AI system detects UXBs
Seabed safety
Researchers at the University of Bath in the UK have developed a machine learning (ML) technique to detect unexploded bombs at the bottom of the sea (writes Nick Flaherty).
The system uses large, unlabelled survey datasets to aid automatic classification from synthetic aperture sonar (SAS) data. The researchers simulated this data to train an AI framework that would be used by vehicles such as the REMUS 620 (see page 16) to autonomously detect munitions that have been discarded. Autonomous underwater vehicles equipped with SAS can survey large areas at centimetre resolution, but that generates a lot of data that needs an automated approach to detecting and classifying unexploded munitions.
The ML model encodes a representation of SAS images from which new SAS views can be generated. This requires the model to learn the physics and content of the images without the need for human labels in self-supervised learning.
A more accurate graphics technology called ray tracing was used to generate realistic images, and noise was then added to match the statistics of real SAS images. These were 18,000 images of the Skagerrak UXO dumpsite taken using the HISAS 1030 sonar system.
The pre-trained model can then be fine-tuned to perform classification on a small amount of labelled examples with 700 training images and 2500 test images.
A 250 kg, 1.8 m-long bomb was used to demonstrate the accuracy of the system, which worked better than a traditional self-supervised approach and systems with no pre-training.
UPCOMING EVENTS
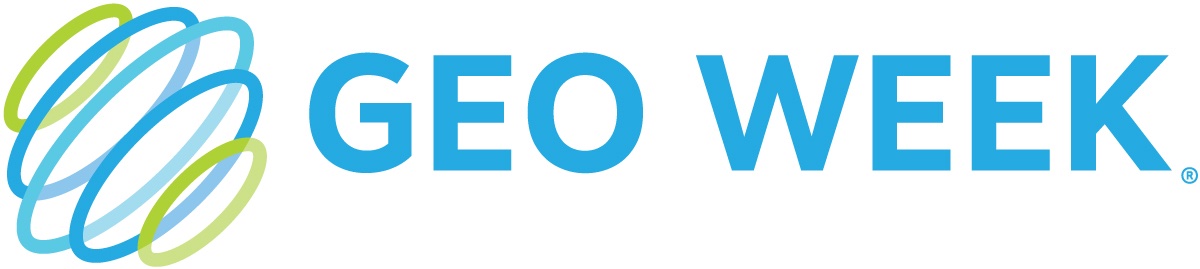
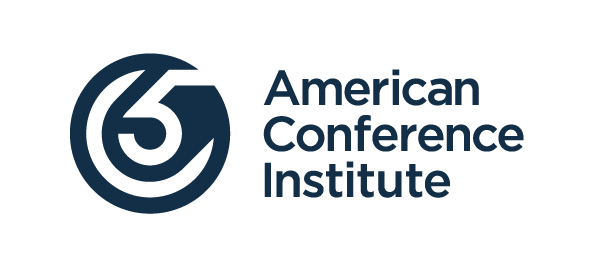
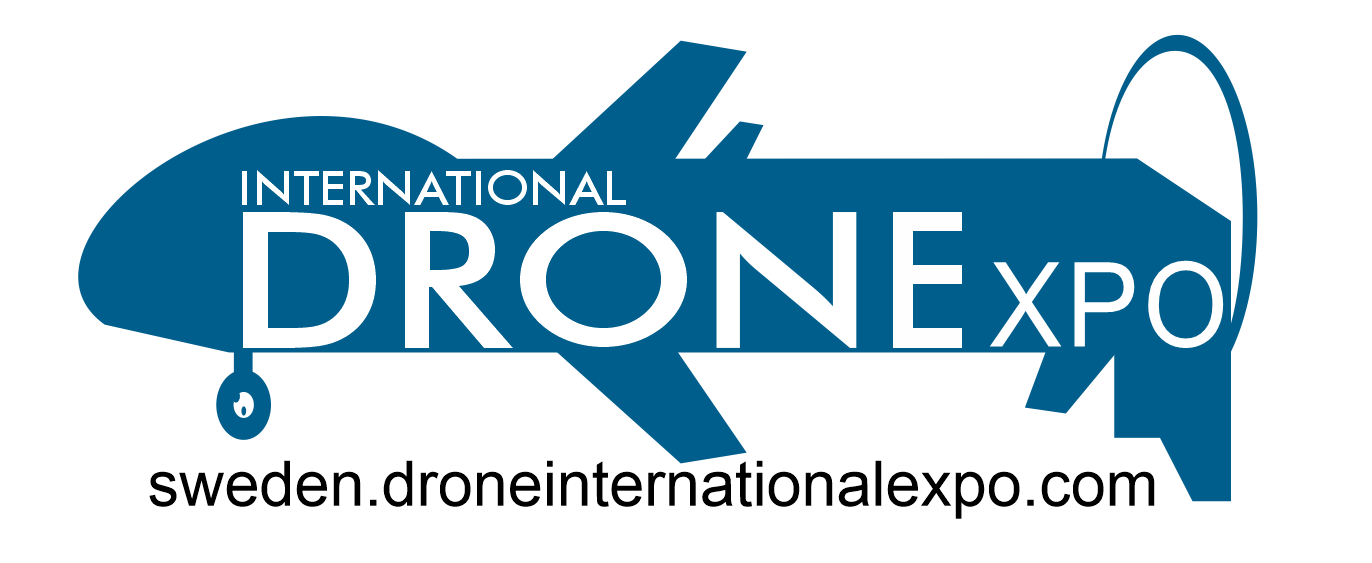
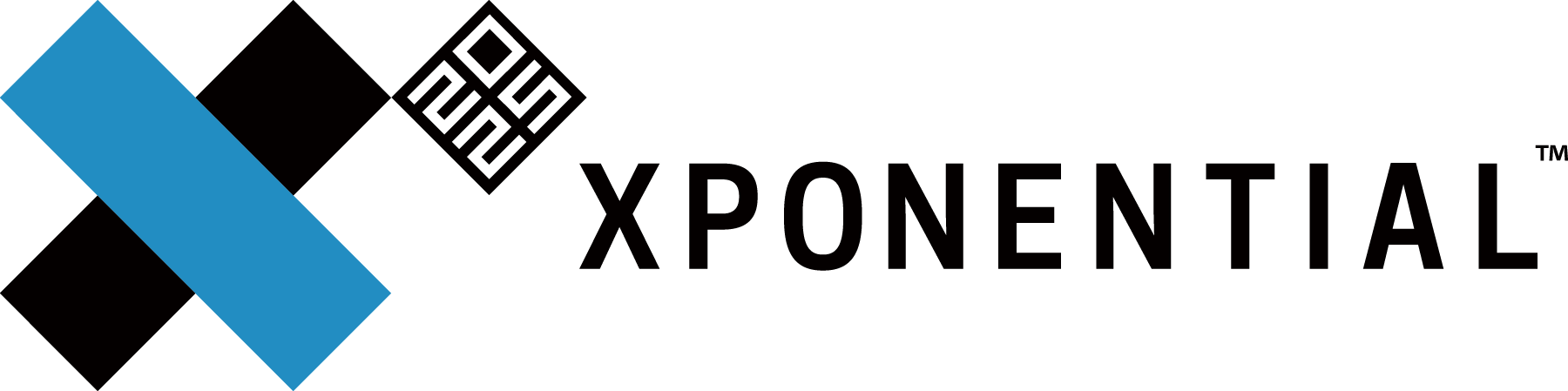
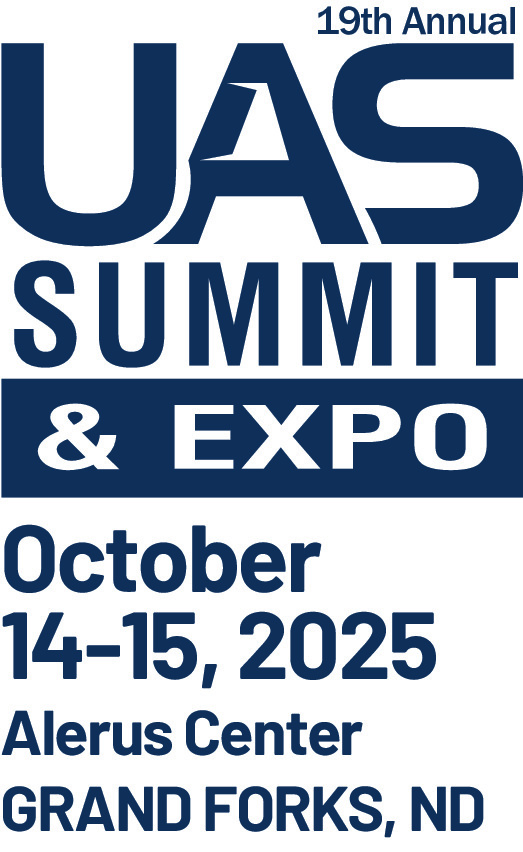

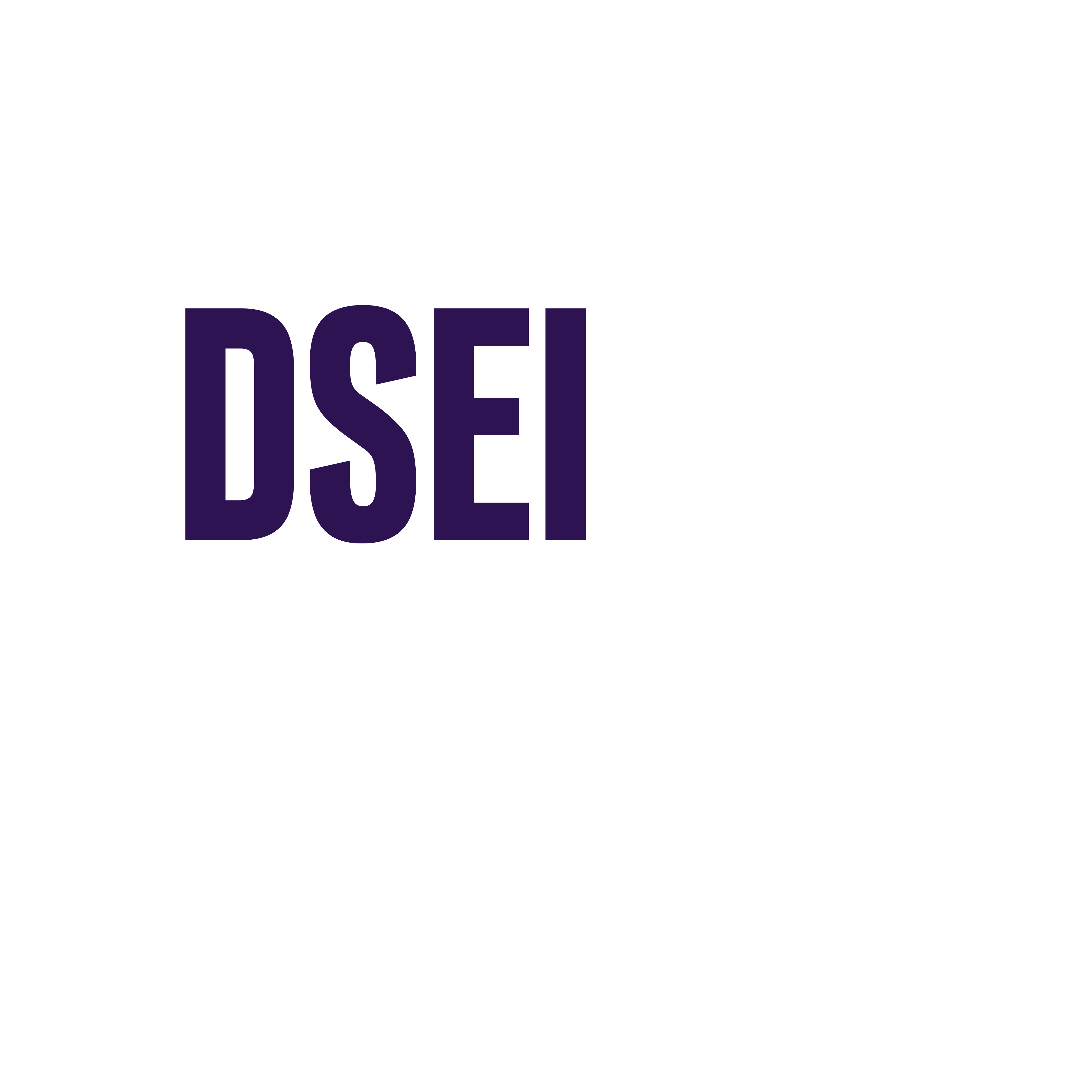
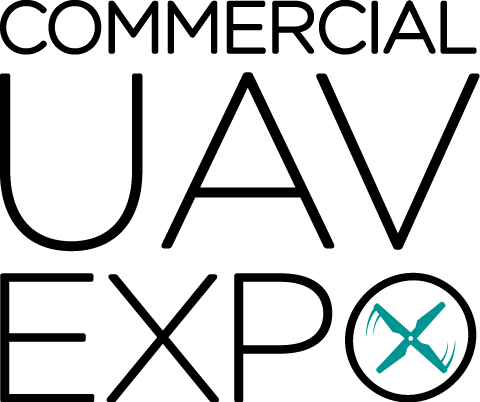
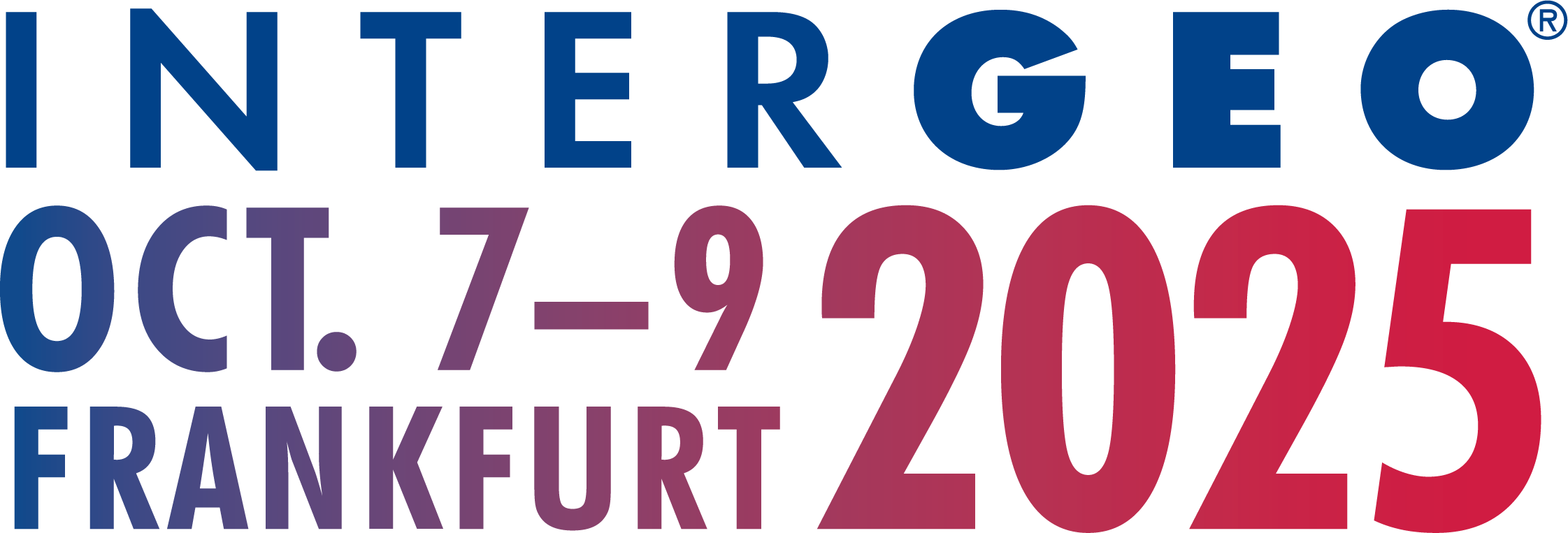
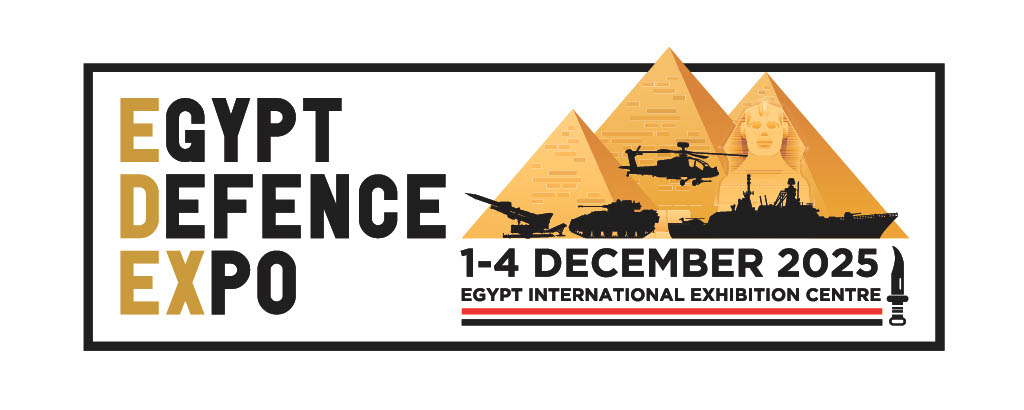
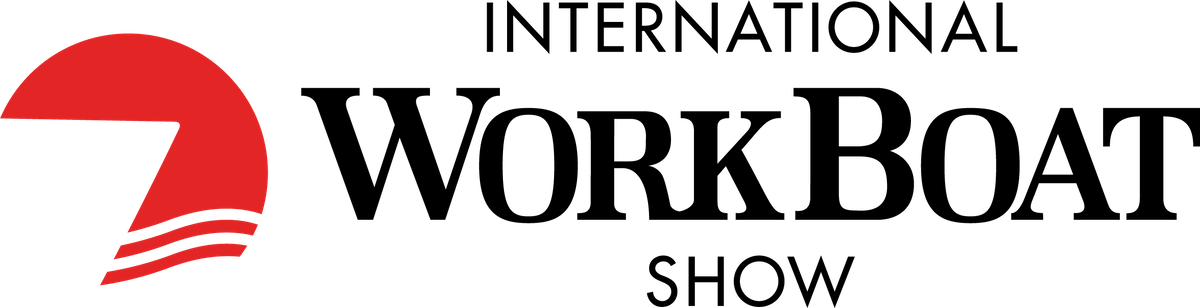