AI speeds up 3D surveying
Researchers in Switzerland have used machine learning to develop a way to make 3D mapping more cost-effective (writes Nick Flaherty).
“Switzerland is currently mapping its entire landscape using airborne laser scanners – for the first time since 2000– but the process will take 4 or 5 years, since the scanners have to fly at an altitude below 1 km if they are to collect data with sufficient detail and accuracy,” said Jan Skaloud, a senior scientist at the Geodetic Engineering Laboratory in the EPFL’s School of Architecture, Civil and Environmental Engineering, which developed the algorithms.
“With our method, surveyors can send laser scanners as high as 5 km and still maintain accuracy. Our lasers are more sensitive and can beam light over a much wider area, making the process five times faster,” he said.
The accuracy of Lidar scanners can be lost when mounted on UAVs o rother moving vehicles, especially in areas with numerous obstacles such as cities, infrastructure sites and places where GNSS satellite navigation signals are interrupted. This results in gaps and misalignments in the data used to generate the 3D point cloud, and can lead to ‘double vision’ of scanned objects. These errors must be corrected manually before a map can be used.
“For now, there’s no way to generate perfectly aligned 3D maps without a manual data correction step,” said Davide Cucci, senior research associate at the Research Center for Statistics of the Geneva School of Economics and Management of the University of Geneva, who also worked on the technology.
“A lot of semi-automatic methods are being explored to overcome this problem, but ours has the advantage of resolving the issue directly at the scanner level, where measurements are taken, eliminating the need to subsequently make corrections. It’s also fully software-driven, meaning it can be implemented quickly and seamlessly by end-users.”
The technique uses machine learning to detect when a given object has been scanned several times from different angles. It involves selecting correspondences and inserting them into a dynamic network to correct gaps and misalignments in the Lidar point cloud. That improves the point cloud registration accuracy by a factor of five in normal use and by a factor of 10 in simulations with a GNSS outage.
UPCOMING EVENTS
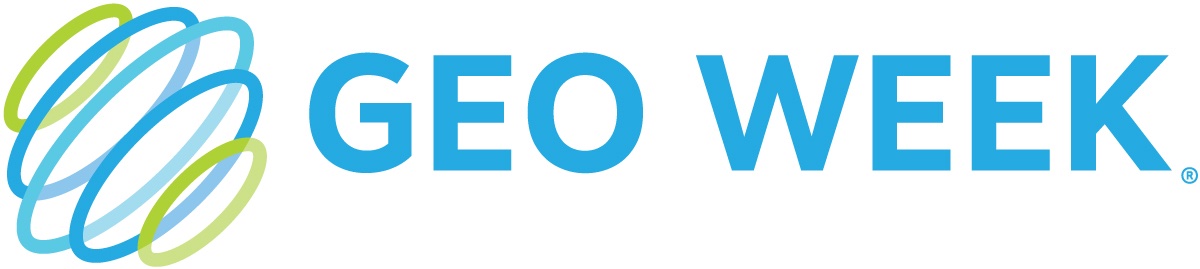
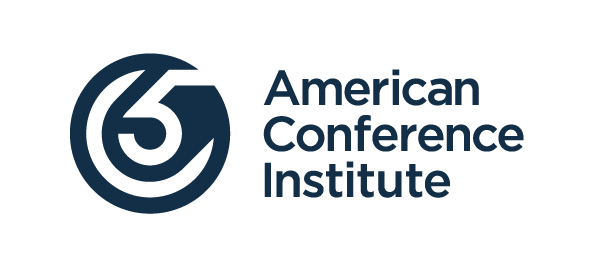
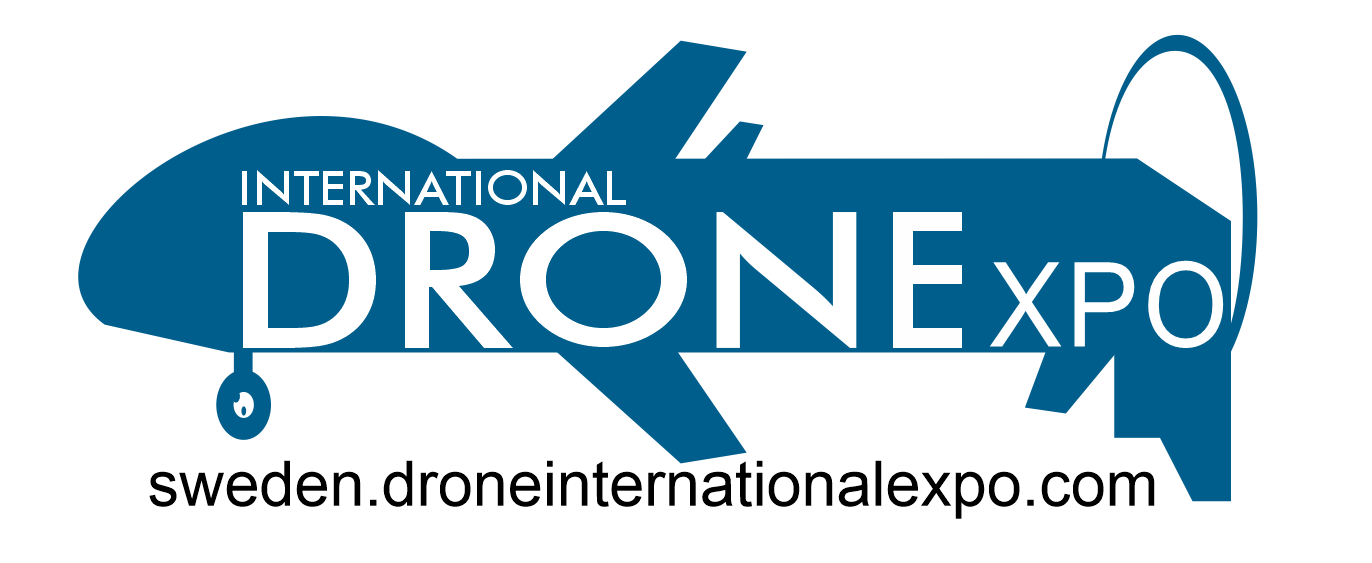
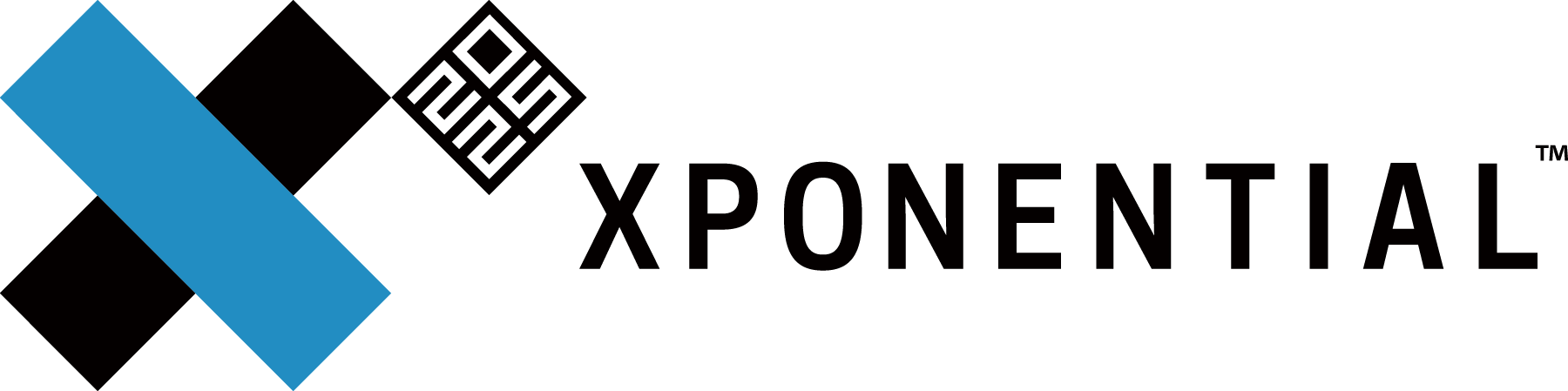
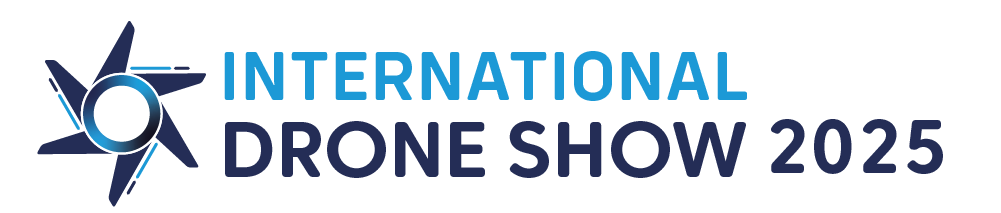