Kodiak Driver
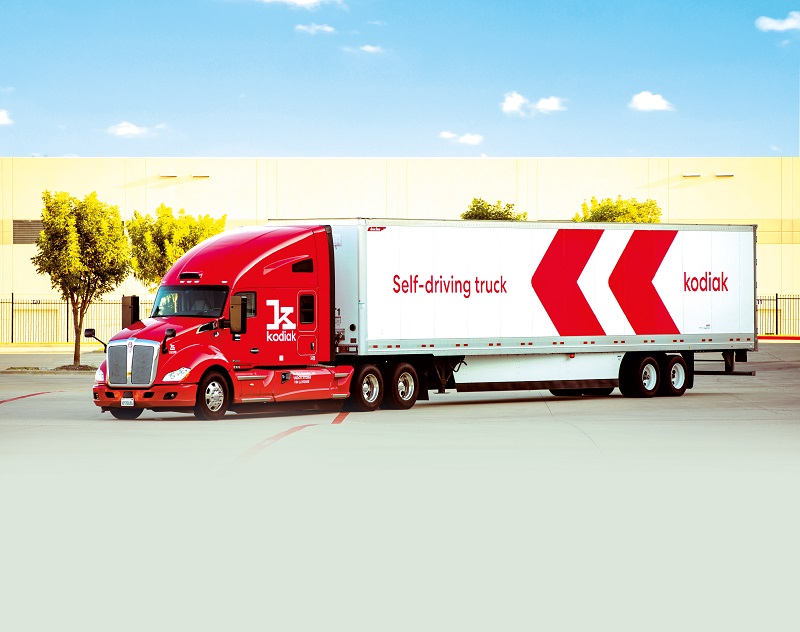
Self-driving ambition
Autonomous trucks could solve many of the growing problems in the US logistics industry. Rory Jackson reports on this prime example
Road freight is vital to the US economy. The industry is worth more than $800 billion, and readers in the US can safely assume that two-thirds of the objects around them were transported at some point in a Class 8 truck such as the archetypical five-axle 18-wheeler.
The problem though is that the US logistics sector faces a dire shortage of truck drivers, owing partly to its onerous training and licencing requirements, as well as what to many are the unattractive conditions and terms of the work. As a result, the country’s logistics industry was roughly 80,000 truck drivers shy of its optimal worker count in 2021, and as more and more older drivers retire without anyone to replace them, that figure is expected to grow to 160,000 by 2030.
However, autonomous trucking would allow freight workers to limit their journeys to local logistics centres, truck transfer hubs and remote monitoring stations, instead of enduring countless uncomfortable driving hours, sleeping in or above the cab, and so on.
And without drivers needing to sleep and recuperate between their 11-hour driving stints, self-driving cargo trucks could operate 24/7, with only short intermittent stops for refuelling, tyre changes and other maintenance. The growing demands on the logistics industry for freight and better working conditions could therefore be addressed entirely through autonomy.
Such benefits have been recognised by the industry for some time, and as a result there are now some major players achieving success in autonomous trucking. Kodiak Robotics in particular is led by a number of experts in the field, many of whom cut their teeth on the Google self-driving car project (better known these days as Waymo), including its CTO Andreas Wendel, who has worked on autonomous driving for more than 10 years.
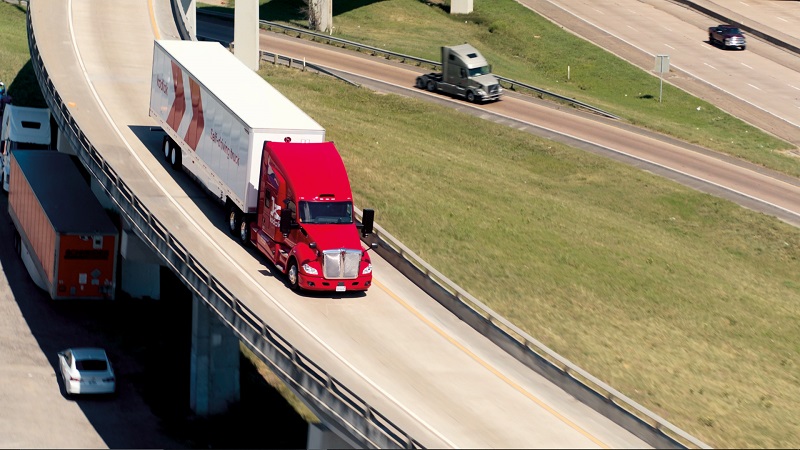
Why self-driving trucks work
“I was there with our now-CEO Don Burnette, and it became clear to us early on that autonomous driving is very solvable, and you can engineer it to be as safe as you want – but the product case is much more complicated,” Wendel says.
“With robotaxis for example, the bigger challenge was getting dropped exactly where you want and having the right arrival times, and that sort of thing is still very tricky. We have no doubt it will happen, but it takes time to make a well-rounded product out of that, as well as any autonomous road system working in urban environments.”
Wendel explains though that if big trucks avoid urban traffic by using logistics hubs, truck transfer centres and gas stations outside cities, they can be kept to a controlled set of operating conditions, analogous to how robotaxis are often trialled in university campuses and business parks.
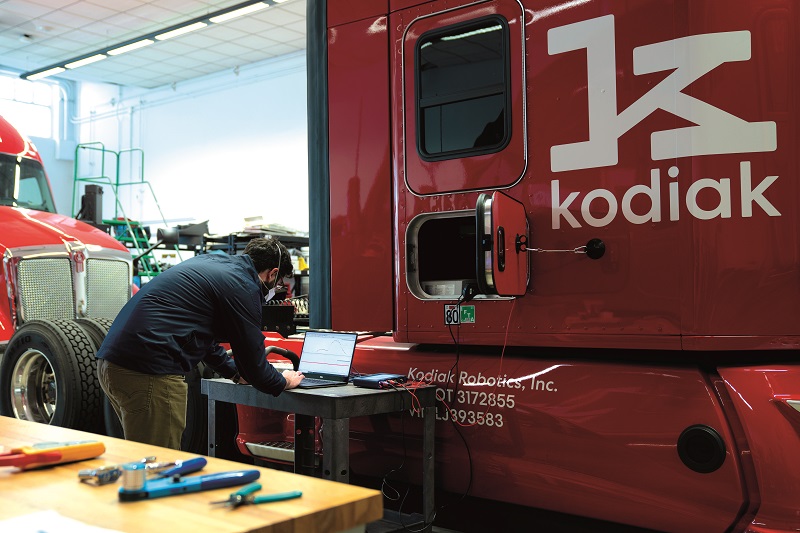
“Don left Google in 2016 to co-found Otto, one of the earliest autonomous trucking companies, but that was acquired by Uber and he was forced to pivot back towards passenger vehicles,” Wendel says.
In April 2018, Burnette left Uber to co-found Kodiak Robotics, with Wendel joining 3 weeks later at Burnette’s invitation. “I was the perception technology lead at Waymo, but through Don I’d learned that via trucking, we could get to a real product much faster, and fulfil the enormous demand for autonomy across logistics.”
Kodiak’s fourth-generation truck represents that product. It is now highly matured technologically and tapped for use by IKEA, CEVA Logistics, US Xpress, Werner Enterprises and numerous other companies seeking solutions to their trucking problems through something buildable, scalable and maintainable by their technicians.
Early r&d
Kodiak started from a blank sheet, bringing together engineers from Waymo, Apple, Uber, Lyft and other technology majors to ensure the concept phases were structured and executed pragmatically.
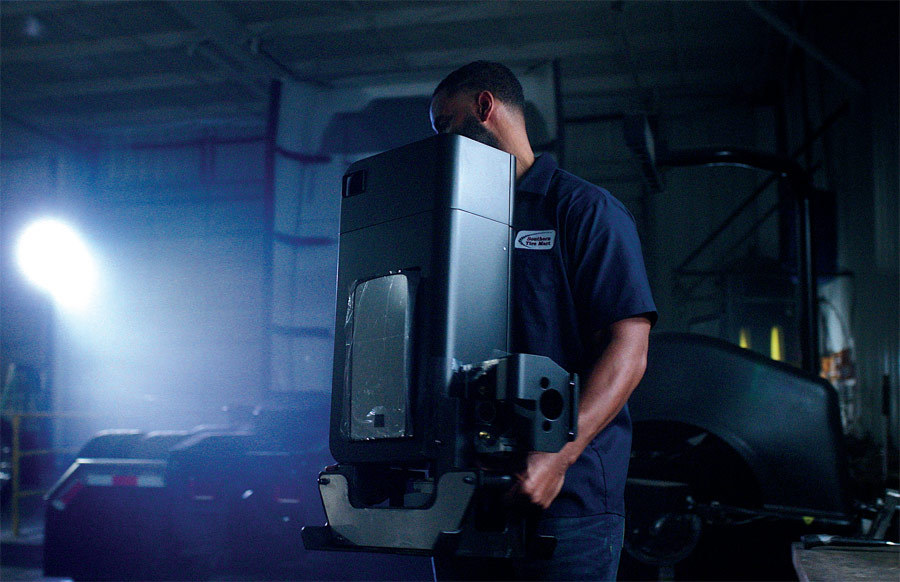
“We didn’t have any Class 8 truck drivers though, let alone anywhere to store and work on a Class 8 truck, so we acquired a little box truck, equipped it with sensors and used that to develop the first build of our hardware and software stack,” Wendel says.
“We also talked to several leading truck OEMs, and soon reached an early development agreement with PACCAR for our first Class 8s in December 2018. The model used as our platform is therefore a Kenworth T680, Kenworth being a PACCAR company.”
By then, Kodiak’s first generation of hardware and software had been completed through virtual simulations and bench testing, ISO 26262 providing a crucial baseline for component validation tests, with aspects of other standards overlaid on that baseline where prudent.
Key to that was Applied Intuition, which built the essential simulation tools Kodiak uses in development, and Scale AI, which carried out data labelling and annotation to help with Kodiak’s testing.
Over the following 2 weeks in December 2018, Kodiak’s engineers installed their systems onto the truck. After that they ran the truck autonomously in their parking lot, first evaluating safety-critical benchmarks such as the truck’s ability to cede control to a driver in the event of an emergency (which Wendel confirms proved successful).
“Then, in July 2019, we managed a test case of our first autonomous commercial delivery, and since it was in Texas, we opened our first real office there, where we’ve kept making commercial deliveries to learn from our customers and inform our r&d,” Wendel explains.
“In December 2020, we did our first 230-mile, disengage-free commercial delivery. Technically we did four of those in a row, so it was more like 920 miles on the freeway, without the in-cabin safety driver taking over except when leaving the freeway exit ramps to enter delivery hubs. There’s a video online showing this – it’s obviously very long, but it shows every step of what we achieved.”
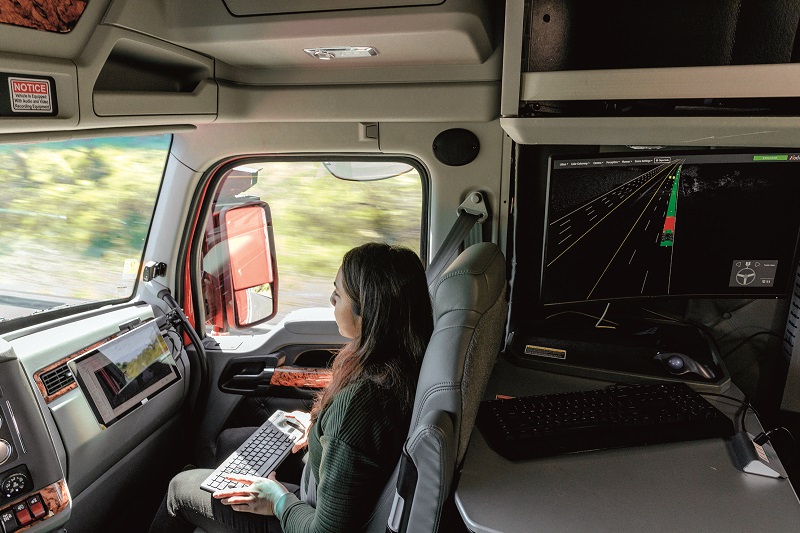
Kodiak Driver system
Kodiak tested its truck without a safety driver on a closed test track for the first time in August 2021, and in the following month unveiled its fourth-generation truck, which the company calls the Gen4. Wendel notes here that generation-centric nomenclature belies quite a fluid development philosophy, which he illustrates by pointing out that the r&d for the fifth-gen truck began only weeks after the fourth-generation’s tyres touched a public road.
“The Gen4 carries what we feel is the industry’s most modular and discrete sensor suite, vastly simplifying sensor installation and maintenance compared with any other autonomous truck – and most autonomous road vehicles – and increasing safety via a plethora of sensor redundancies,” he explains.
The sensor suite is contained mostly within structures at the side mirrors of the cockpit, with an additional and largely conformal sensor pod on top of the roof, although Kodiak can already disclose that the Gen5 will do away with the pod. Instead, everything perception-critical will be integrated into the mirror structures, and as of the Gen4 the mirrors can be swapped in and out very quickly.
That level of modularity contributes directly to fleet uptime, a crucial metric for Kodiak’s customers, as discovered through feedback on the truck post-delivery. Having achieved that, the company says all future Kodiak truck generations will look nearly identical to the Gen4, regardless of any changes under the hood.
Also, given Kodiak’s business model, the company emphasises that the truck is mainly a carrier of its main product, the Kodiak Driver technology. This consists of the sensors, actuators, processors, data links, algorithms and integration processes that can be applied to any Class 8 truck and other vehicles. It is the metaphorical torch that is passed from one generation of Kodiak truck to the next, and which can be used by any Class 8 fleet operator.
“If we want to offer a tangible, turnkey product to truck fleet managers, there are three ingredients to that. First is the Kodiak Driver, which comprises all our autonomous technologies. It includes map and software updates over the air to ensure our localisation and autonomy systems are kept up to date.
“Then there’s Kodiak OnTime, which is our user-oriented program and API designed to interface with fleet management, logistics and scheduling applications, so that fleet managers can plan their deliveries, their trucks’ maintenance, business reports and so on.
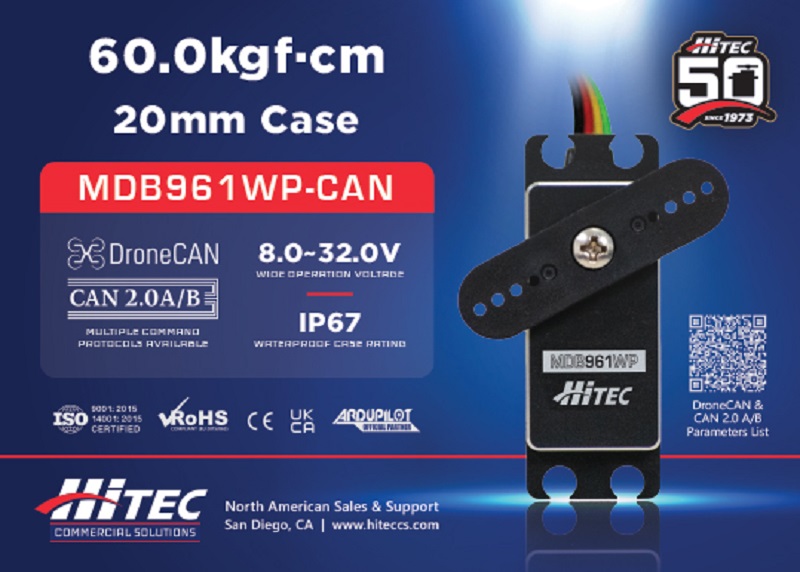
“Third is the Kodiak Network, which is the combination of mapped routes and autonomous truck-capable transfer hubs, as well as the network of truck and AV service providers. The network provides the environment for the Kodiak Driver.”
Truck and Kodiak Driver
As discussed, most of the sensors in the Gen4 and Kodiak Driver are housed in the cockpit’s wing mirror structures and a frontal roof-mounted structure, referred to as the SensorPods and the CenterPod respectively.
Each SensorPod contains two radars, three cameras and a Lidar. The radars and cameras together provide 360o of awareness, while the Lidar provides side and rear perception. The CenterPod contains a forward-looking Lidar and camera for perceiving and reacting to objects and events in the direction of travel.
Data from these feed into the Kodiak Driver’s main computer, in the back of the Gen4’s sleeper cab. This was part of the original Kenworth T680 on which the Gen4 is based, and contained a bed for the driver for overnight stays. It has now been removed though in order to store the autonomy stack’s computer hardware; also located here are two seats principally for seating customers during demonstrations.
An IMU provides motion reference data, along with a GNSS receiver and antennas for additional position and heading information. The main computer runs the autonomy software to map the truck’s environment, localising itself, calculating its optimal next routes and sending control outputs to its actuator ECUs. Kodiak calls these two electronic controllers its ACEs (actuation control engines).
“The ACEs, including the actuators they link to, are highly redundant systems, to the extent that we’re working on certifying them to ASIL-D,” Wendel explains.
“They interface with the main computer directly over CAN bus as well as some GPIO ports – we work with various Tier1 suppliers for our actuators – so they take care of our proprietary steering and braking processes. Drive-by-wire systems are not standard for Class 8 trucks yet, so we put in actuators that can communicate electronically with the ACEs.”
NVH and ruggedness
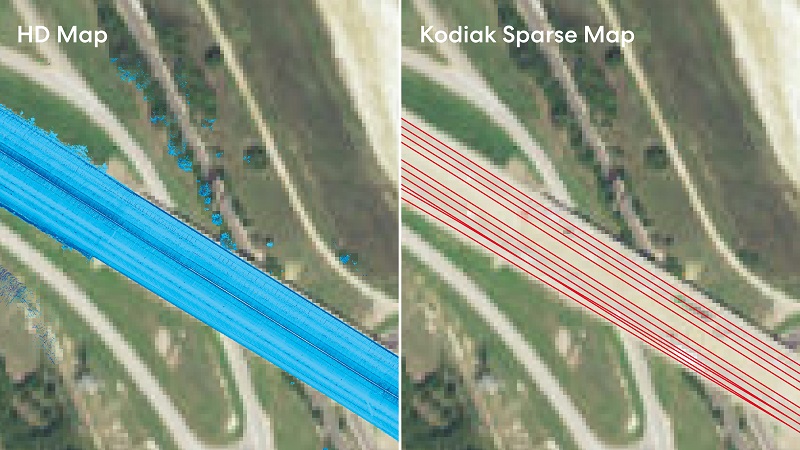
While passenger cars are generally built to be driven for 100,000-200,000 miles, Class 8 trucks are meant to last for a million, and to do so while enduring harsh weather and persistently severe noise and vibration.
Kodiak’s hardware therefore had to be capable of enduring such conditions before it could be entrusted to fulfil any of its end-users’ other requirements, or it would not give the mileages and lifetimes fleet operators would want.
“Many AV [autonomous vehicle] companies these days just take computer and sensor hardware they’ve designed for passenger vehicles, and stick it on a truck, but it doesn’t last,” Wendel says.
“We’ve focused on trucking from the beginning, so our hardware is built and enclosed to fit the trucking ecosystem, and to endure things like your 80,000 lb truck hitting a pothole. We found from the start of our testing that some of our sensors couldn’t actually survive a shock like that, so through trials and working with our partners we’ve gone all out to harden them against NVH-related failures.”
To ensure their testing methods truly represented trucking conditions, Kodiak’s engineers first selected different testing standards for trucks, passenger cars and Mil-Spec vehicles. They then cross-referenced them with vibration profiles captured from their own testing routes to determine the kind of vibration profile they ought to set their shaker tables to.
Through in-house testing on those tables, all of Kodiak’s electronics have their enclosures and connections redesigned and remanufactured until the internal components’ survivability over a minimum lifetime of NVH is confirmed.
“As for weather, people think heavy rain or maybe light flooding are the worst wet conditions that road trucks will be subjected to, but it’s actually the pressure washing that happens during routine maintenance,” Wendel muses. “The power of those hoses is so much worse than any rain, and that set the bar for the ingress protection we needed.”
Driving safety
While the truck’s AI is equipped to obey traffic laws and react appropriately to road signs, traffic lights and pedestrians, the initial trials, deliveries and roll-outs of the Kodiak Driver are being kept to freeways, away from populated areas. That has cut the amount of work Kodiak has had to do on the dangers typical of city environments.
As Wendel reiterates, “Kodiak’s current operational design domain is limited to access-controlled highways like the US Interstate Highway System, where pedestrians and cyclists are not allowed. Still, our system regularly observes any pedestrians on those highways – mostly on the roads’ shoulders and, rarely, in the road – and responds safely by slowing or changing lane.”
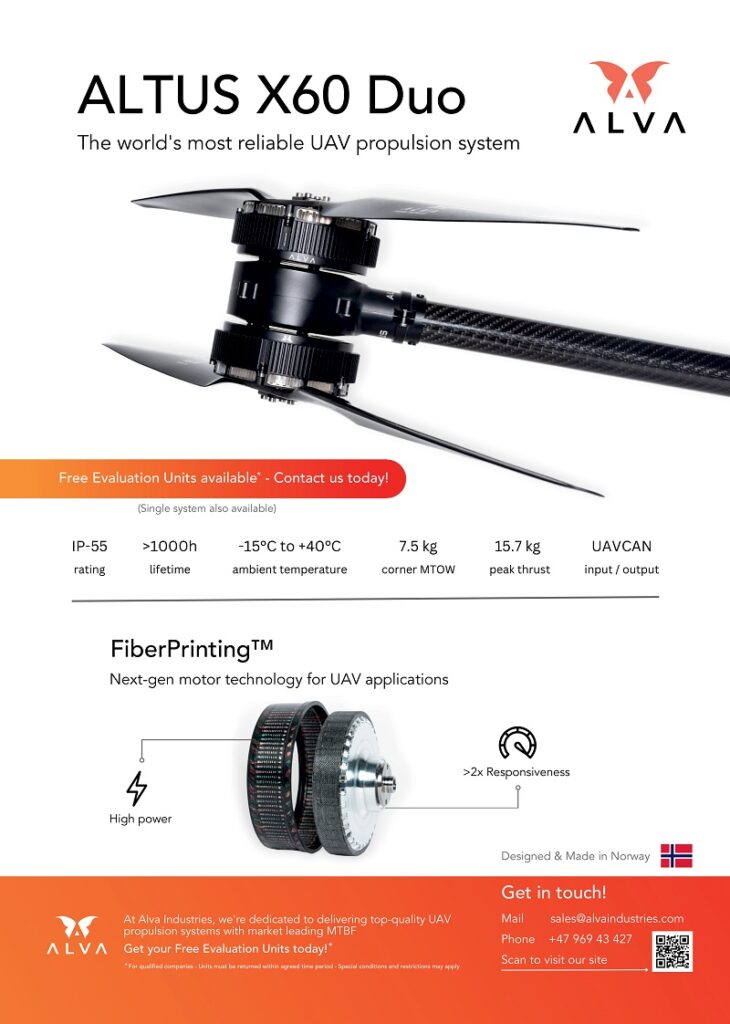
Within the limitations of their cross-country freeway routes, the ACEs and actuators are engineered such that the truck can always come to a safe stop in the worst-case scenario – such as an imminent traffic incident or a detected subsystem failure – otherwise they ensure that the truck can continue following its optimal route.
“In that respect, it’s worth noting that we are the first company to show that we can execute what we call a ‘fallback manoeuvre’ on highways, also called a minimum-risk condition,” Wendel says.
“Basically, 10 times per second, our system checks more than 1000 fault signals, including engine coolant temperature, tyre pressures, camera data validities and the main computer’s execution timings.
“That means we can constantly and rapidly generate new routes, not just for how we can follow our preferred path down the road but also for situations where we can’t continue with those plans and their general objective. That could be through a main computer failure or a check engine light, say, and how we can get the truck to safety, potentially without multiple key subsystems.
“That usually means an autonomy pull-over trajectory onto the shoulder. In one test we videoed, an engineer actually severed a cable inside the truck, and the Kodiak Driver immediately realised what had happened and pulled over until both its tractor and trailer were out of harm’s way.”
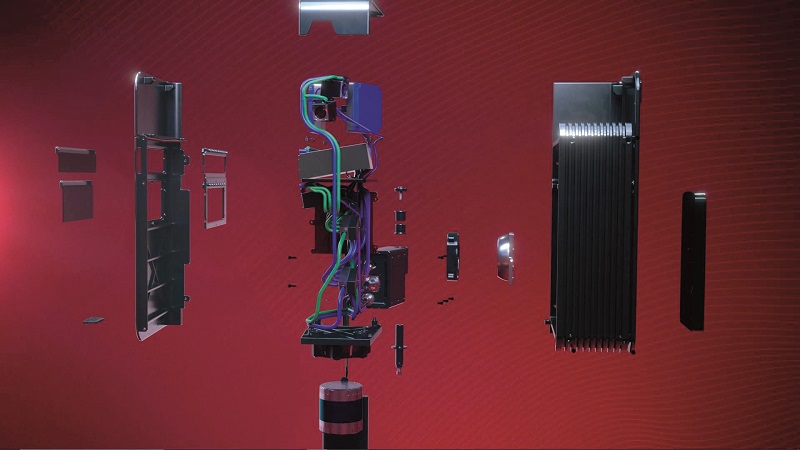
Sparse Maps
Notably, the Kodiak Driver does not use RTK-GNSS, and in fact makes little use of GNSS in general, other than an initial reading on where the truck is located upon start-up.
“We start up the truck, and the GNSS antennas and receiver tell us if we’re in California or Texas; it’s only a rough idea of where we are,” Wendel notes. “What really gets us our localisation is a system we call Sparse Maps, or Lightweight Maps, to contrast it with the HD Maps supplied and used in some AVs.
“HD Maps is useful in cities for localising yourself relative to buildings, signs, trees or kerbs next to you, using what is a very fine-grain, usually laser-based representation of the street. But if you try to do that on the highways where trucks operate, most of what you’ll see around you are fields or deserts. There isn’t a lot to localise with, and what little there is can easily change overnight, which HD Maps doesn’t account for.
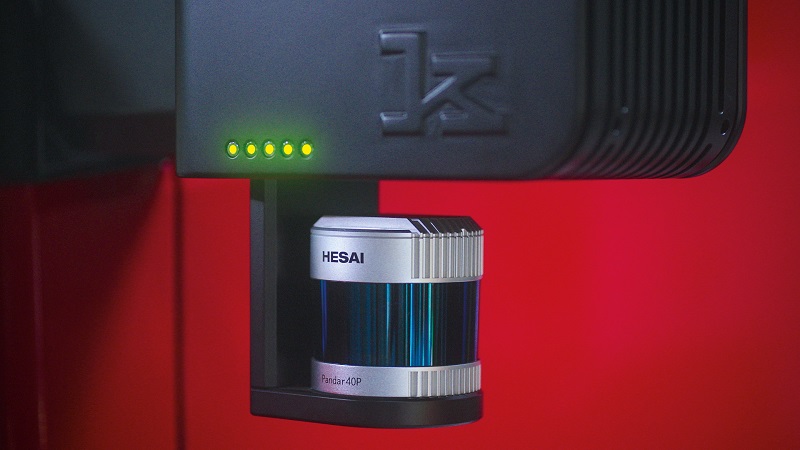
“Moreover, it’s not so important for Class 8 trucks to be driving correctly relative to a tree so much as staying correct relative to the lane markers on the road. That’s what they need to follow.”
Kodiak has accordingly developed Sparse Maps as a lane marker-based mapping and localisation system. After taking their initial GNSS-based fix of which road they’re on (or which logistics hub they’re at) each truck uses its cameras and Lidars to view the lane markers and follow them, with any changes in the road being recognised and submitted via LTE modems for all the other trucks to download, and with all of them using the most recent map of their local lane markers for reference.
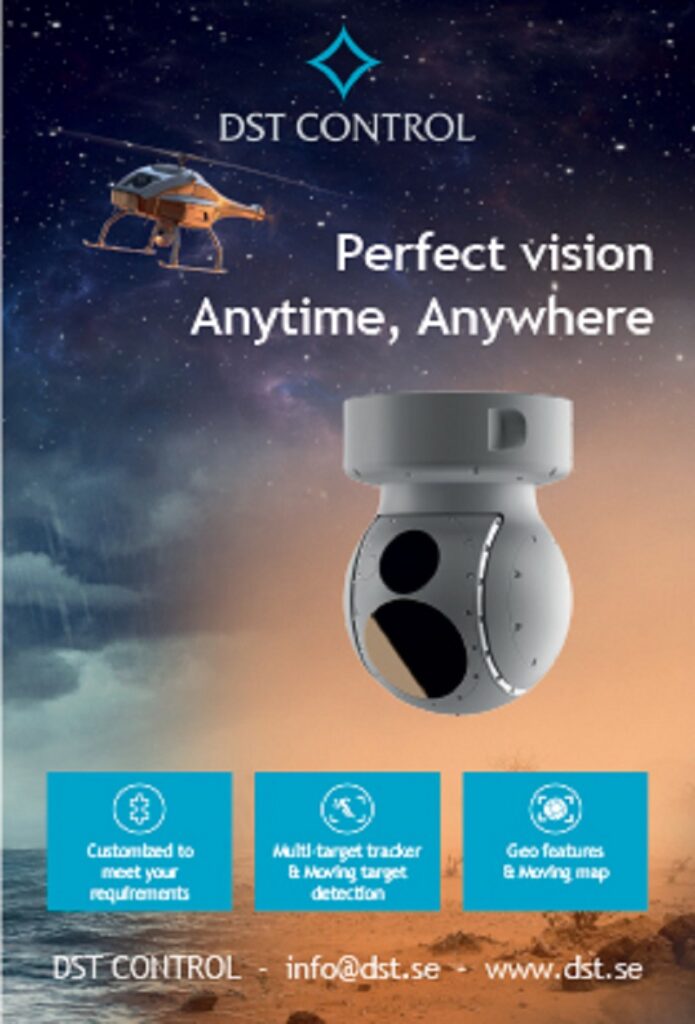
“In the best-case scenario, the truck sees a matching image of whatever the last Kodiak Driver saw,” Wendel explains. “That way, the truck knows the route to follow, where the lane will exit or merge and where it can change lanes. Our topology means it knows about the surrounding roads, just as a human driver does.
“Most of the localisation comes from the truck recognising which lane it’s in, with the sensors also accurately performing odometry. It makes for a very lightweight localisation technique: even with the thousands of miles of road we’ve mapped across the US, it’s a minimal amount of data the trucks need to download, upload and store.
“The Sparse Maps approach has allowed us to develop our maps faster than any other AV company. Our network now stretches from coast to coast, and we’ve done a commercial run over that distance with one of our partners in which we mapped road changes too. That’s a major step towards our commercial ambitions, because we’re able to prep for new delivery routes in 3 weeks, while our customers have been led to expect it taking months.”
The SensorPods
As discussed, the sensor housings are built for ease of maintenance. Kodiak has shown that they can be swapped faster than tyres, with the entire SensorPod being removed and replaced in 9 minutes.
“The main reason it’s so fast is because all our SensorPods and CenterPods come pre-calibrated; if you don’t have the right set-up, calibration is very tricky,” Wendel says. “You need to see a lot of views of a certain target, you need to move the vehicle around, and a truck technician who has never dealt with HD cameras or Lidars can’t be expected to perform calibration quickly or easily. Fleet uptime is everything in trucking, so ensuring we can get each vehicle back on the road in 9 minutes is imperative.”
A pole-and-hinge system mounts the SensorPod housing to the cockpit, and can be customised for a Kenworth T680 or any other Class 8 truck. Also, a single military-grade connector provides the software interface between pod and truck, and liquid lines for air and water from the truck run into the sensor pod to enable automated cleaning of the camera lenses and Lidars.
A total of seven cameras are integrated across the Gen4, three of these being forward-facing, one pointing left, another pointing right, and two looking rearward on each side. The forward cameras are the most important for object detection and localisation, while the side cameras provide a view around the truck.
“The cameras in general can see objects up to 1000 m away, but in practice 300 m is really the sweet spot where we want to see reliably and precisely,” Wendel says. “What’s most important is knowing how far you can see at any time. Say you’re driving in fog: in such conditions, a person can’t see very far, but they can still drive reliably and slow down when appropriate, because they understand that their visibility is reduced and they need to be more cautious.”
This range enables a detailed and deterministic FoV analysis of objects at different ranges. To ensure this analysis bears useful data outputs, Kodiak aims to integrate cameras of at least 3-4 MP, and if supplies of those are short then the company opts for higher resolutions.
Meanwhile, the forward-facing CenterPod camera’s wide-angle lens ensures a 120o FoV and high resolution of close-up objects. The forward-facing cameras on the SensorPods integrate a zoom lens for hi-res vision up to 1000 m down the road with a 30o FoV.
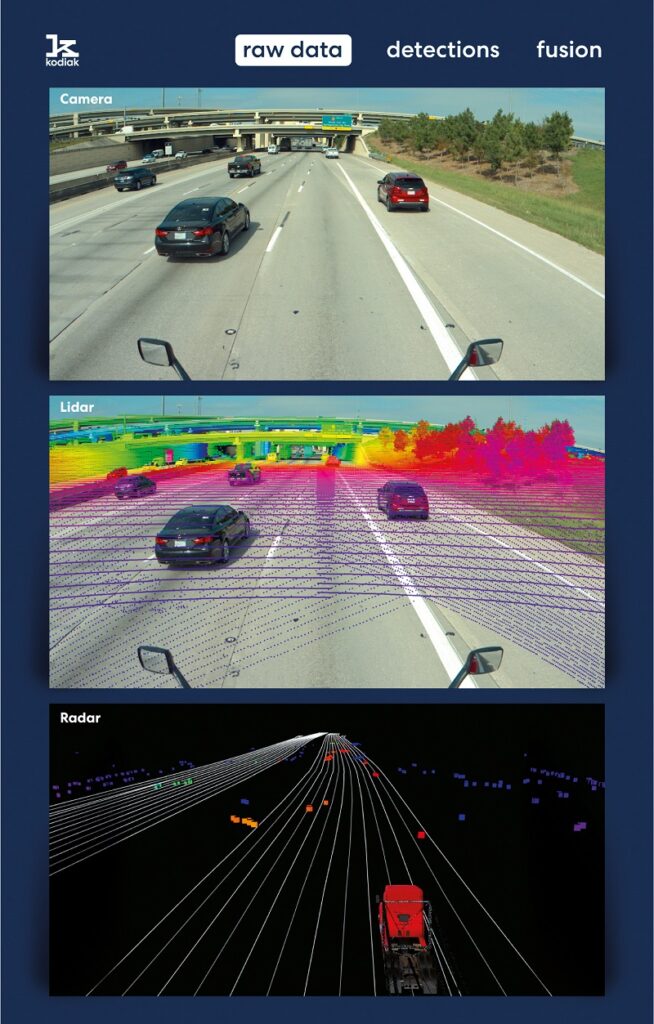
“If I had to describe in one word what autonomous driving means to us though, I would say ‘redundancy’,” Wendel adds. “That’s why, as well as the seven cameras looking about the truck, we also have three Lidars and four radars [two per side].
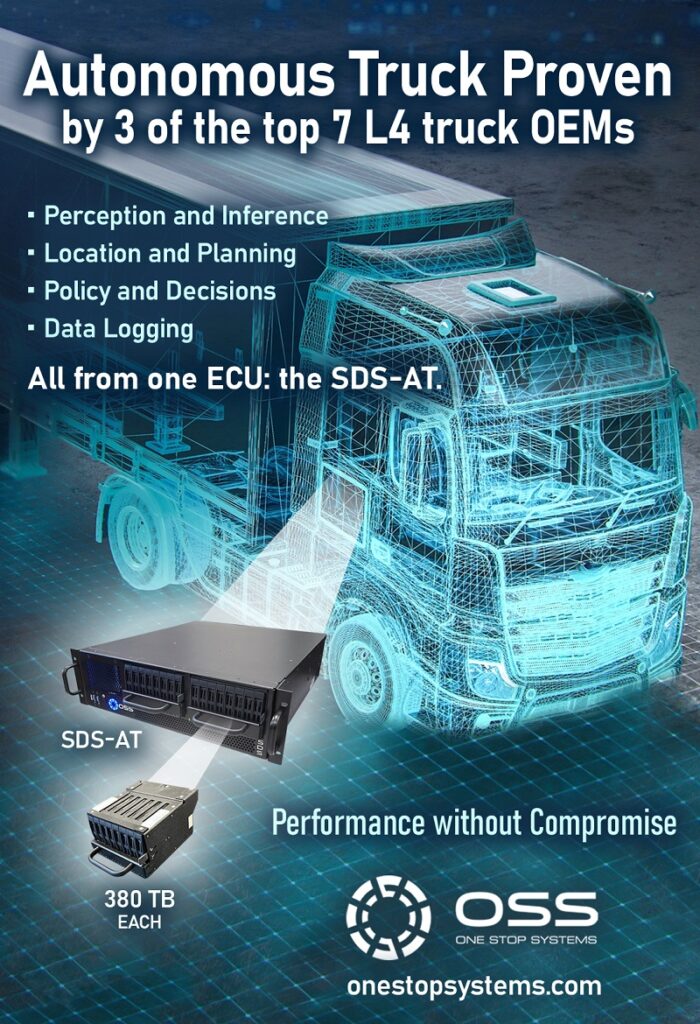
“And rather than having different roles for everything, we’re doing a lot of the same things redundantly, using different sensors with different modalities, so that we can tell if one of them isn’t working properly. That’s what makes an AV safe for use on roads.”
The SensorPods each integrate a Hesai Lidar, while the CenterPod uses a Luminar Lidar. Both companies benefit from the feedback Kodiak accumulates for them through their testing and commercial deliveries.
Wendel lauds the robustness and reliability of both Lidars, saying, “When you look at a laser’s specs, people often ask first how far it can scan, then if there’s another that can see 20 m further, but those don’t make for a robust and reliable AV. ‘Guaranteed range’ does: how far can I fully trust that the real-world objects are reported by the laser and not missed?
“We’ve therefore tested our Lidars extensively to know that these ones will report data with an accuracy and integrity at the 300 m distances imperative to our safe driving and obstacle avoidance.”
The Hesai Lidar models are rotating 360o units, with one either side to cover the blind spots created by the truck. Relying on a single unit on top of the cab could render the truck partially blind to nearby vehicles. In Kodiak’s configuration, any vehicles or motorcyclists next to the truck can be detected by the Hesai units to ensure the truck can avoid excessively risky movements.
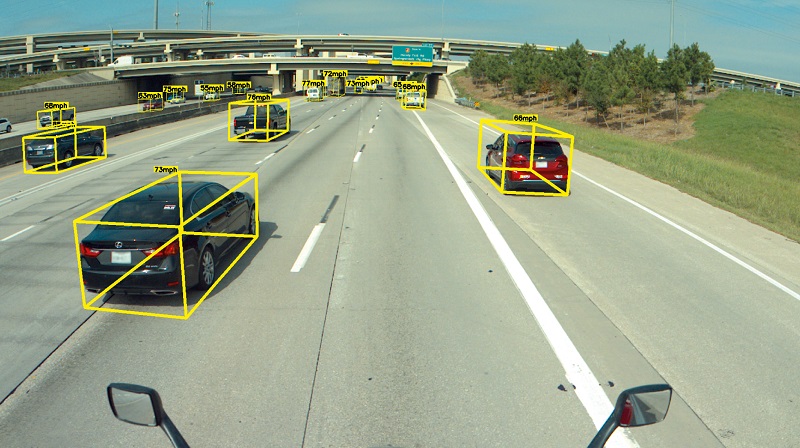
The Luminar Lidar in the CenterPod has a longer range. In the 300 m ahead of the truck, all three Lidars, the three forward-looking cameras and the two forward-facing radars provide overlapping views, ensuring the redundancy of object detection and lane counting in that safety- and localisation-critical ‘cone’.
“The cameras are the first source of data in lane detection, but the Lidars are also important,” Wendel says. “Traffic lanes are highly reflective surfaces, and often even have little reflectors between them that pop out even more to the Lidars. In general, our perception processing software treats all the data equally, so there isn’t really any prioritisation of one data feed over another.
“The radars, from ZF, are key to perception, but not the localisation aspect of it: they are ‘4D’ systems. Most automotive radars have a very flat view of the world, with just 2D distances and velocity, whereas ours also measure elevation over ground.
“That helps us to see a lot of important obstacles that other AVs miss, for instance cars that have stopped under bridges. The radars can tell physically where the car ends and where the bridge begins. Without that distinction, the truck would have to slow down and lose time at every bridge.”
Wendel adds that the radars have an effective range of 300 m as well, and that their ability to discern velocity is particularly critical for ensuring safety at the rear and sides, from where other vehicles might be approaching.
Kodiak Vision
The camera images and Lidar models are run through a deep neural network to extract key properties such as lane markings and road boundaries. These directly inform calculations regarding the truck’s trajectories, as well as specific updates to Sparse Maps to ensure any changes in roads are fed to other trucks.
“The information gleaned through that neural network lets the truck know for instance if its exit is upcoming, or if it needs to change lane for a merge later down the road,” Wendel says.
“There are convolutional elements, but it isn’t a purely convolutional neural network [CNN]; it has a more advanced architecture than that. There are actually quite a few standardised architectures for image-based machine learning and AI that can’t just be called CNNs any more, like transformer networks, attention networks or recurrent networks. So we’ve developed one of our own in-house specifically for traffic lane detection in this instance.
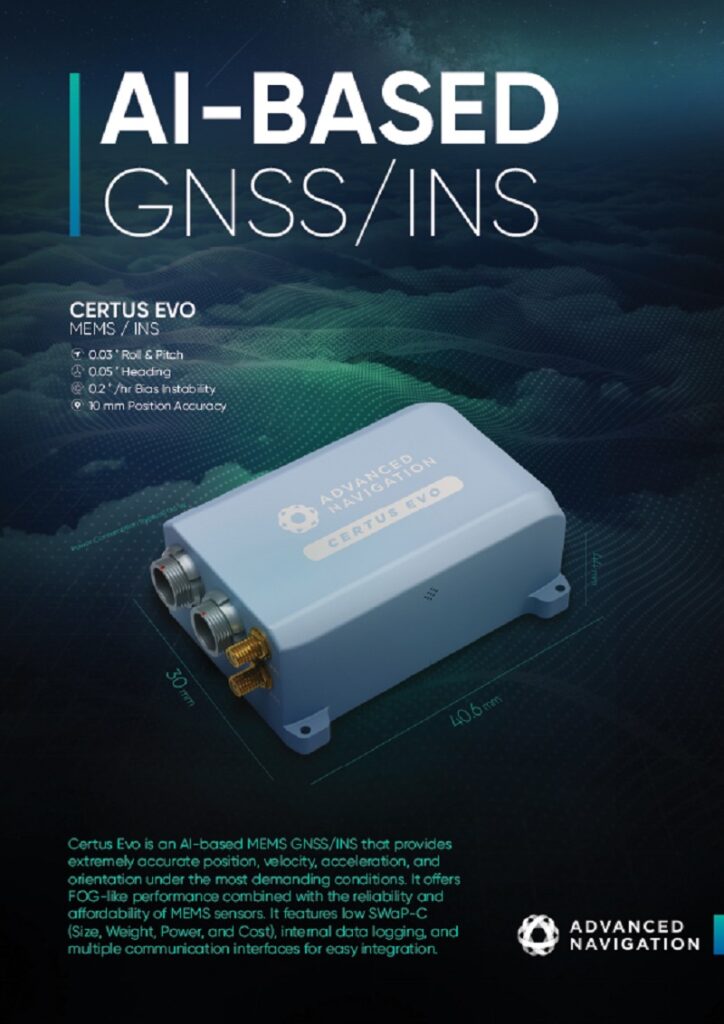
“But we also have other, similar ones for object detection using purely camera imagery, ones where we fuse the camera and Lidar data, ones for radar alone, and radar fused with the other sensors. So again, the redundancy aspect is key to our AI philosophy as much as it is for our sensor hardware. We have numerous modalities producing multiple outputs that we can check against each other to ascertain the presence, location and heading of objects on the road.”
In describing the software layers of Kodiak’s perception architecture, collectively known as Kodiak Vision, Wendel also makes a distinction between early data fusion and late data fusion. In the earlier stages of perception, the data from the Gen4’s cameras, Lidars and radars can be fused in different permutations before being fed into the various neural networks (also called ‘detectors’ in-house).
In late fusion, the outputs from the detectors are fused together to construct a 4D image of the world around the truck. This contains recognisable objects such as cars and any available velocity information to intelligently inform safe avoidance trajectories where needed, as well as obstacles such as barriers that cannot be defined or categorised but nonetheless must be avoided.
Computing and control systems
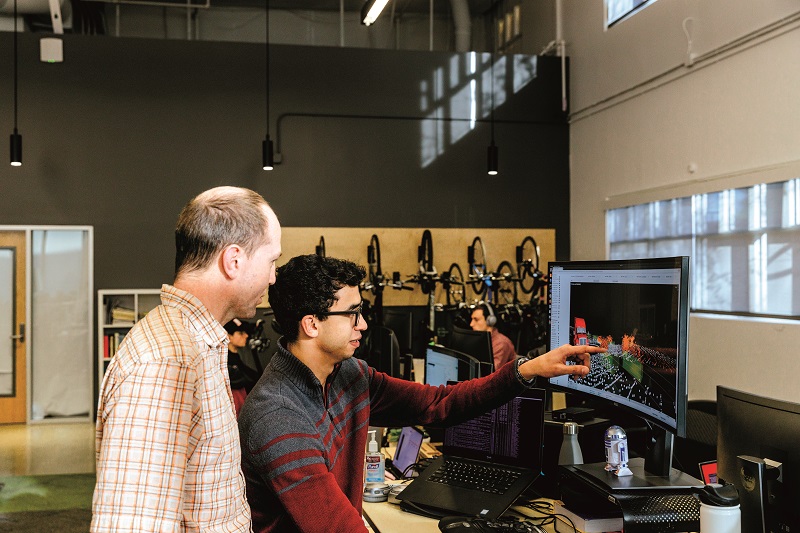
The ability to take in all the perception data and communicate it intelligently to the downstream systems was vital to Kodiak selecting a multi-core and military-grade main computer that would be able to meet its reliability requirements. It is also responsible for some calculations for the detectors using the early fusion data, and the motion planning functions using the late fusion data.
Paramount among the downstream systems is an Nvidia Drive AGX Orin, which serves as the platform for processing the autonomous perception data. While choosing the main computer was a matter of broad functionality, the Orin was chosen because of many its specifications, including its 250 TOPS of computing performance, which is key to processing high-resolution images.
The control functions derived via the motion planning software are communicated to the ACEs that then control the steering, throttle, braking, lights and indicators. Although there are multiple ECUs throughout the vehicle, the ACEs are the most important for driving the truck. Just as the SensorPods remain the same between each truck configuration, the ACEs also provide a universal actuation interface for every truck the Kodiak Driver might be installed on.
“The main computer and Orin talk to each other over Ethernet, while the ACEs use the SAE J-1939 version of CAN bus, meaning we can interface with a wide variety of trucks and other automotive systems and actuators,” Wendel says.
“The ACEs also connect to multiple interfaces for our safety drivers, so they can see at a glance whether they need to take control.”
Both the network architecture and software algorithms were developed using C++, with C used for some of the subsystem embedded computers. And while various frameworks were used to train the neural networks, TensorFlow was generally the tool of choice for developing and maturing Kodiak’s perception and detector AIs.
Remote assistance
To enable remote operation where necessary, the LTE modems used in Sparse Maps also ensure a reliable connection with plentiful bandwidth between each truck and its home base.
“We bond different channels using several SIM cards from a variety of providers, and we spread our modems’ signals across those,” Wendel says. “That gives us more bandwidth, better reception and overall better reliability of our video, telemetry and control links, to the point that we can quickly update all our software and maps over the air.
“Those links are fused together on the back end, so all the packets sent and received through them come together at a central point before being passed to off-board systems.”
As well as being on standby for emergencies, the operator can also remotely set new destinations if requested by a customer or traffic authority for example, and answer ‘questions’ that the truck generates during unusual situations beyond those the AI has recorded in previous instances of certain routes.
“We’ve programmed the Kodiak Driver to output questions in instances that are strange but not always critical,” Wendel explains. “For example, the truck might ask its remote assistant, ‘I’m stuck in a single line of traffic on the highway, which is unusual. Should I go around or wait for the cars in front to move?’ The operations team can then look at the camera feed and make a judgement call.
“These kinds of edge cases are what we expect remote assistance to be most helpful with.”
Multiple layers of security are built into the data links and remote systems, including encryption of comms, automated authentication and authorisation systems, along with a signed boot process as part of the software. Cybersecurity standards such as ISO 21434 provide key guidance to Kodiak’s engineers.
Power
Little of Kodiak’s r&d has gone into the powertrain, aside from selecting components with sufficient interfacing capabilities. The engine for instance comes from Cummins, which through Kodiak’s feedback provided a usable interface to the X15 engine, a 15 litre, turbocharged I6 running on diesel and a four-stroke cycle. This is housed in the front of the Gen4’s cab, ahead of and beneath the windshield.
More notable is the power management system, which is currently integrated on top of a system of batteries totalling 10 kWh to ensure consistent back-up electric power for the onboard electronics. It is a standard item on trucks for powering the cabin’s driver systems and heating.
“The power management is also being continually developed to improve its redundancy, but right now it regulates the output power going to each sensor, actuator and computer to maximise efficiency and electrical safety,” Wendel adds. “And for both the current and next-gen power system, we’re working with Vicor, a leader in power management systems.”
For additional safety during driving, Kodiak works closely with Bridgestone, which integrates its Smart Strain Sensors inside its tyres for gauging key parameters such as tyre pressure, tread wear and wheel axle load.
Future plans
The fifth-generation Kodiak truck does not yet have a specific release date, but it is expected to be unveiled sometime this year. As mentioned, outwardly it will bear a close resemblance to the Gen4.
As the company moves more and more into commercial operations, it will increasingly offer trucks integrating the Kodiak Driver as a transportation service (called Kodiak Express), with payment linked to the mileage requested.
Also, in about 2025 the company anticipates starting to offer Kodiak Driver product kit for companies to integrate it into their own trucks. Kodiak would continue providing remote monitoring, maintenance, network updates and technical support – while still offering autonomous mileage-as-a-service – to enable the companies to focus on their core activities.
Although the Gen5’s development roadmap is well-defined, the company recognises that additional opportunities might arise outside this central focus. Wendel notes for instance that the Kodiak Driver could be applied to other vehicles, meaning autonomous vans, buses, coaches, off-road military vehicles and more could figure in the company’s future.
We want to focus on trucking first though,” he says. “It’s the harshest operating environment for the Driver to be put through, and if we can show repeatedly that it works on a truck, that’ll be the proof that it works on any vehicle. And we don’t want to be stuck in theoretical r&d for different types of self-driving vehicle for years when we already have a viable product.”
- Some key suppliers
- Chassis: PACCAR
- Simulation and testing tools: Applied Intuition
- Test data handling and labelling: Scale AI
- Autonomy hardware platform: Nvidia
- Camera data processors: Ambarella
- Forward Lidars: Luminar
- Side and rear Lidars: Hesai
- Radars: ZF
- Powertrain: Cummins
- Power management: Vicor
- Tyre strain sensors: Bridgestone
UPCOMING EVENTS
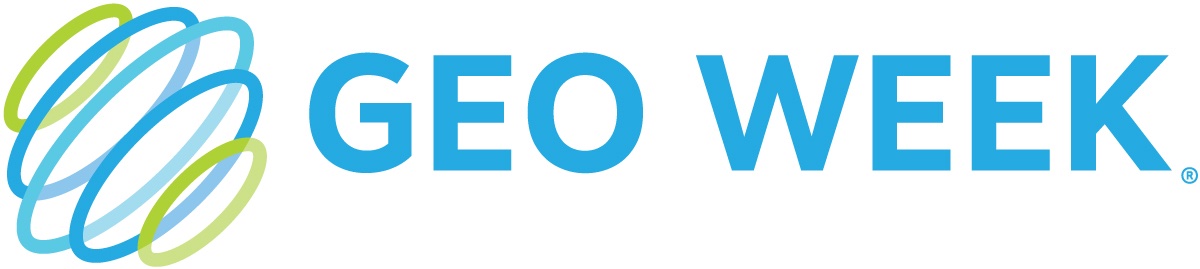
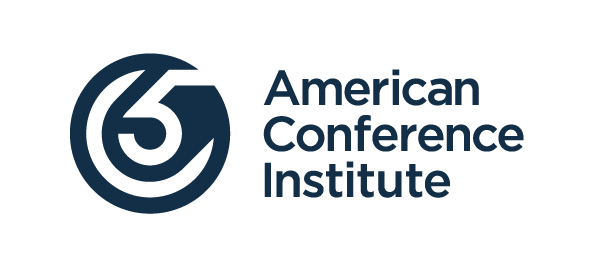
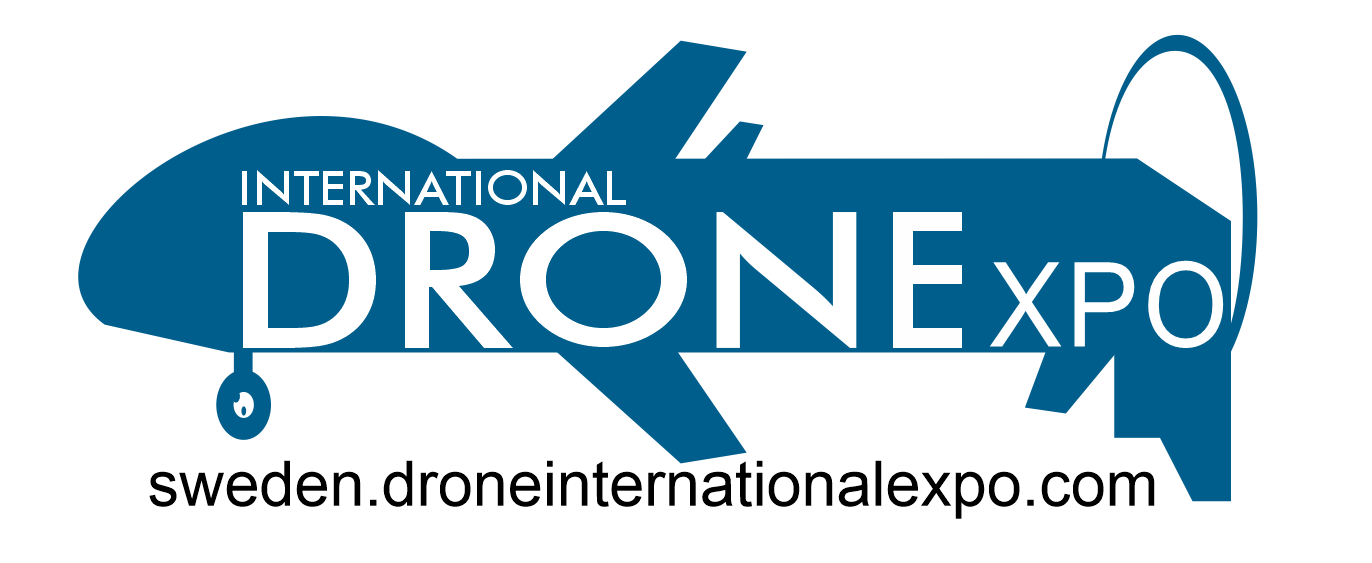
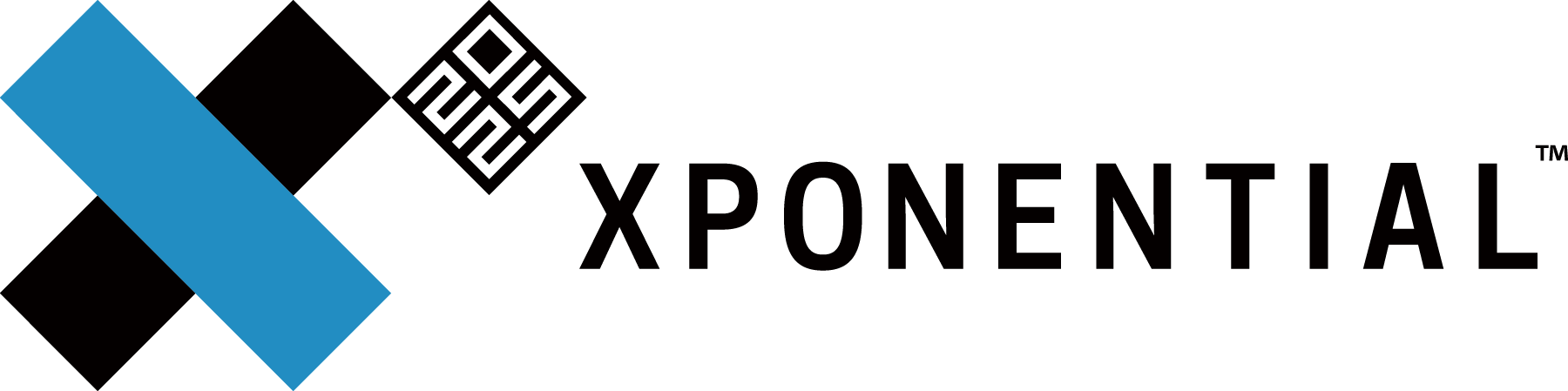
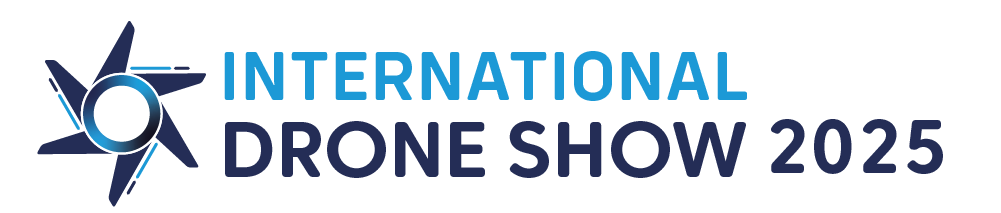