Avionics design uses ML
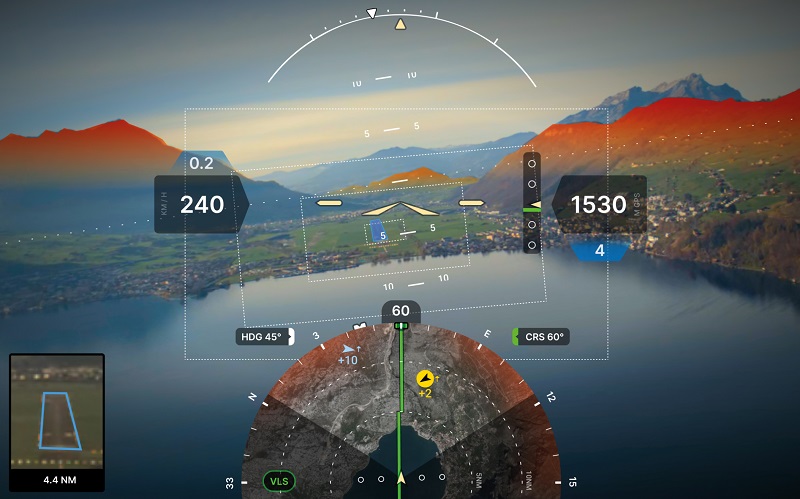
Intel and Daedalean have developed the first multi-core reference design for certifiable avionics using machine learning, or ML writes Nick Flaherty.
The design provides vision-based situational awareness using neural networks with high-resolution, high-throughput camera inputs based on Intel’s 11th Gen Core i7 and Agilex F-Series FPGAs.
On top of the SWaP constraints, autonomous aircraft developers face two other challenging circumstances when incorporating ML and AI into avionics systems.
First, ML and neural network applications have increasingly high computational requirements. Second, no ML application has yet been certified by aviation regulators.
The problem is that processor manufacturers typically withhold details about how a multi-core processor’s shared cache works, such as how cache lines are flushed, despite the common understanding that cache operation has a major impact on the determinism of how applications run.
Intel has already introduced the Airworthiness Evidence Package (AEP) that provides manufacturers with processor artefacts and the analysis and mitigation of non-deterministic and unintended behaviour to support DO-254 certification up to design assurance level (DAL) A for aircraft.
Now Daedalean has designed a system for a sensor computer using the AEP and Intel processors for use in AI/ML aviation applications and with the available documentation to support certification.
This is the first real-world working example to provide guidance on how to approach these challenges in general: how to ensure an ML-based system can meet the computational requirements, certification requirements and SWaP limitations at the same time
Without understanding the behaviour of multi-core processors, system developers can struggle to guarantee mitigation of all potential failure conditions. This is currently solved by disabling three cores in a four-core system for certification, because the interaction between the cores cannot be sufficiently managed for the certifying authorities.
Daedalean’s AI-enhanced situational awareness software provides certifiable ML in safety-critical aerospace applications with a full multi-core system. Careful partitioning of the hardware allows all the software components to be tested independently without interference.
While partitioning all resources is not necessary for certification up to DAL-C, Daedalean chooses to conduct partitioning for two reasons – for the potential to upgrade to DAL-B in the future and to simplify the testing process.
The Daedalean system runs on Vyper, a lightweight hypervisor developed in-house. For each software component, it determines what it is allowed to execute and when.
Vyper’s minimal partitioning hypervisor runs on small code size, typically 1500 lines, making it easier to certify, and runs multiple isolated partitions with each partition statically assigned to one of four physical CPU cores.
Memory management is a key issue for such systems, and a two-level paging architecture provides each partition with its own isolated address space, allowing memory buffers to be safely shared between partitions.
Daedalean does not need to use an RTOS in its system, because the virtual machine extensions are designed to be lightweight and self-contained without relying on third-party code.
That allows the system to operate without the need for insulation from external sources. However, developers using an RTOS or commercial hypervisor can still run Daedalean software, provided that the RTOS supports the 11th Gen Intel Core i7 processor.
That allows developer applications to run on the architecture, as Daedalean reserves CPU cores for this purpose.
Aviation guidance from the CAST 32A standard and the more recent AMC 20-193 adopted in Europe recommends – and in some cases mandates – that all available resources are partitioned among applications to ensure predictable execution and prevent competition for access.
In the case of a system with four cores, Vyper provides robust partitioning of CPU time, cache levels, system memory and device access by partitioning the cores into non-interfering time slices. It uses Intel’s Time Coordinated Computing settings in the processor BIOS to make execution more deterministic, and is coupled with VMX Virtual Machine Extensions to create virtual machines that run the partitions. Virtualization for Directed I/O isolates the PCIe devices and assigns them to partitions.
Intel’s Resource Director Technology Framework, particularly Cache Allocation Technology, is used to partition the shared Level 3 cache memory. This allows each partition to have its own cache and memory, and the only primitive used is a FIFO for comms, which is set up at compile time. That allows the system to operate without interrupts.
Vyper runs a scheduler on each processor to execute software partitions according to a compile time-defined schedule.
To simplify certification, there is no dedicated processor for handling partition hypercalls or managing the overall system state. Instead, the schedule on each processor is responsible for all partitions pinned to that processor. Software partitions can run either application logic or driver code; this distinction is transparent to Vyper.
The first implementations are supporting pilots in the cockpit with situational awareness but can scale for fully autonomous systems that are certified as safe to carry passengers.
UPCOMING EVENTS
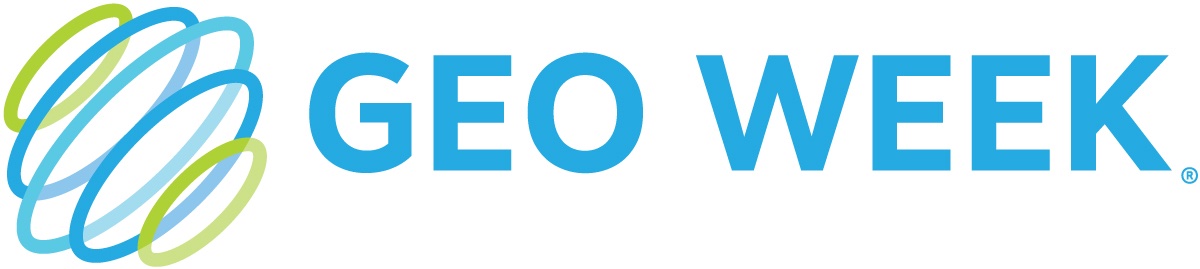
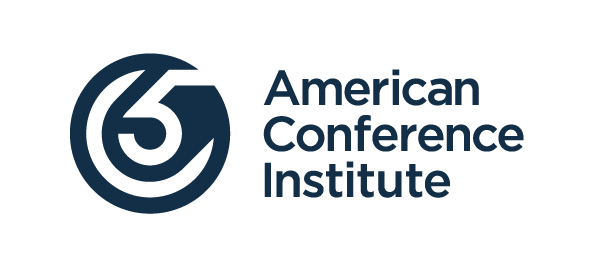
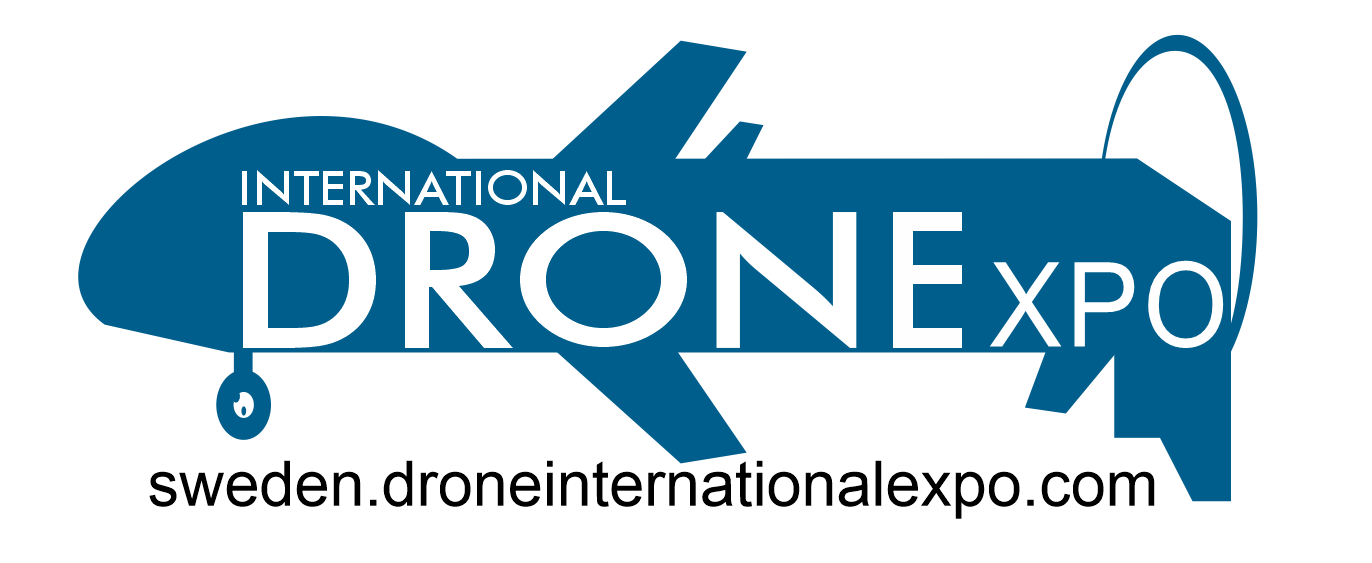
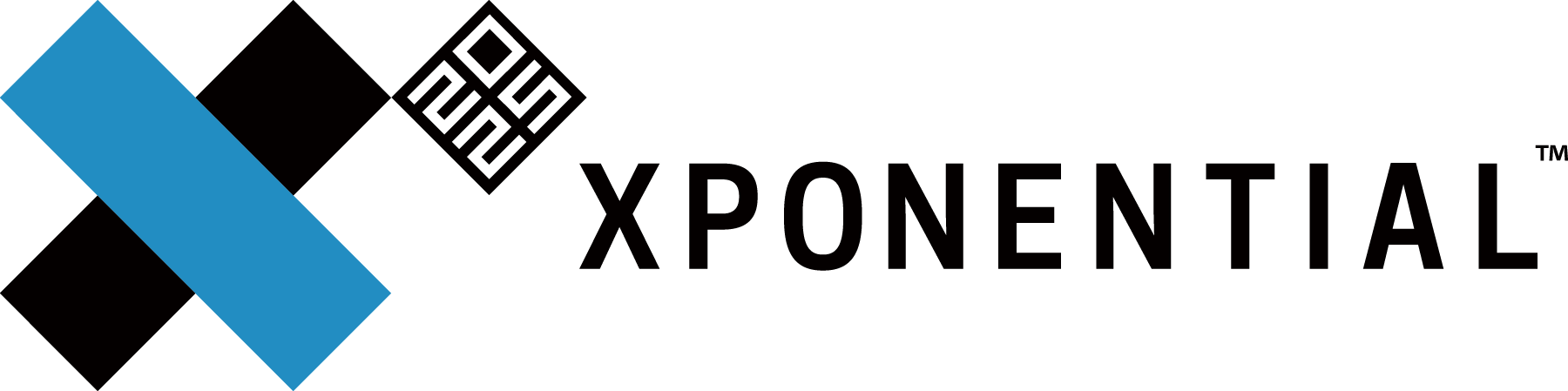
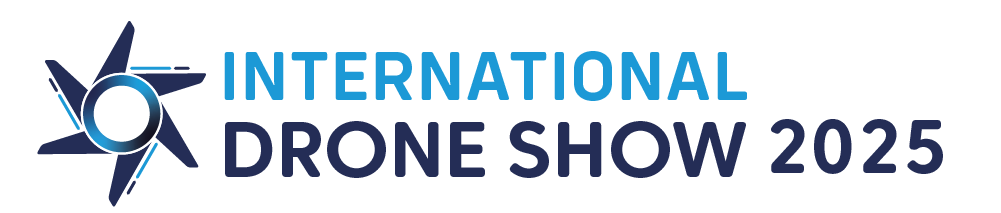