its co-director Dr Cara LaPointe.
A marine engineer, diver and former naval officer, LaPointe likes to define that as creating trustworthy technology and building trust. “We are operating at the intersection of technology and humanity,” she says. “These systems will never be used if people don’t trust them.”
With a background in engineering, science and the arts, LaPointe says it is vital to combine technical knowledge with an appreciation for the humanities across different cultures if developers are to understand what people need from technology. She illustrates the importance of that with an experience she had in her early 20s as a student on the island of Yadua, Fiji, where she studied the impact of a novel technology on the indigenous culture.
“In that case, the novel technology was a motorboat that gave people on the remote island easy access to the main islands, so the subsistence economy was transitioning to a cash economy, and that was disrupting the traditional common property resource management regimes and governance structures,” she recalls.
In creating assurance, it is critical to create AI and autonomous systems that people from diverse backgrounds will trust. “What it will take for me to trust them is different from what it will take for you to trust them, and completely different from what it will take for the people of Yadua to trust them,” she says. “There are a lot of complex social science issues embedded in this, which is why we take a multi-disciplinary approach.”
Run jointly by the Johns Hopkins Applied Physics Laboratory (APL) and the Johns Hopkins Whiting School of Engineering, the IAA works by engaging with academia, industry, governance bodies and the military to draw on expertise from different disciplines.
The IAA extends this approach to uncrewed systems, an example being the partnership it formed with AUVSI in 2019 to provide an opportunity to bring together researchers, technology developers, users and regulators to address assurance issues.
A major focus of the IAA’s work, LaPointe adds, is aimed at directly helping engineers through the development of tools and methods of assurance that can be generalised across different types of systems and multiple domains, from uncrewed vehicle systems to medical robotics. “A big piece of that is starting to develop a comprehensive and robust systems engineering process for intelligent or learning-based autonomous systems,” she says.
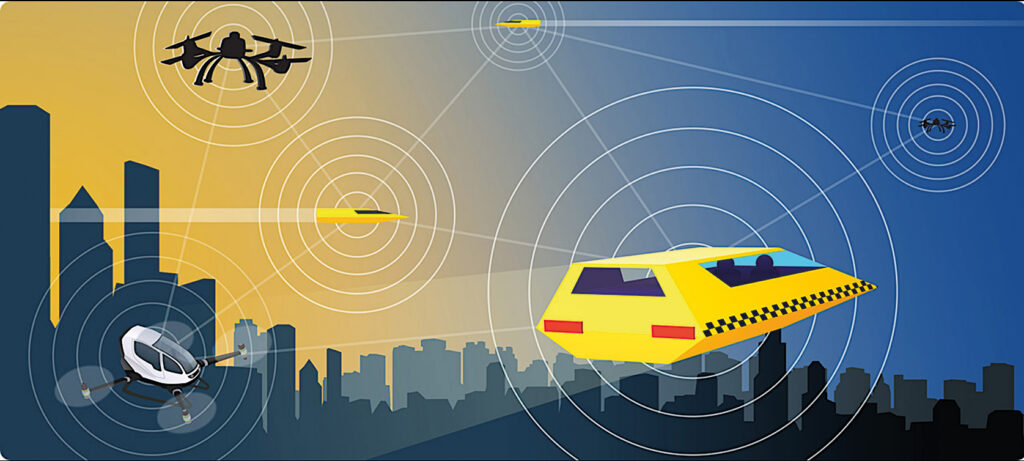
‘Guardian’ tools
Ultimately, this could result in a set of standards analogous to those that make sure certified aircraft are safe, but LaPointe cautions that before such standards are possible there is a lot of fundamental research to be done. “The problem with learning-based systems is that, often, we can’t predict where the safe operating envelopes are going to be, as there are edge cases that we don’t always know exist,” she says.
“There are so many amazing potential benefits of autonomous systems and AI, and we want to realise all of them. At the same time, there are potential negative impacts that are either unintended consequences or the results of malicious actors manipulating the technology, so we need tools to provide guard rails.”
Those could be cyber security tools or tools to make sure developers understand their datasets. If there is something wrong with the data, it might be because someone is ‘poisoning’ the dataset, or it could simply be that a system’s sensors aren’t working very well and are therefore providing poor data.
“There is no silver bullet, so we need a set of tools and processes to help us throughout the life cycle of the technology,” she says. “We need tools to help us better drive requirements for technology.
“That is where the human need for trust comes in, and you have to have a feedback loop between that and the requirements for how you develop a system. We also need tools for the design and development phases.”
One crucial decision to be made at the requirements stage is whether systems have to be ‘explainable’, LaPointe notes. In an explainable system, engineers can understand the reasoning that leads to decisions or predictions, while a learning-based AI system that is not explainable is essentially a ‘black box’ whose reasoning cannot be followed.
“In some cases they might have to be explainable; in others they might not. That makes a really big difference, and you have to understand that before you start to design an autonomous system. It’s an important feedback loop.”
In LaPointe’s view, there is no way to design out all the risk from an AI-enabled system, so we need tools for use with operational systems, such as monitors and governors. What she calls ecosystem management tools with much broader application are also important, she stresses, because it is not sufficient to consider the safety of individual autonomous systems in isolation.
“We are looking to a future when there will be many different autonomous systems, including cyber-physical systems and vehicles, plus many AI-enabled decision algorithms running transportation grids and other critical infrastructure,” she says. “So we spend a lot of time thinking about how the entire ecosystem needs to involve in concert with the individual systems so that we get to the point where autonomous systems are trustworthy contributors to society.”
Ethical AI
When any AI or autonomous system has to make decisions that affect lives, ethical issues arise. LaPointe spent much of the last 10 years of her US Navy career in various technical and policy roles in Washington DC that were concerned with introducing more autonomy and AI into the US fleet. During that time she pushed for the inclusion of ethics into the service’s approach to the technology as early as possible, and now says she is heartened by what she sees as the widespread understanding of the importance of ethics to technology.
“For a while, there was a tendency to assume that if an algorithm is doing something it must be fair, but the fact is that there are always values built into technology,” she says. “You can be intentional about it or you can be unintentional. The latter is the worst-case scenario, because you don’t even understand what you are building in.”
Different approaches to learning what it means to incorporate ethics into AI have been taken over the past 5 years or so, she says, resulting in the emergence of development frameworks from the US Department of Defense and many other organisations.
“What is really interesting when you dig into those frameworks is that most are not about the specific values to be built into the systems so much as they are about the engineering principles that you need to follow in order to achieve ethical outcomes,” she says.
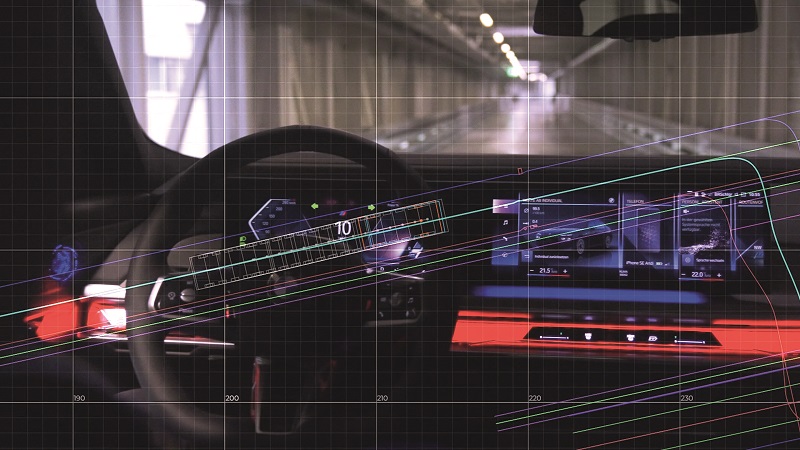
Looking for bias
Over the past year, LaPointe and her colleagues at Johns Hopkins APL and the university itself have been looking into creating ethical AI tools, building on earlier work by the IAA. The main areas of focus are how to eliminate or mitigate bias in the systems and the underlying datasets.
“Any learning-based AI is a reflection of the data that went into it,” she says. “We have had teams looking at the training datasets going into AI algorithms so that we can understand where the biases exist. There are all kinds of bias, but in an ethics framework we are talking about undesirable types of bias that lead to a lack of fairness.
“So we have teams looking at the types of bias that we don’t want the systems to reflect, at how to integrate architectures within the data to show whether the dataset is fair, or ‘balanced’, and at how to create balanced datasets proactively and use them to train algorithms. Our team has applied this to images to identify facial recognition biases and to health data to see if there is age or gender bias in healthcare decisions, among many other things, but it is also a generalisable tool that you can apply to other types of application, even vehicles.”
It is unlikely that an AI running an underwater vehicle, for example, would have much opportunity to exhibit sexism or racism, intentionally or unintentionally, but a facial recognition algorithm might.
“Concerns about what is going to be ethical in use can vary widely,” LaPointe says. “When discussing vehicle systems, we often hear people going straight to the ‘trolley problem’ of how the system is going to decide what to run into. But way before you get to that, you need to make sure you have a system that is robust, safe and reliable, and can perceive the environment well enough to get to the point of making ethical decisions. There is a whole layer of technical systems engineering that we need to do first.”
One important part of that is assessing the uncertainty of how well an AI-based autonomous system is working. This is a systems engineering problem that has an impact on the ethics of operating and deploying such systems, she says.
“When you’re talking about systems that could be life-critical, such as moving vehicles, you need to know what your safe operating envelopes are. It is really important to be able to assess, in real time and in situ, how confident that AI system is in its assessment of its situation, so we have done a lot of work on how better to calibrate self-assessments of uncertainty in AI. There are a lot of different pieces that go into that, including data uncertainty, model uncertainty and distributional shift.”
Distributional shift deals with the transferability of training data between environments. “Let’s say I train my system in the Caribbean and then deploy it in the Arctic: will it go wrong because I trained it on a completely different dataset? That is an example of how you look at the applicability and appropriateness of your training datasets and your models for the situations you are putting these systems into,” she says.
The IAA works with autonomous systems developers and researchers in Johns Hopkins University and beyond in an effort to build an understanding of assurance.
“People often don’t recognise that they are doing assured autonomy, even if they are in the autonomy space,” LaPointe notes. “But they really are doing assured autonomy because the whole process of making sure their systems work correctly is the assurance. So we work with folks to understand what they are having a hard time with, and that is where we set our priorities in creating tools and methodologies to help them.”
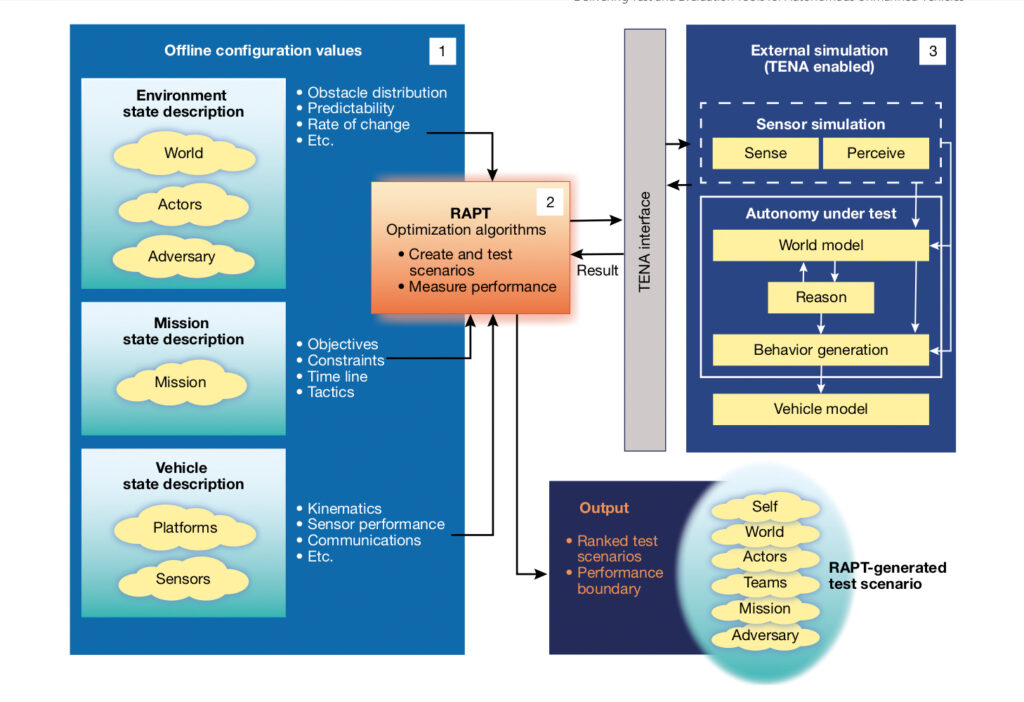
Safety testing
Tools developed by the APL and other organisations are already available and in use. For example, the APL and the US government have developed the Range Adversarial Planning Tool (RAPT), a software framework that allows test engineers to identify safe operating envelopes for decision-making systems in simulated environments.
With a deterministic system it is straightforward to put it in a particular mode to see whether it does what it is expected to do, LaPointe explains, but that does not suit AI operating in conditions of high uncertainty. “When you are testing an autonomous system at the ultimate mission or goal level you need a completely different type of tool to evaluate its performance,” she says.
The RAPT focuses on finding tests that represent the thresholds of, for example, a UUV’s performance in scenarios that are known to demonstrate relevant changes in its behaviour. The tool identifies performance boundaries of the system under test through unsupervised learning methods, and it has been used to test the compliance of USVs with collision regulations, for example.
The IAA has funded an enhancement to the RAPT in the form of a GUI. It helps engineers quickly visualise where changes to an autonomous system’s software has improved its performance and where it has not, LaPointe says.
The IAA’s involvement with tools for developers is broadening. For example, it is working towards presenting what LaPointe describes as a life cycle-based roadmap of tools from multiple sources for project phases from requirements development through design and beyond, which have wide applicability.
“It is important to have tools that are generalisable and, ultimately, tailorable, because every technology and application is going to have different needs,” she notes.”
Ultimately, LaPointe wants the benefits of assured autonomy to help as many people as possible. “When I worked in the office of the Deputy Assistant Secretary of the Navy for Unmanned Systems, one of our colleagues was legally blind, so he couldn’t drive. For him, it was a personal issue to get assured autonomous cars on the road so that he could have a level of individual freedom and mobility. I think a lot about marginalised and vulnerable populations, and I am very focused on ensuring that those systems work for every segment of society.”
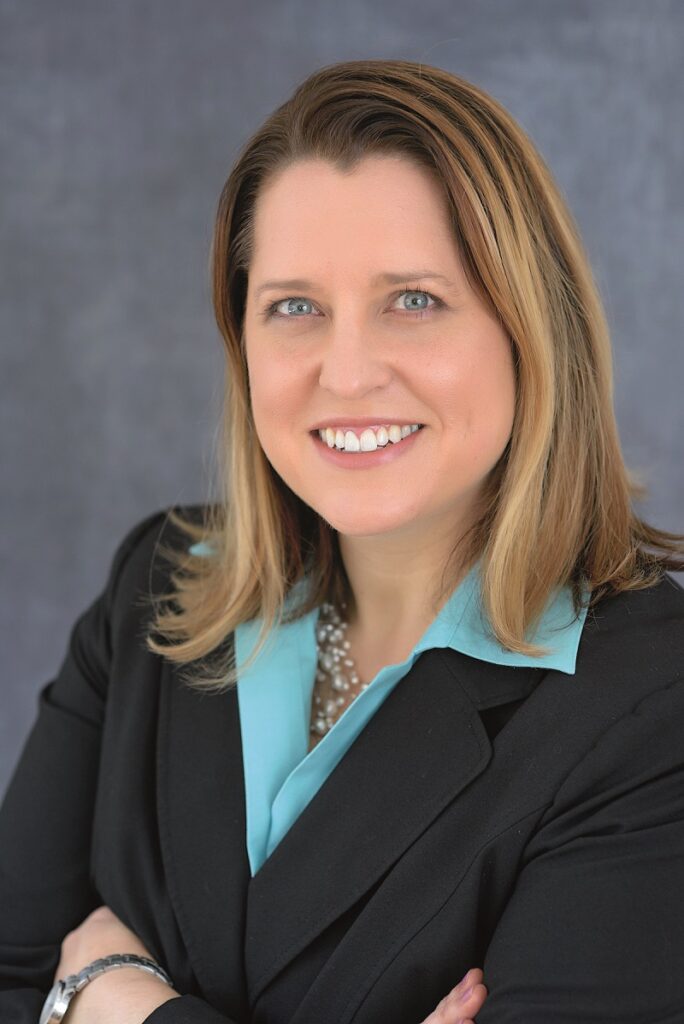
Cara LaPointe
Born in Washington DC to a multi-generational naval family, Dr Cara LaPointe’s love of the ocean drew her into scuba diving in her early teens.
That in turn drew her into the US Naval Academy, where she earned a bachelor’s degree in ocean engineering in 1997. She went on to earn a master’s in international development studies from the University of Oxford in 1999, followed by service at sea in engineering and navigation roles aboard destroyers in the Pacific Ocean.
From 2003 to 2009 she was at the Massachusetts Institute of Technology, where she gained a master’s in ocean systems management, an engineer’s degree in naval construction and engineering, and a PhD in mechanical and oceanographic engineering jointly awarded by MIT and the Woods Hole Oceanographic Institution (WHOI). In the WHOI’s Deep Submergence Lab, she researched autonomous underwater vehicle navigation and sensor fusion algorithms.
In 2009 she took up the post of a ship concept manager for the Navy, working on design, shipbuilding industrial base analysis and the shipboard integration of uncrewed and autonomous systems. In 2011, she joined the Littoral Combat Ship programme, first as a production engineer and then rising to deputy technical director of the programme.
Other senior Navy posts followed, including deputy program manager for electric ships and chief of staff to the Deputy Assistant Secretary of the Navy for Unmanned Systems.
Dr LaPointe founded emerging technology and strategy consulting firm Archytas in 2018, and remains its CEO. She took up the position of co-director of the Johns Hopkins Institute for Assured Autonomy and Intelligent Autonomous Systems programme manager at its Applied Physics Laboratory in January 2019.
UPCOMING EVENTS
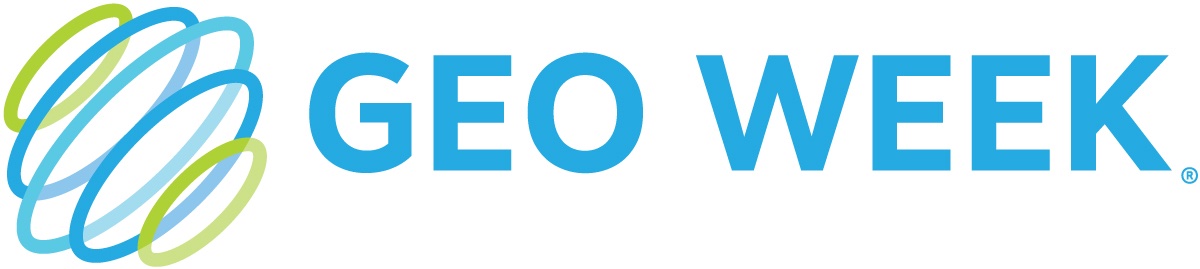
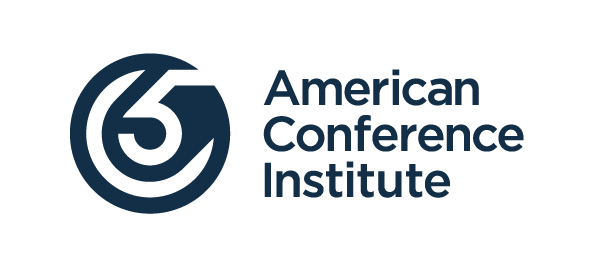
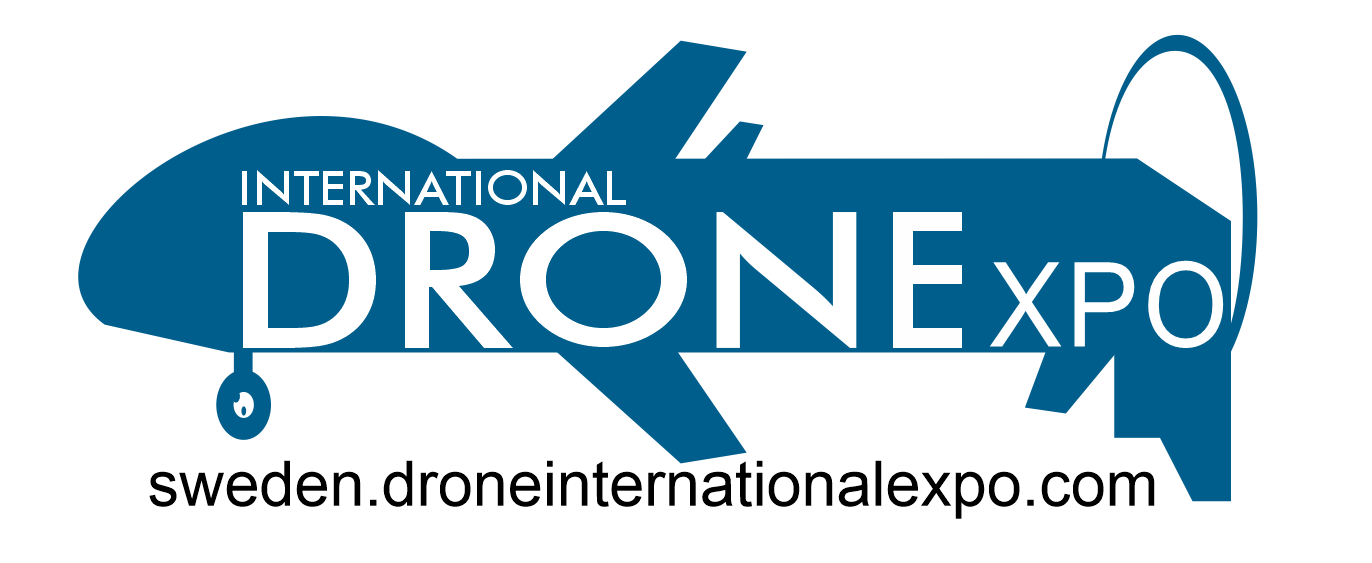
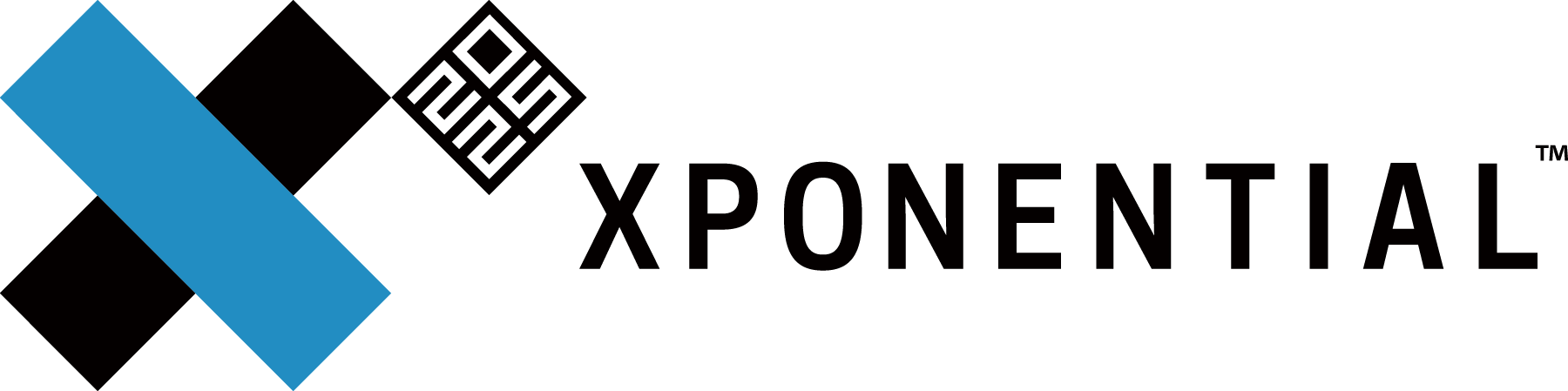
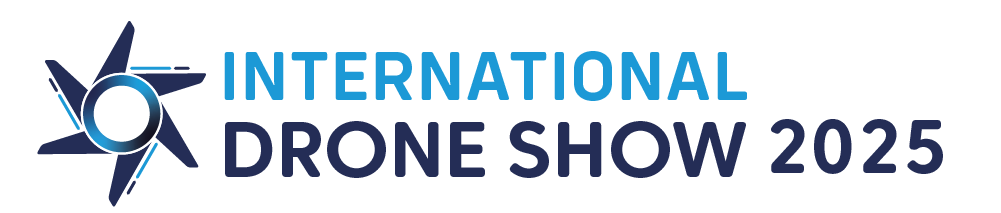